A Constrained Deep Reinforcement Learning Optimization for Reliable Network Slicing in a Blockchain-Secured Low-Latency Wireless Network
arxiv(2024)
摘要
Network slicing (NS) is a promising technology that supports diverse
requirements for next-generation low-latency wireless communication networks.
However, the tampering attack is a rising issue of jeopardizing NS
service-provisioning. To resist tampering attacks in NS networks, we propose a
novel optimization framework for reliable NS resource allocation in a
blockchain-secured low-latency wireless network, where trusted base stations
(BSs) with high reputations are selected for blockchain management and NS
service-provisioning. For such a blockchain-secured network, we consider that
the latency is measured by the summation of blockchain management and NS
service-provisioning, whilst the NS reliability is evaluated by the BS
denial-of-service (DoS) probability. To satisfy the requirements of both the
latency and reliability, we formulate a constrained computing resource
allocation optimization problem to minimize the total processing latency
subject to the BS DoS probability. To efficiently solve the optimization, we
design a constrained deep reinforcement learning (DRL) algorithm, which
satisfies both latency and DoS probability requirements by introducing an
additional critic neural network. The proposed constrained DRL further solves
the issue of high input dimension by incorporating feature engineering
technology. Simulation results validate the effectiveness of our approach in
achieving reliable and low-latency NS service-provisioning in the considered
blockchain-secured wireless network.
更多查看译文
AI 理解论文
溯源树
样例
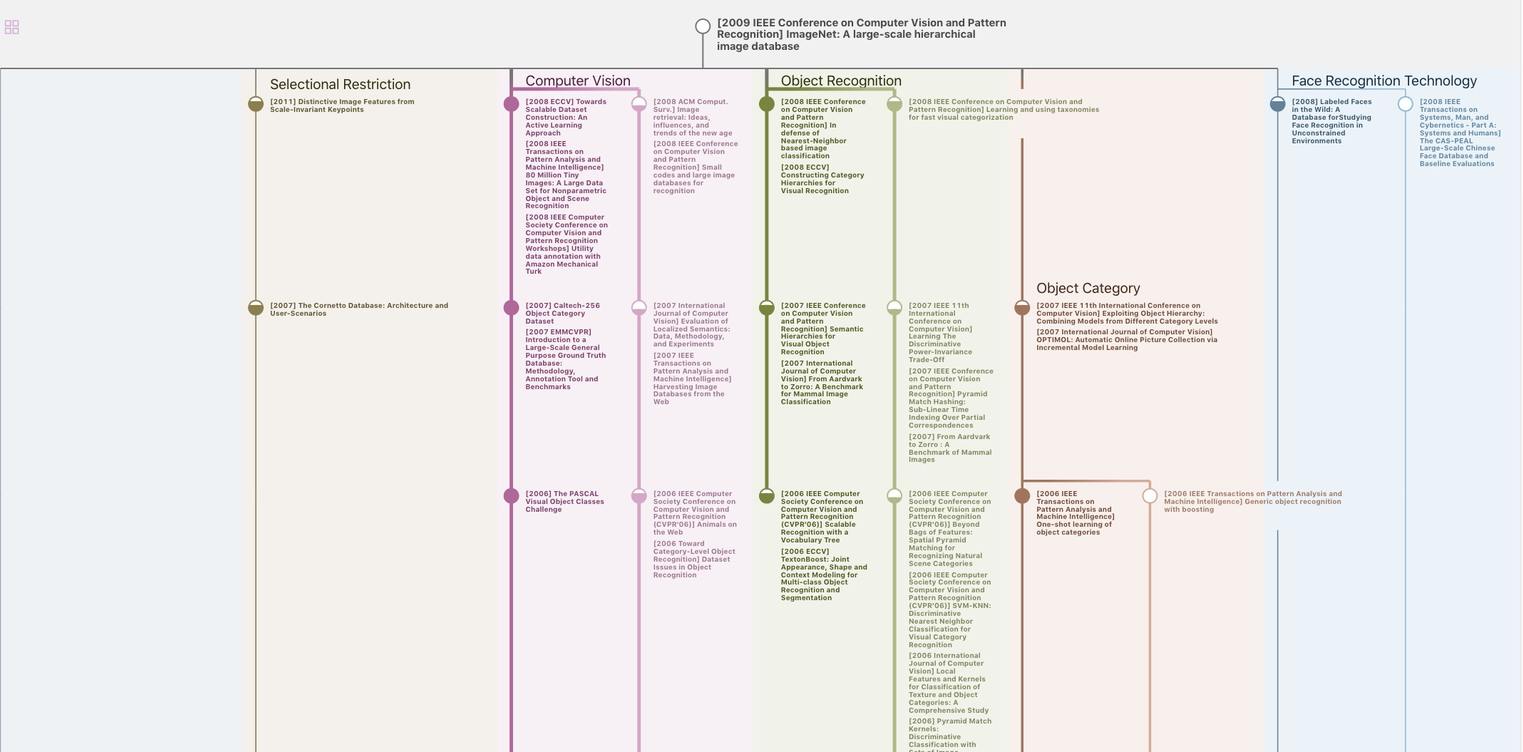
生成溯源树,研究论文发展脉络
Chat Paper
正在生成论文摘要