A developed convolutional neural network model for accurately and stably predicting effective thermal conductivity of gradient porous ceramic materials
INTERNATIONAL JOURNAL OF HEAT AND MASS TRANSFER(2024)
摘要
Accurate and stable prediction of the effective thermal conductivity (ETC) of porous ceramic materials is of great significance for their application in areas such as optimizing the design of thermal barrier coatings and improving energy conversion efficiency. Porous ceramic materials exhibit complex porous structures with gradient porosity distributions that pose a great challenge for effective medium theory (EMT) and conventional convolutional neural networks (CNN) in attempting to accurately and stably predict the ETC of porous media. In this study, a CNN model that integrates self-attention and a multiscale feature-fusion mechanism is proposed to predict the ETC of porous media with greater accuracy and stability. The integration of the self-attention and multiscale feature-fusion mechanisms enhances the CNN's ability to learn long-range dependencies and preserve detailed information. The optimization of the CNN's accuracy and stability is visually illustrated using gradient-weighted class activation mapping (Grad-CAM) for ETC. Additionally, by employing the proposed gradient quartet structure generation set (QSGS), a gradient porous ceramic media dataset comprising 10 000 images was built to train the CNN model. Finally, the prediction results and relative error distribution of the ETC were compared across different models. Our model demonstrated improvements in statistical metrics, including a 33.7 % decrease in mean error, 25.2 % decrease in median error, and 59.6 % decrease in maximum error. The decreases in these metrics and Grad-CAM for the ETC strongly demonstrates that the model proposed in this work greatly improved the accuracy and stability of predicting the ETC of ceramic materials with gradient porosity distributions.
更多查看译文
关键词
Gradient porous ceramic,Effective thermal conductivity,CNN,Self-attention mechanism,Grad-CAM
AI 理解论文
溯源树
样例
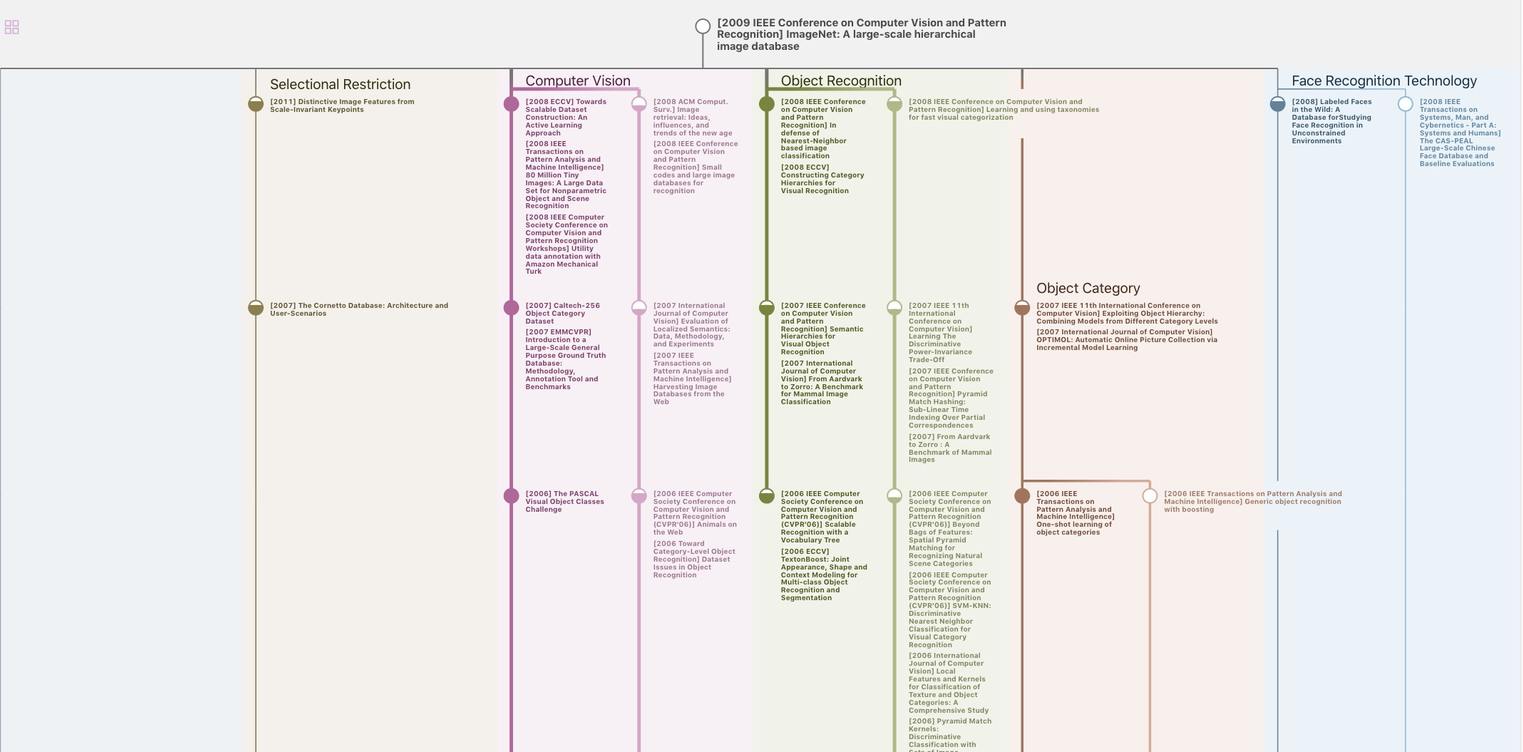
生成溯源树,研究论文发展脉络
Chat Paper
正在生成论文摘要