Enhancing Gamma-Ray Burst Detection: Evaluation of Neural Network Background Estimator and Explainable AI Insights
Galaxies(2024)
摘要
The detection of Gamma-Ray Bursts (GRBs) using spaceborne X/gamma-ray photon detectors depends on a reliable background count rate estimate. This study focuses on evaluating a data-driven background estimator based on a neural network designed to adapt to various X/gamma-ray space telescopes. Three trials were conducted to assess the effectiveness and limitations of the proposed estimator. Firstly, quantile regression was employed to obtain an estimation with a confidence range prediction. Secondly, we assessed the performance of the neural network, emphasizing that a dataset of four months is sufficient for training. We tested its adaptability across various temporal contexts, identified its limitations and recommended re-training for each specific period. Thirdly, utilizing Explainable Artificial Intelligence (XAI) techniques, we delved into the neural network output, determining distinctions between a network trained during solar maxima and one trained during solar minima. This entails conducting a thorough analysis of the neural network behavior under varying solar conditions.
更多查看译文
关键词
GRB,neural network,XAI
AI 理解论文
溯源树
样例
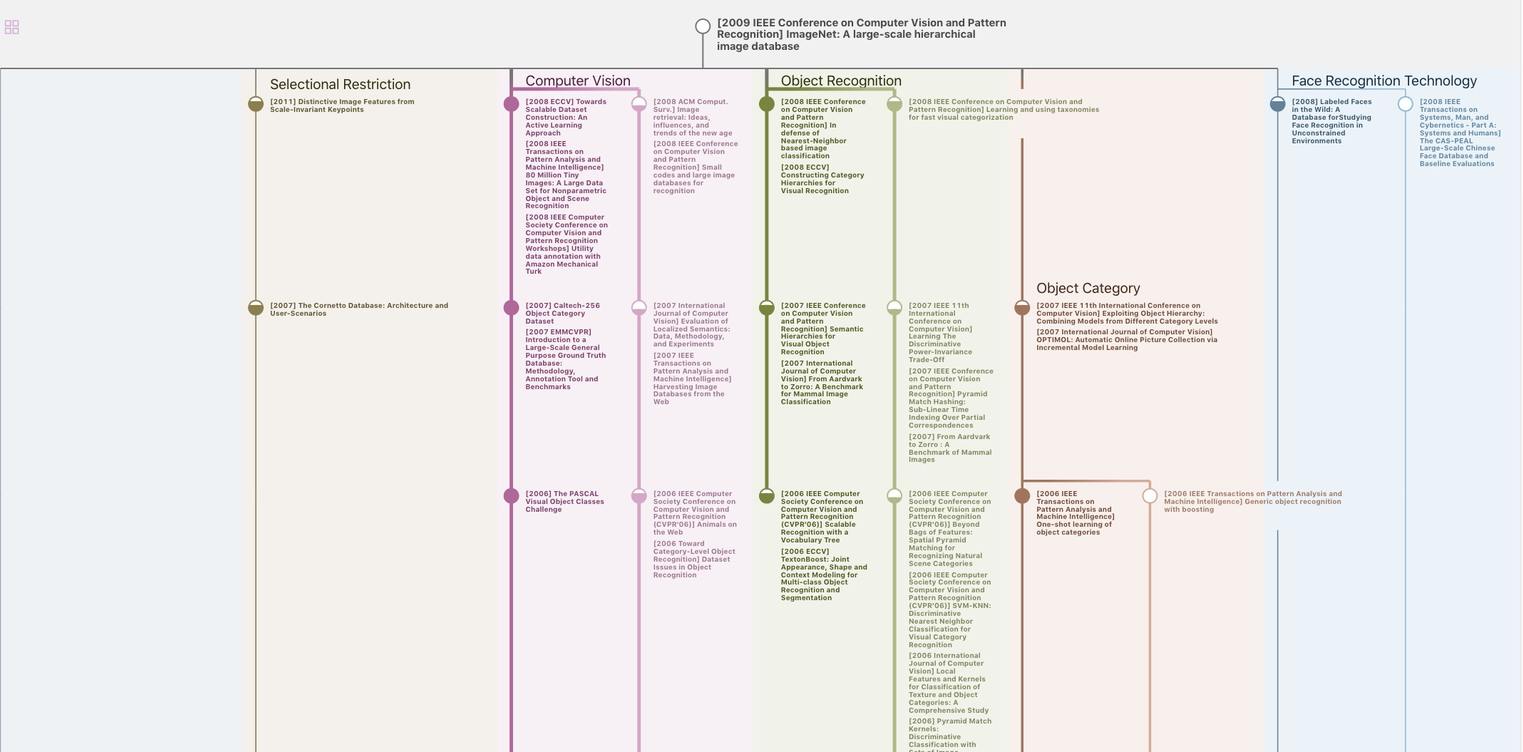
生成溯源树,研究论文发展脉络
Chat Paper
正在生成论文摘要