Reality Bites: Assessing the Realism of Driving Scenarios with Large Language Models
arxiv(2024)
摘要
Large Language Models (LLMs) are demonstrating outstanding potential for
tasks such as text generation, summarization, and classification. Given that
such models are trained on a humongous amount of online knowledge, we
hypothesize that LLMs can assess whether driving scenarios generated by
autonomous driving testing techniques are realistic, i.e., being aligned with
real-world driving conditions. To test this hypothesis, we conducted an
empirical evaluation to assess whether LLMs are effective and robust in
performing the task. This reality check is an important step towards devising
LLM-based autonomous driving testing techniques. For our empirical evaluation,
we selected 64 realistic scenarios from –an open driving scenario
dataset. Next, by introducing minor changes to them, we created 512 additional
realistic scenarios, to form an overall dataset of 576 scenarios. With this
dataset, we evaluated three LLMs (, , and ) to assess their
robustness in assessing the realism of driving scenarios. Our results show
that: (1) Overall, achieved the highest robustness compared to and
, consistently throughout almost all scenarios, roads, and weather
conditions; (2) performed the worst consistently; (3) achieved
good results under certain conditions; and (4) roads and weather conditions do
influence the robustness of the LLMs.
更多查看译文
AI 理解论文
溯源树
样例
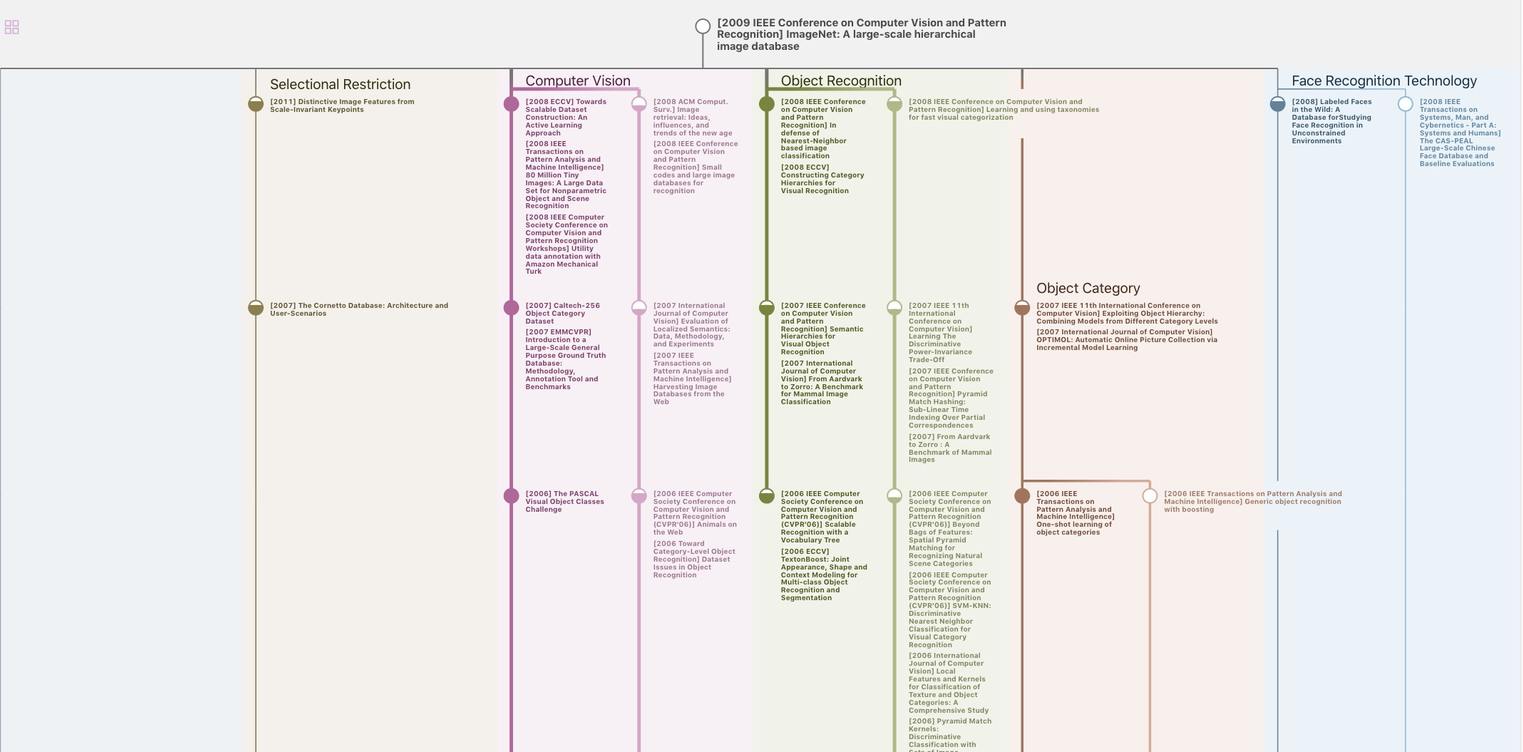
生成溯源树,研究论文发展脉络
Chat Paper
正在生成论文摘要