Simulation-free Reliability Analysis with Importance Sampling-based Adaptive Training Physics-informed Neural Networks: Method and Application to Chloride Penetration
Reliability Engineering & System Safety(2024)
摘要
Surrogate model-based reliability analysis aims at building a cheap-to-evaluate mathematical model as a substitute for the original performance function to enhance computational efficiency. Data-driven surrogate models, such as Kriging, Support Vector Machines and Polynomial Chaos Expansion, have been popularly studied from a point of active learning. On the other hand, Physics-informed Neural Networks, called PINNs, have recently gained much attention as a physical-informed surrogate model to directly solve partial differential equations. Building on the capability of avoiding the simulation of traditional numerical solvers such as finite element analysis, the PINN-based reliability analysis can achieve highly efficient simulation-free uncertainty quantification. This paper focuses on the development of the PINN-based reliability analysis method and its application in practical engineering applications. Reliability analysis with Importance sampling-based Adaptive Training Physics-informed Neural Networks (IAT-PINN-RA) is proposed in this work. Compared with the existing PINN-based reliability analysis method, IAT-PINN-RA introduces a pre-training stage for the establishment of the importance sampling distribution, and therefore achieves better performance when handling rare events. The modeling and reliability analysis of chloride penetration, which can pose serious challenges to the durability of concrete structures, are investigated. A practical example demonstrates the feasibility of using PINNs to model this physical phenomenon and the performance of the proposed method to achieve accurate and efficient reliability analysis results.
更多查看译文
关键词
Reliability analysis,Physics-informed neural networks,Active learning,Deep neural network,Importance Sampling
AI 理解论文
溯源树
样例
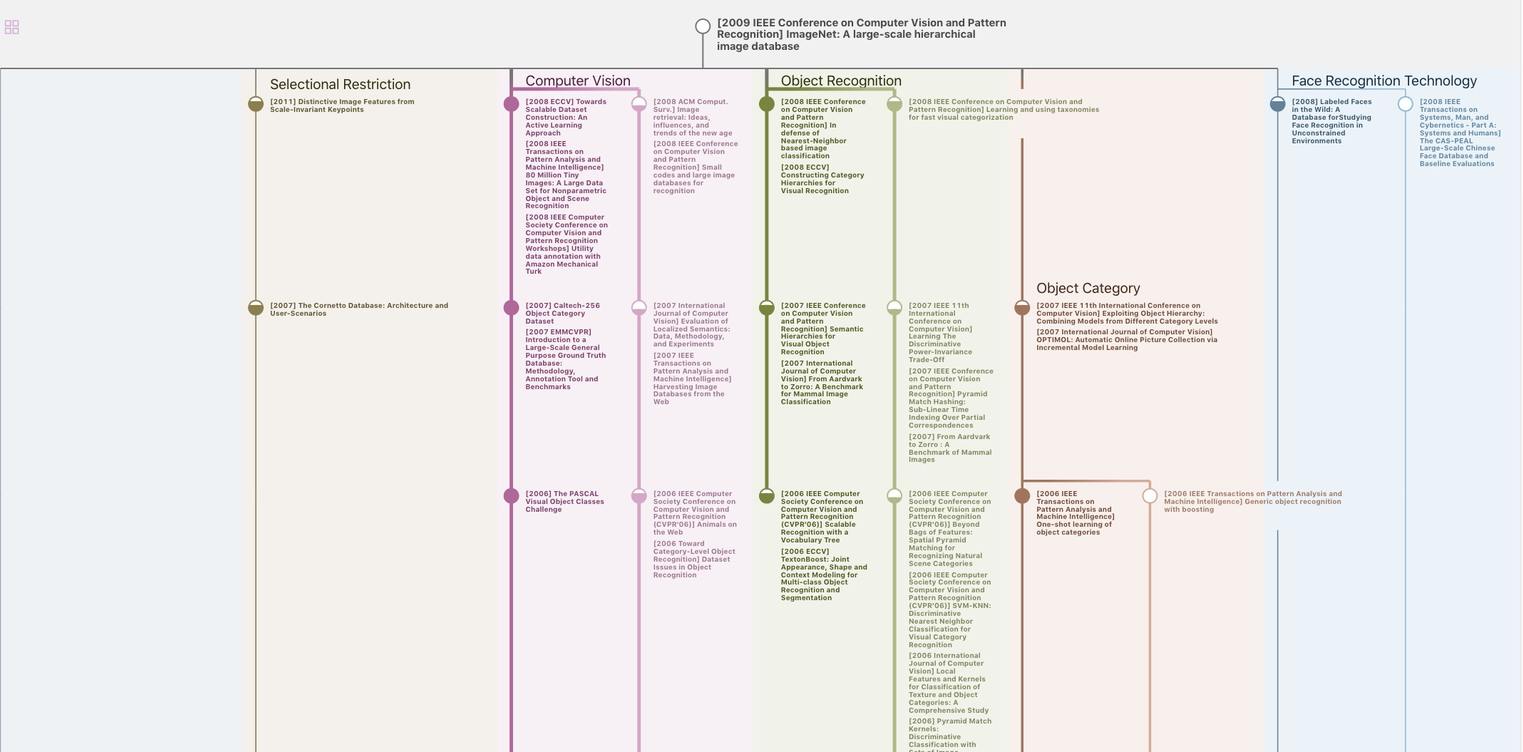
生成溯源树,研究论文发展脉络
Chat Paper
正在生成论文摘要