Optimal Space-Borne ISAR Imaging of Space Objects with Co-Maximization of Doppler Spread and Spacecraft Component Area
REMOTE SENSING(2024)
摘要
The Inverse Synthetic Aperture Radar (ISAR) has been proven to be an effective tool for space target sensing due to its capability of performing high-resolution imaging. Since the component information of the spacecraft is key to the identification of the target and diagnosis of its status, ISAR images with a clear and complete representation of the typical components are much desired. This requires a selection of the imaging time, during which a certain spacecraft component has a good projection on the ISAR image plane with the shape feature well conserved and a high resolution. In addition, a fully automated implementation with a high computational efficiency is also highly preferred for on-orbit operations so as to improve the intelligence level of the space-borne system. We propose a bicriterion-based automated optimal imaging time-selection method for the space-borne ISAR, which is seeking the slow time section of the data that result in the best image. A good image means a high azimuth resolution and the best presentation of the solar panels. One criterion is the Maximum Doppler Spread (MDS), which indicates the maximum Effective Rotational Velocity (ERV) leading to high image resolution, but it is influenced by the satellite attitude. of the spacecraft. The other is the Maximum Component Area (MCA), which is defined to indicate the completeness of the component considered. The radar echoes are processed sequentially by way of a sliding window. The interval with the co-maximization of the DS and CA is selected, and fine processing is performed further to obtain the best images. The results of the simulation experiments show that the proposed method can achieve spacecraft images with the solar panels presented the best. The computational complexity is low.
更多查看译文
关键词
inverse synthetic aperture radar (ISAR) imaging,optimal time selection,space targets,component information
AI 理解论文
溯源树
样例
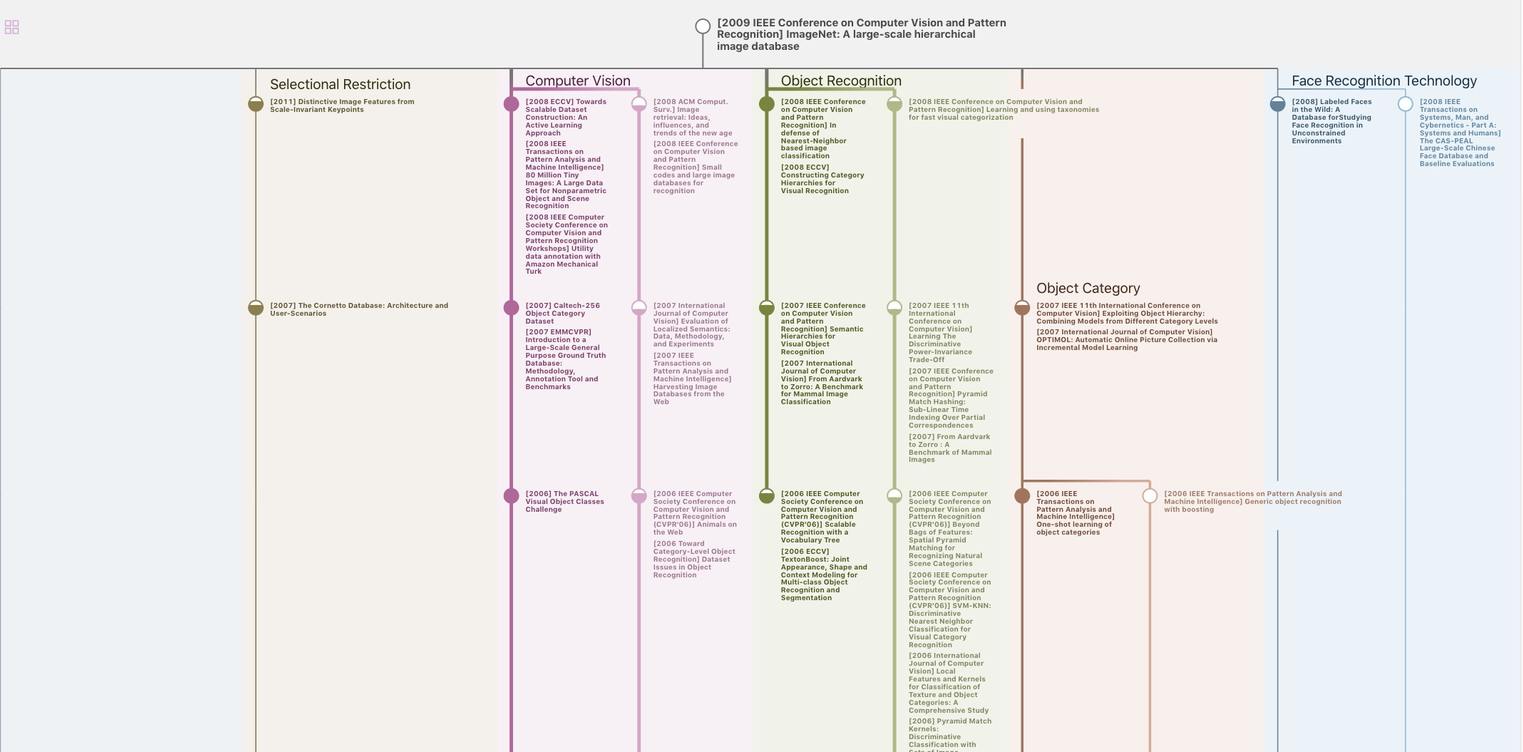
生成溯源树,研究论文发展脉络
Chat Paper
正在生成论文摘要