Combining machine learning with structure-based protein design to predict and engineer post-translational modifications of proteins
PLOS COMPUTATIONAL BIOLOGY(2024)
摘要
Post-translational modifications (PTMs) of proteins play a vital role in their function and stability. These modifications influence protein folding, signaling, protein-protein interactions, enzyme activity, binding affinity, aggregation, degradation, and much more. To date, over 400 types of PTMs have been described, representing chemical diversity well beyond the genetically encoded amino acids. Such modifications pose a challenge to the successful design of proteins, but also represent a major opportunity to diversify the protein engineering toolbox. To this end, we first trained artificial neural networks (ANNs) to predict eighteen of the most abundant PTMs, including protein glycosylation, phosphorylation, methylation, and deamidation. In a second step, these models were implemented inside the computational protein modeling suite Rosetta, which allows flexible combination with existing protocols to model the modified sites and understand their impact on protein stability as well as function. Lastly, we developed a new design protocol that either maximizes or minimizes the predicted probability of a particular site being modified. We find that this combination of ANN prediction and structure-based design can enable the modification of existing, as well as the introduction of novel, PTMs. The potential applications of our work include, but are not limited to, glycan masking of epitopes, strengthening protein-protein interactions through phosphorylation, as well as protecting proteins from deamidation liabilities. These applications are especially important for the design of new protein therapeutics where PTMs can drastically change the therapeutic properties of a protein. Our work adds novel tools to Rosetta's protein engineering toolbox that allow for the rational design of PTMs. Machine learning (ML) is changing the world of protein design, from structure prediction methods like AlphaFold to fixed-backbone design methods like ProteinMPNN. ML methods have made much progress in various aspects of protein computational biology, both complementing and, in some cases, surpassing traditional macromolecular modeling methods such as those combined in libraries like the Rosetta software suite. However, a lack of compatibility and flexibility can hinder interoperability with existing methods, preventing the full potential of these new solutions from being realized. Here, we first present a new machine learning tool for predicting post-translational modifications (PTMs), which play an important role in the stability and function of proteins, and then highlight how the implementation of this tool in the existing Rosetta toolbox can facilitate new applications. To this end, we combine PTM prediction with protein design, maximizing or minimizing the predicted probability of a post-translational modification occurring at a specific site. As one example, we predict the N-linked glycosylation of influenza hemagglutinin, which has applications in both understanding the evolution of viral strains over time, and engineering additional glycosylation sites to mask unwanted epitopes of vaccine candidates.
更多查看译文
AI 理解论文
溯源树
样例
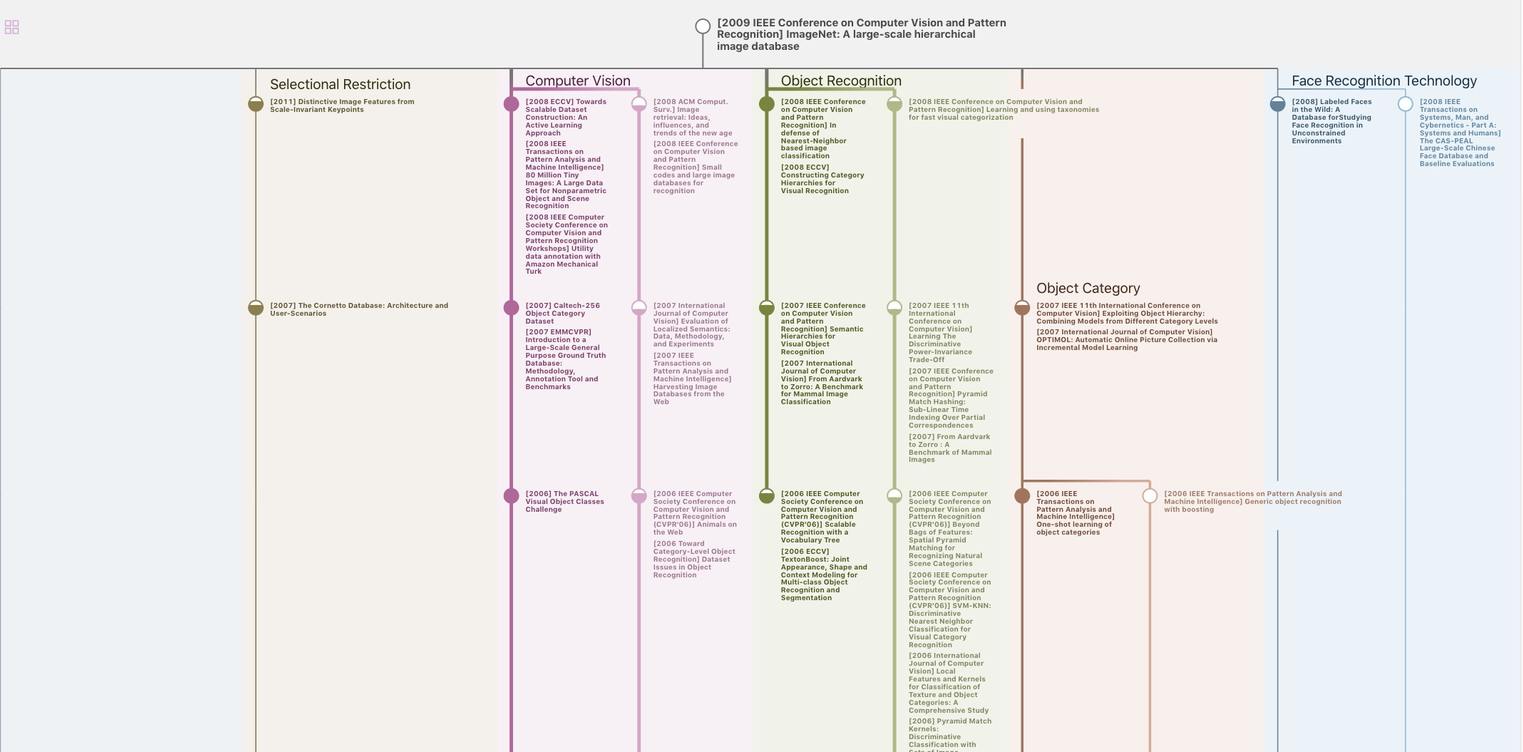
生成溯源树,研究论文发展脉络
Chat Paper
正在生成论文摘要