Efficient and self-recoverable privacy-preserving k-NN classification system with robustness to network delay
Journal of Systems Architecture(2024)
摘要
Online classification services based on machine learning have been widely used in fields such as healthcare and finance. To enhance the data privacy and avoid server collusion, companies usually deploy each server on different cloud service providers in distant regions separately, which implies high network delay between servers. However, many existing schemes inevitably involve a large number of communication rounds, resulting in inefficient online performance. To mitigate the negative effect of network delay, we propose an efficient and delay-robust approach for privacy-preserving k-NN classification on two non-interactive servers. Our method utilizes the state-of-the-art homomorphic secret sharing (HSS) technique and only introduces constant communication rounds. We also design an efficient self-recovery mechanism by enabling redundant cloud servers to securely back up the encrypted dataset, which is efficient even under high network delay. As the result of experiments we conducted in various network environment, our scheme is more online-efficient and is more robust to high network delay compared with related works.
更多查看译文
关键词
k-nearest neighbor,Privacy-preserving cloud computing,Homomorphic secret sharing,Multi-party computation
AI 理解论文
溯源树
样例
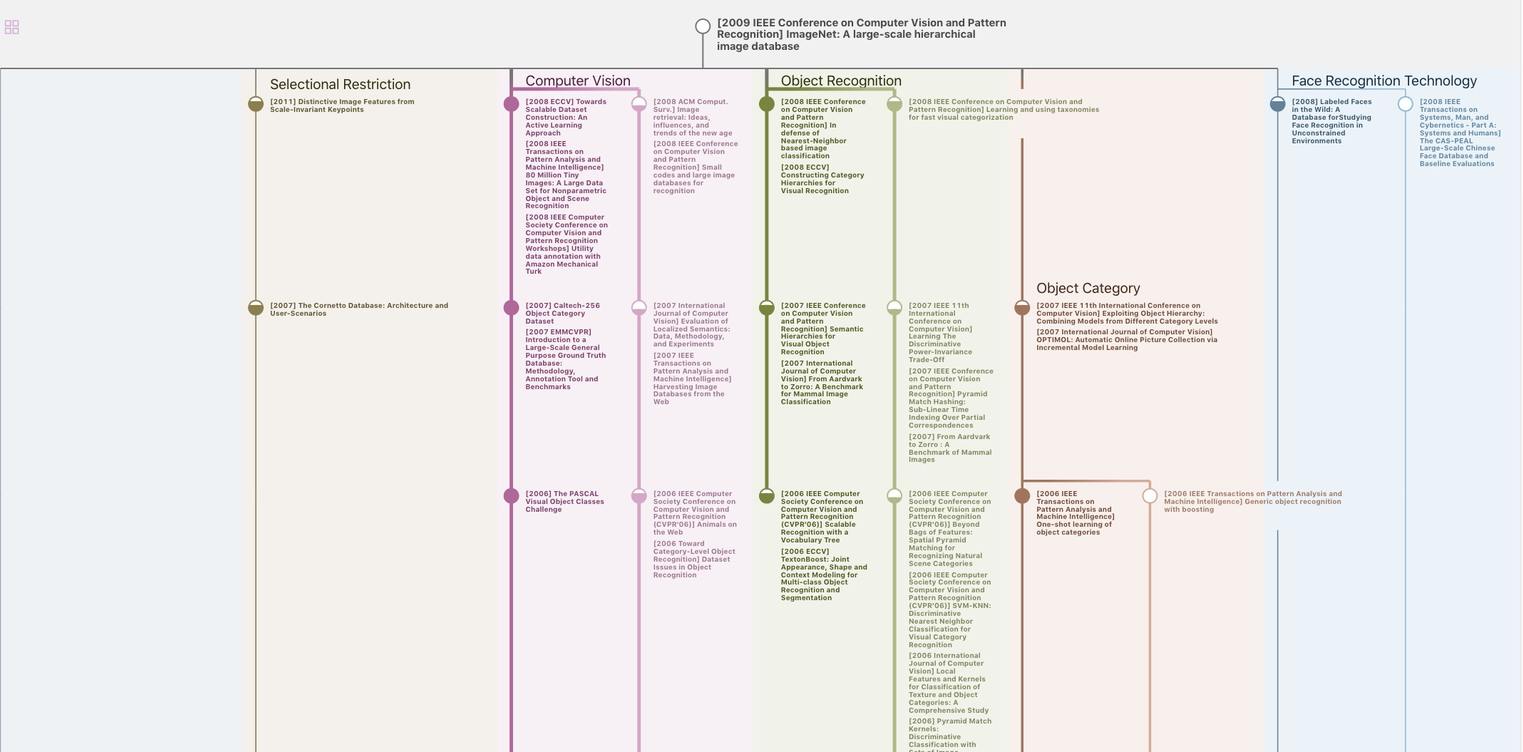
生成溯源树,研究论文发展脉络
Chat Paper
正在生成论文摘要