Joint Task and Computing Resource Allocation in Distributed Edge Computing Systems via Multi-Agent Deep Reinforcement Learning
IEEE Transactions on Network Science and Engineering(2024)
摘要
Edge servers can collaborate to enhance service capability. However, cloud servers may be unable to execute centralized management due to unpredictable communications. In such systems, distributed task and resource management are vital but challenging due to heterogeneity and various restrictions. Therefore, this paper studies such edge systems and formulates the distributed joint task and computing resource allocation problem for maximizing the quality of experience (QoE). Given the restrictions on real-time state observations and resource management involving other facilities, we decompose it into sub-problems of distributed task allocation and computing resource allocation. After formulating the problem as a partially observed Markov decision process, we propose a two-step approach that depends on multi-agent (MA) deep reinforcement learning. First, each edge server performs a policy to allocate tasks for its associated users according to a partial observation. We employ the MA deep deterministic policy gradient to tackle vast spaces of discrete actions. Besides, we incorporate the action entropy of massive users' task allocation to enhance exploration. Then, we prove that the QoE-maximized computing resource allocation is a problem of maxing a sum of sigmoids, and we address it by sigmoidal programming. Simulation results reveal that the proposed approach dramatically improves the system QoE and reduces the average service latency. Besides, the proposed solution outperforms benchmarks in training and convergence.
更多查看译文
关键词
Edge computing,distributed task allocation,resource allocation,quality of experience,multi-agent deep reinforcement learning
AI 理解论文
溯源树
样例
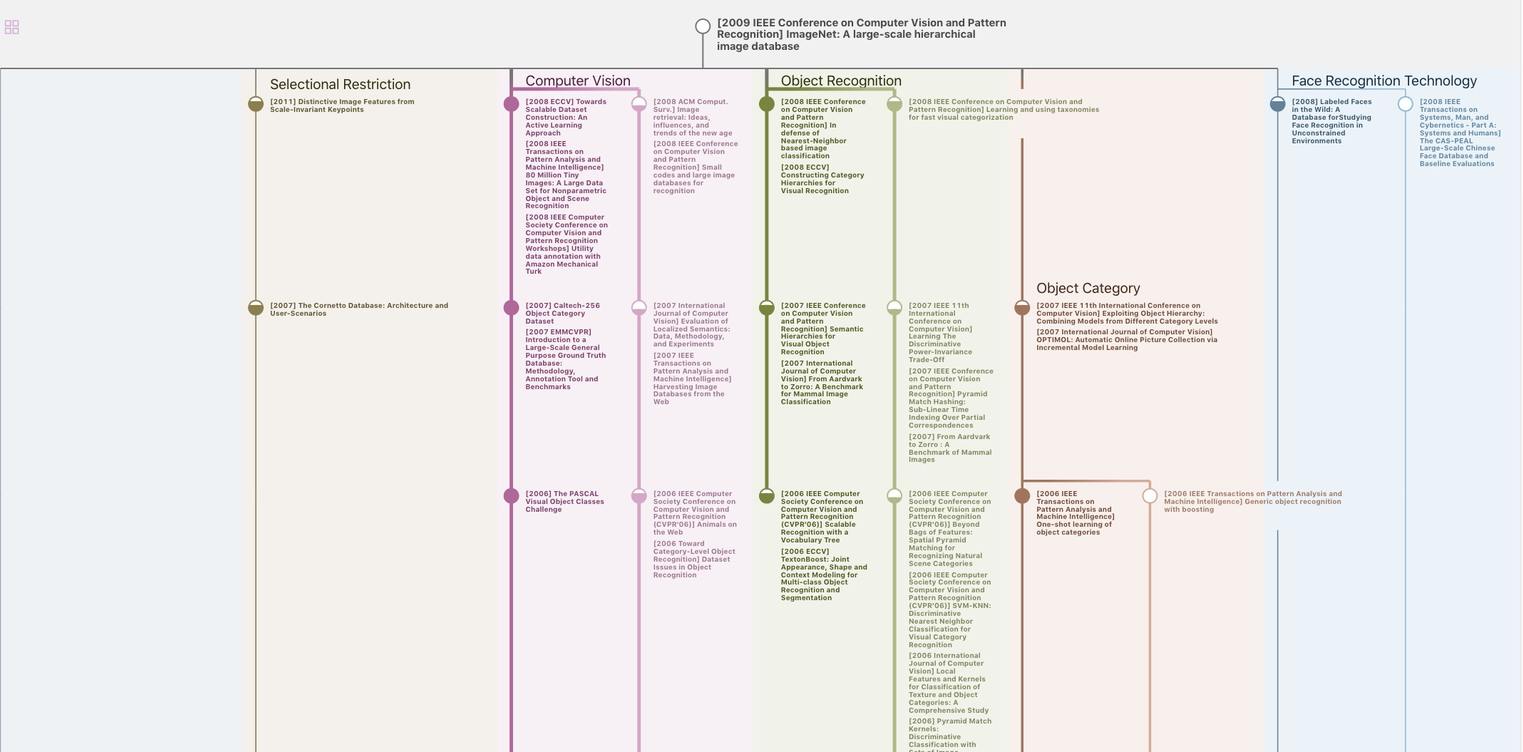
生成溯源树,研究论文发展脉络
Chat Paper
正在生成论文摘要