Taming Cross-Domain Representation Variance in Federated Prototype Learning with Heterogeneous Data Domains
arxiv(2024)
摘要
Federated learning (FL) allows collaborative machine learning training
without sharing private data. While most FL methods assume identical data
domains across clients, real-world scenarios often involve heterogeneous data
domains. Federated Prototype Learning (FedPL) addresses this issue, using mean
feature vectors as prototypes to enhance model generalization. However,
existing FedPL methods create the same number of prototypes for each client,
leading to cross-domain performance gaps and disparities for clients with
varied data distributions. To mitigate cross-domain feature representation
variance, we introduce FedPLVM, which establishes variance-aware dual-level
prototypes clustering and employs a novel α-sparsity prototype loss. The
dual-level prototypes clustering strategy creates local clustered prototypes
based on private data features, then performs global prototypes clustering to
reduce communication complexity and preserve local data privacy. The
α-sparsity prototype loss aligns samples from underrepresented domains,
enhancing intra-class similarity and reducing inter-class similarity.
Evaluations on Digit-5, Office-10, and DomainNet datasets demonstrate our
method's superiority over existing approaches.
更多查看译文
AI 理解论文
溯源树
样例
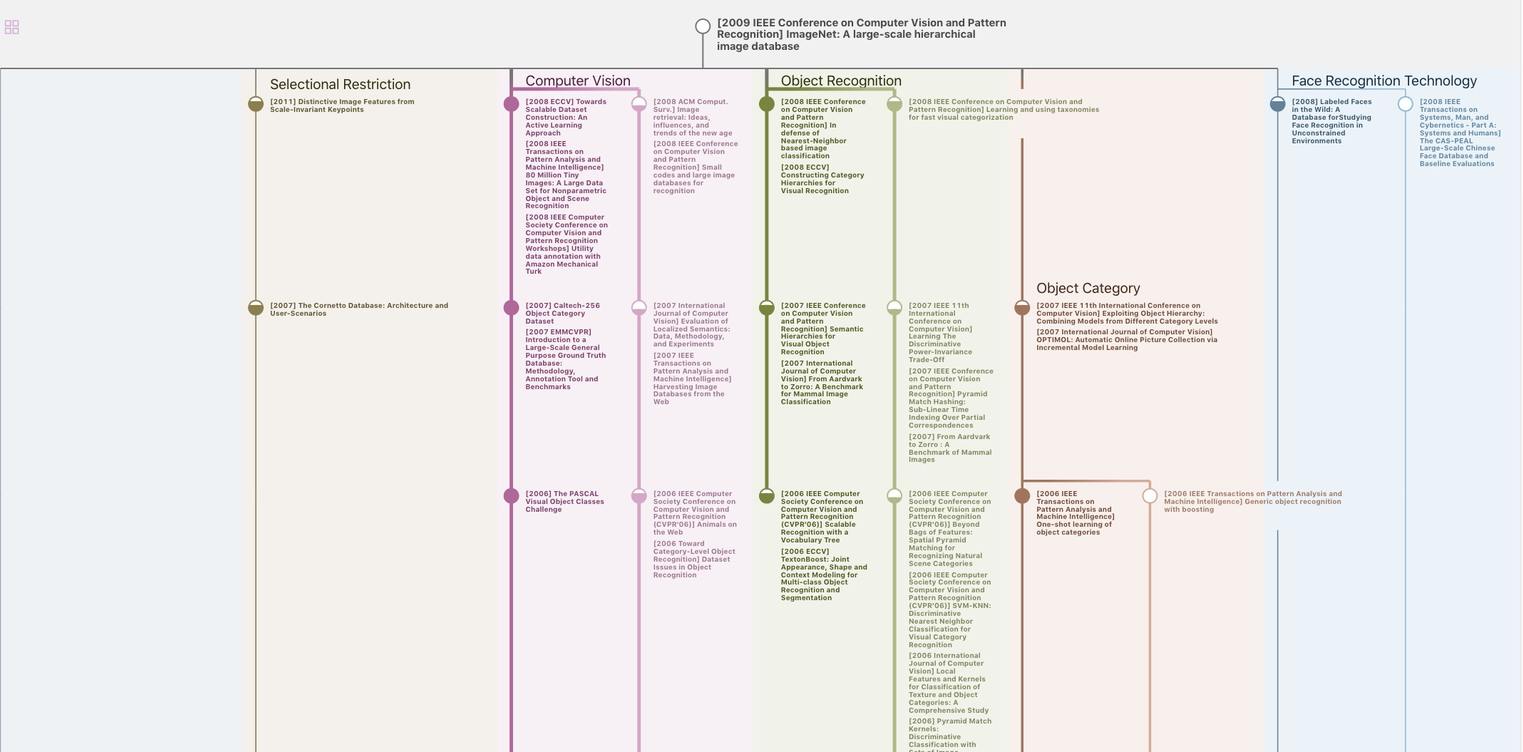
生成溯源树,研究论文发展脉络
Chat Paper
正在生成论文摘要