An eDRAM Based Computing-in-Memory Macro With Full-Valid-Storage and Channel-Wise-Parallelism for Depthwise Neural Network
IEEE Transactions on Circuits and Systems II: Express Briefs(2024)
摘要
Computing-in-memory (CIM) provides a highly efficient solution for neural networks in edge artificial intelligence applications. Most SRAM-based CIM designs can achieve high energy efficiency and area efficiency in standard convolutional layer’s multiply-and-accumulate (MAC) operations. However, when deploying depthwise separable convolution, they face several challenges. For these CIMs with weight-stationary, the lower activations reuse increases redundant memory reducing area efficiency and the fewer parameters of depthwise convolutional MAC decreases energy efficiency. To address these issues, we propose a depthwise separable convolutional computing-in-memory (DSC-CIM) that supports channel-wise parallel computation to increase area efficiency and energy efficiency. It includes three key techniques: (1) a 5T2C eDRAM bitcell for low power activation update and high area efficiency, (2) an independent update in the column direction to enable horizontal and vertical movement of the convolution window in the feature map, and (3) a data weight configuration circuit (DWCC) that supports both signed and unsigned parameters’ MAC operation. Layout post-simulations show that the proposed 28 nm DSC-CIM macro achieves an energy efficiency of 20.13 TOPS/W for 8b parameters on depthwise convolution. The inference accuracy on CIFAR-10 with 8b MobileNet-V2 model is 92.6%.
更多查看译文
关键词
computing-in-memory,eDRAM,depthwise convolution,energy efficiency,area efficiency
AI 理解论文
溯源树
样例
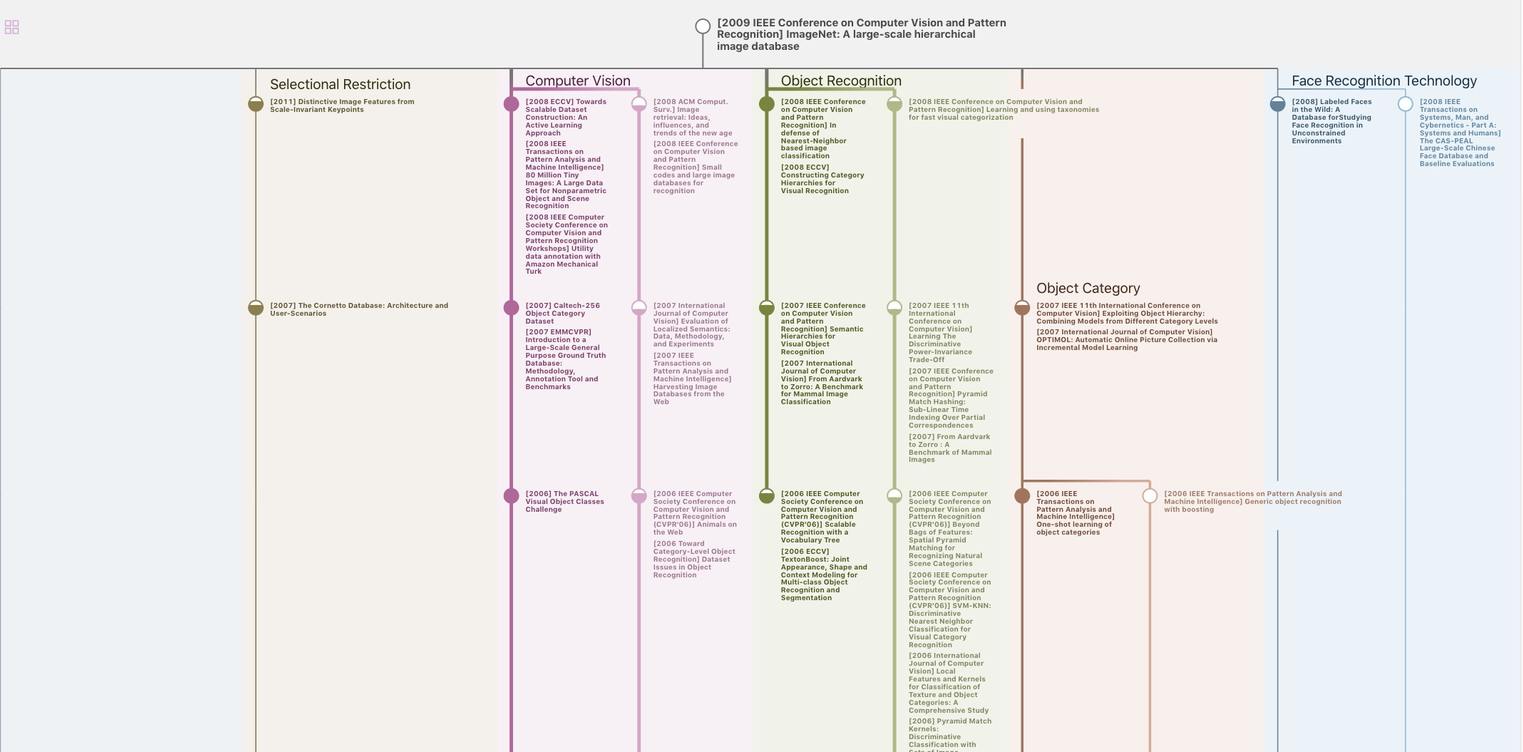
生成溯源树,研究论文发展脉络
Chat Paper
正在生成论文摘要