Fast dominant feature selection with compensation for efficient image steganalysis
Signal Processing(2024)
摘要
Image steganalysis has witnessed significant development but still encounters challenges in detection speed and accuracy. Based on this consideration, this paper proposes a simple yet efficient dominant feature selection method. First, a separability measurement is designed utilizing the principles of “intraclass aggregation and interclass dispersion” and “maximum interclass disparity”, by which the contribution of each feature is better evaluated. Second, a separability measurement is presented considering the “holistic interclass disparity”, and thus dominant features are directly determined. Moreover, a compensation strategy is proposed to reduce the possibility of missing dominant features, thereby further enhancing the accuracy of the selected features. The effectiveness of the proposed method is empirically verified. Extensive experiments are conducted on the BOSSbase 1.01 and ALASKA2 datasets. The results show that, compared to some state-of-the-art works, the proposed method achieves better performance in terms of computational cost, feature dimension, and detection accuracy. In particular, the computational cost of the proposed method is extremely low.
更多查看译文
关键词
Steganalysis,Dominant feature selection,Separability measurement,Feature compensation,Fast selection
AI 理解论文
溯源树
样例
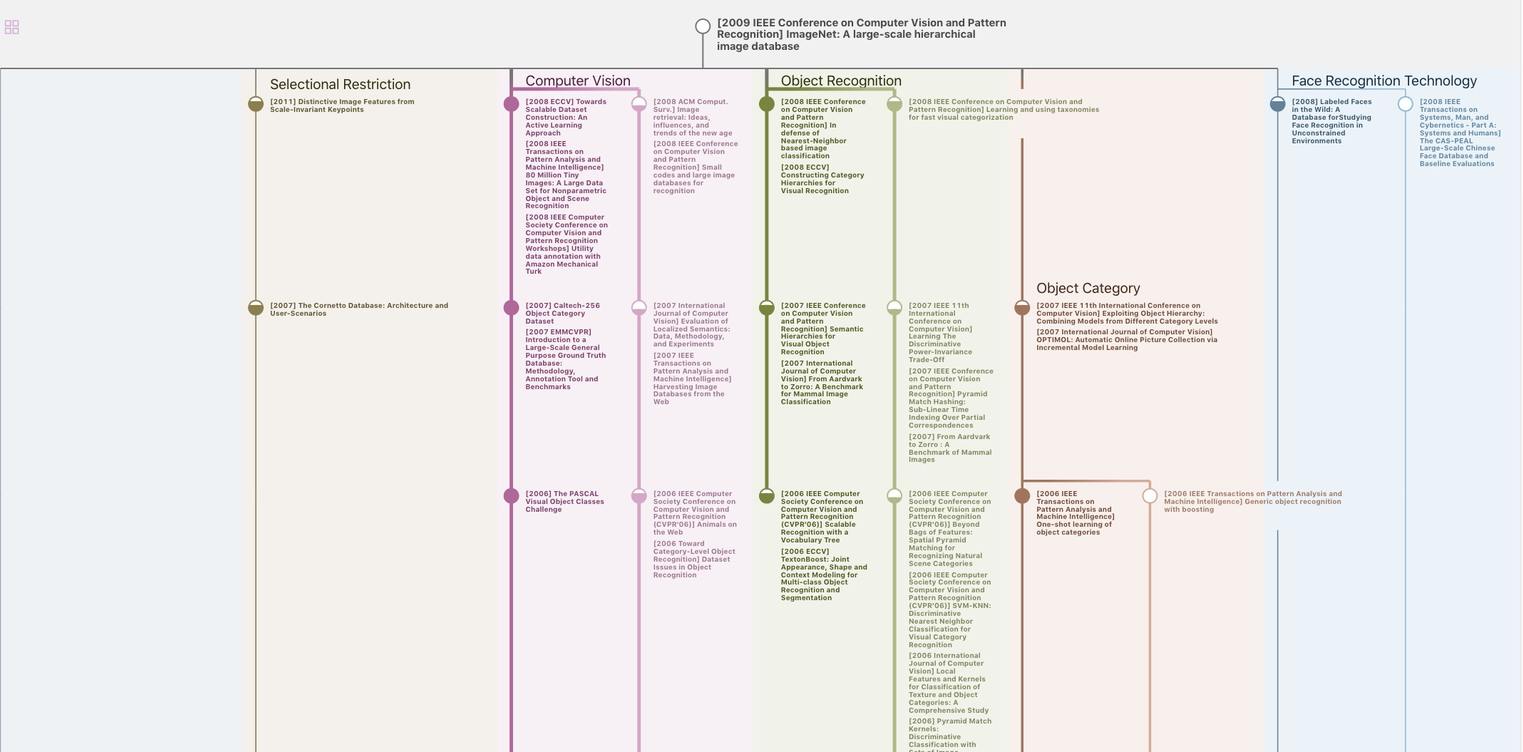
生成溯源树,研究论文发展脉络
Chat Paper
正在生成论文摘要