uaMix-MAE: Efficient Tuning of Pretrained Audio Transformers with Unsupervised Audio Mixtures
ICASSP 2024 - 2024 IEEE International Conference on Acoustics, Speech and Signal Processing (ICASSP)(2024)
摘要
Masked Autoencoders (MAEs) learn rich low-level representations from
unlabeled data but require substantial labeled data to effectively adapt to
downstream tasks. Conversely, Instance Discrimination (ID) emphasizes
high-level semantics, offering a potential solution to alleviate annotation
requirements in MAEs. Although combining these two approaches can address
downstream tasks with limited labeled data, naively integrating ID into MAEs
leads to extended training times and high computational costs. To address this
challenge, we introduce uaMix-MAE, an efficient ID tuning strategy that
leverages unsupervised audio mixtures. Utilizing contrastive tuning, uaMix-MAE
aligns the representations of pretrained MAEs, thereby facilitating effective
adaptation to task-specific semantics. To optimize the model with small amounts
of unlabeled data, we propose an audio mixing technique that manipulates audio
samples in both input and virtual label spaces. Experiments in low/few-shot
settings demonstrate that achieves 4-6
various benchmarks when tuned with limited unlabeled data, such as
AudioSet-20K. Code is available at https://github.com/PLAN-Lab/uamix-MAE
更多查看译文
关键词
Masked audio models,Contrastive tuning,Few-shot learning,Masked autoencoders
AI 理解论文
溯源树
样例
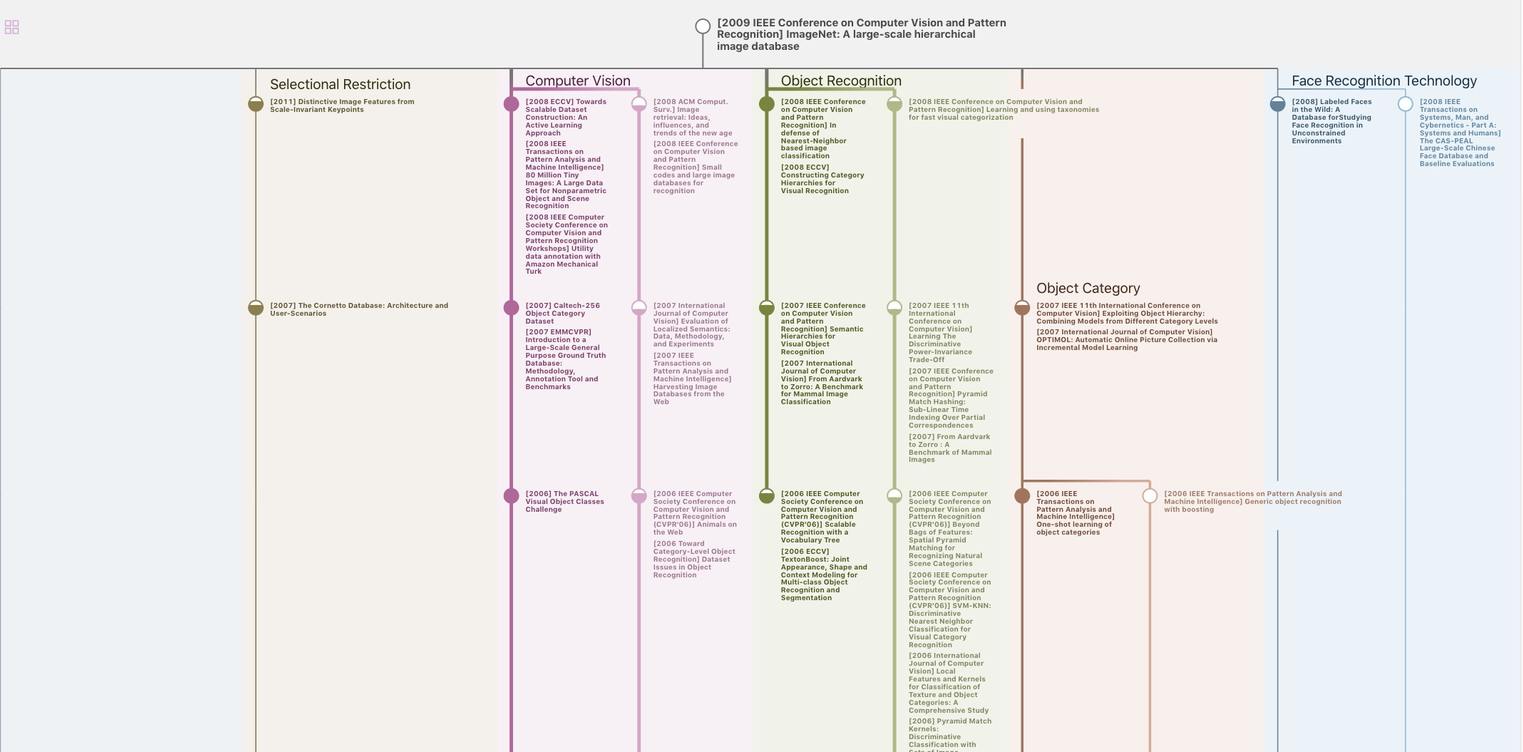
生成溯源树,研究论文发展脉络
Chat Paper
正在生成论文摘要