ECMS-NET:A multi-task model for early endometrial cancer MRI sequences classification and segmentation of key tumor structures
BIOMEDICAL SIGNAL PROCESSING AND CONTROL(2024)
摘要
Endometrial cancer, one of the three major malignant tumors of the female genitalia, has seen an increase in incidence in recent years. The 5 -year survival rate for early endometrial cancer is approximately 80% to 90%. In clinical practice, the accurate segmentation of endometrial cancer tumors is crucial for assessing the extent of hyperplasia and guiding treatment decisions. Developing a deep learning (DL) -based tumor segmentation method for Magnetic Resonance Imaging (MRI) sequences in cases of Endometrial Cancer (EC) can significantly improve the accuracy and efficiency of this process. We proposed an multi -task framework: ECMS-Net. We established a classification model based on the Transformer algorithm to discern images containing tumors within the sequence and U2 -Net segmentation model was trained to perform automated and precise segmentation of early endometrial cancer tumor regions. Our results show that we have firstly proposed a sequence classification method and achieved an accuracy of 0.985 and this is the best result of the study so far, followed by a tumor segmentation maxF1 value of 0.970, which highlights the excellent performance of our proposed method. The entire experimental process aligns closely with the demands of the clinical imaging department, promising to enhance the efficiency of medical professionals, alleviate workload stress, and better cater to the requirements of the medical field.
更多查看译文
关键词
Endometrial cancer,Magnetic resonance imaging,Sequences classification,Tumor segmentation,Deep learning.
AI 理解论文
溯源树
样例
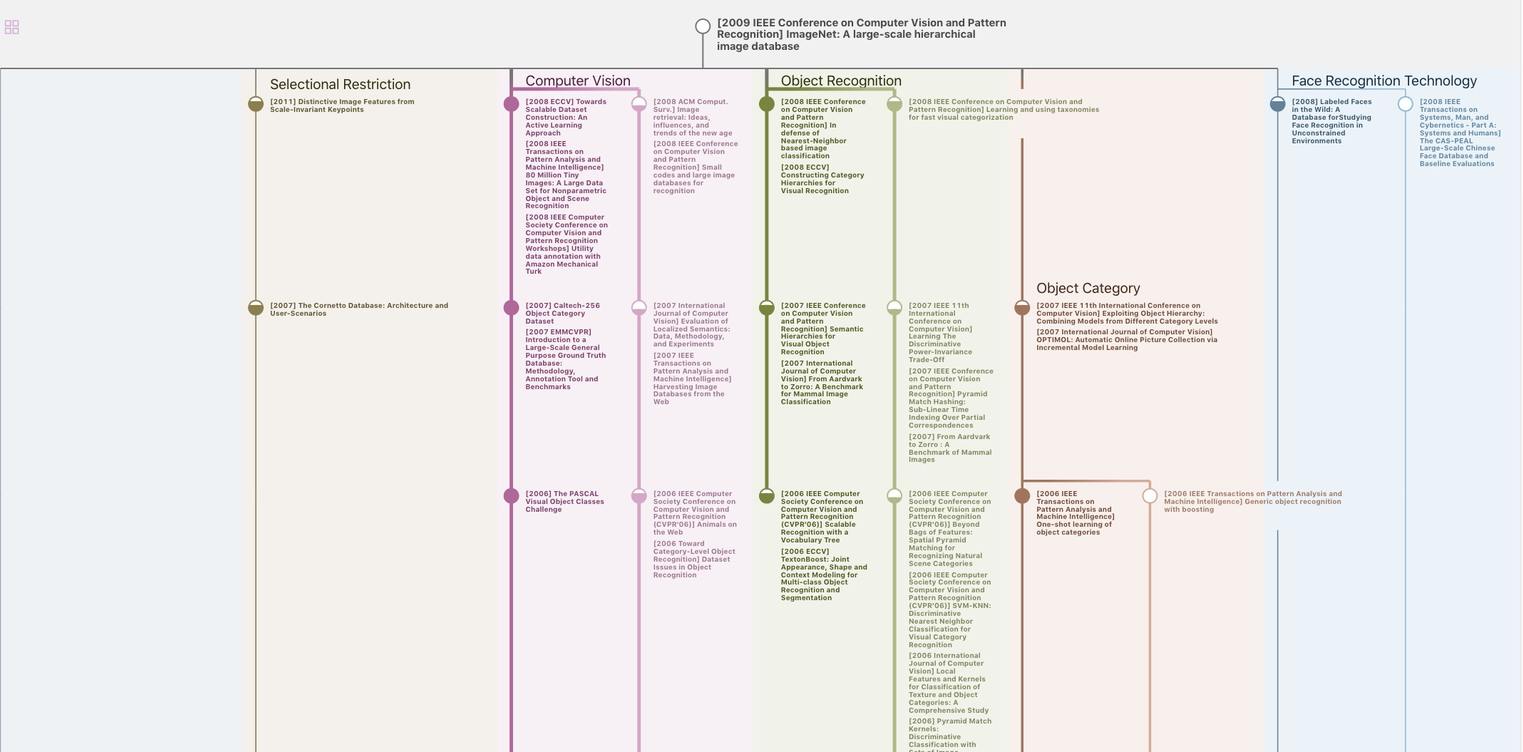
生成溯源树,研究论文发展脉络
Chat Paper
正在生成论文摘要