754 Prediction of 30-day All-Cause Readmission of Neurosurgery Patients Using Large Language Models
Neurosurgery(2024)
摘要
INTRODUCTION: Existing clinical prediction algorithms mostly leverage small cohorts of structured data (e.g., medical imaging or laboratory data). However, large language models have demonstrated the ability to utilize unstructured data to outperform other machine learning approaches given sufficient data. Training large language models on unstructured clinical notes offers a possible alternative to structured data algorithm development in clinical tasks. METHODS: An unlabeled dataset of over seven million unstructured clinical notes (e.g., radiology reports and patient histories) was collected from four hospitals within the NYU Langone Health (NYULH) system and utilized to pre-train a bidirectional encoder representation with transformer (BERT) model. This model was further fine-tuned using a labeled dataset of discharge summaries to predict 30-day all-cause readmission. The resulting model, termed NYUTron, was assessed using a held-out retrospective cohort of patients from June to December 2021. RESULTS: Over the period of the retrospective study, there were a total 1,072 neurosurgery patients. The BERT model achieved an area under the receiver operating curve of 0.7883, a recall of 95.1% at a precision of 27.3%, and an accuracy of 82.1%. CONCLUSIONS: This study demonstrates how large language models and unstructured clinical notes can be used to provide information to physicians on clinical tasks with a flexible framework that is amenable to modification for other clinical tasks.
更多查看译文
AI 理解论文
溯源树
样例
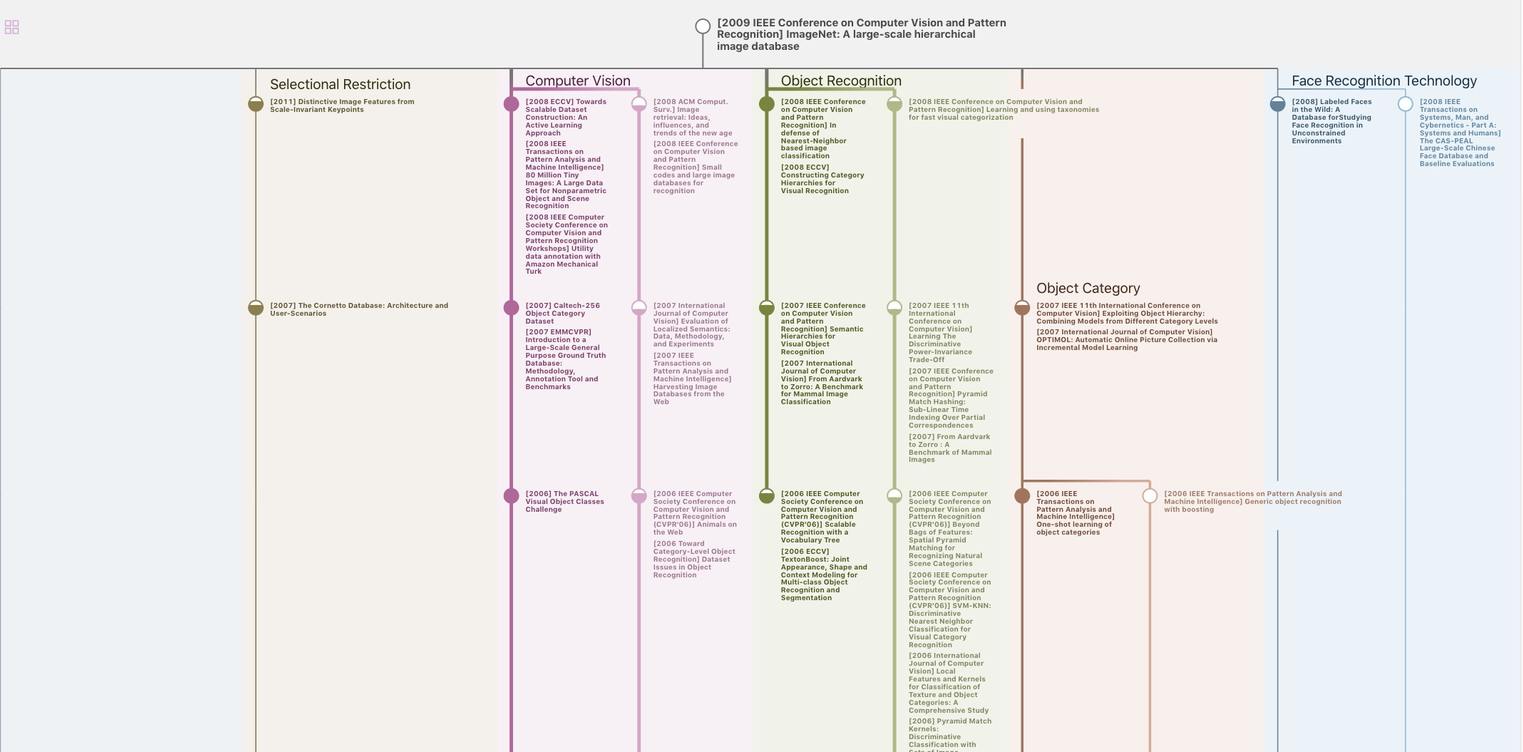
生成溯源树,研究论文发展脉络
Chat Paper
正在生成论文摘要