Disparities in seizure outcomes revealed by large language models
JOURNAL OF THE AMERICAN MEDICAL INFORMATICS ASSOCIATION(2024)
摘要
Objective Large-language models (LLMs) can potentially revolutionize health care delivery and research, but risk propagating existing biases or introducing new ones. In epilepsy, social determinants of health are associated with disparities in care access, but their impact on seizure outcomes among those with access remains unclear. Here we (1) evaluated our validated, epilepsy-specific LLM for intrinsic bias, and (2) used LLM-extracted seizure outcomes to determine if different demographic groups have different seizure outcomes.Materials and Methods We tested our LLM for differences and equivalences in prediction accuracy and confidence across demographic groups defined by race, ethnicity, sex, income, and health insurance, using manually annotated notes. Next, we used LLM-classified seizure freedom at each office visit to test for demographic outcome disparities, using univariable and multivariable analyses.Results We analyzed 84 675 clinic visits from 25 612 unique patients seen at our epilepsy center. We found little evidence of bias in the prediction accuracy or confidence of outcome classifications across demographic groups. Multivariable analysis indicated worse seizure outcomes for female patients (OR 1.33, P <= .001), those with public insurance (OR 1.53, P <= .001), and those from lower-income zip codes (OR >= 1.22, P <= .007). Black patients had worse outcomes than White patients in univariable but not multivariable analysis (OR 1.03, P = .66).Conclusion We found little evidence that our LLM was intrinsically biased against any demographic group. Seizure freedom extracted by LLM revealed disparities in seizure outcomes across several demographic groups. These findings quantify the critical need to reduce disparities in the care of people with epilepsy.
更多查看译文
关键词
electronic health record,natural language processing,clinical informatics,health disparities
AI 理解论文
溯源树
样例
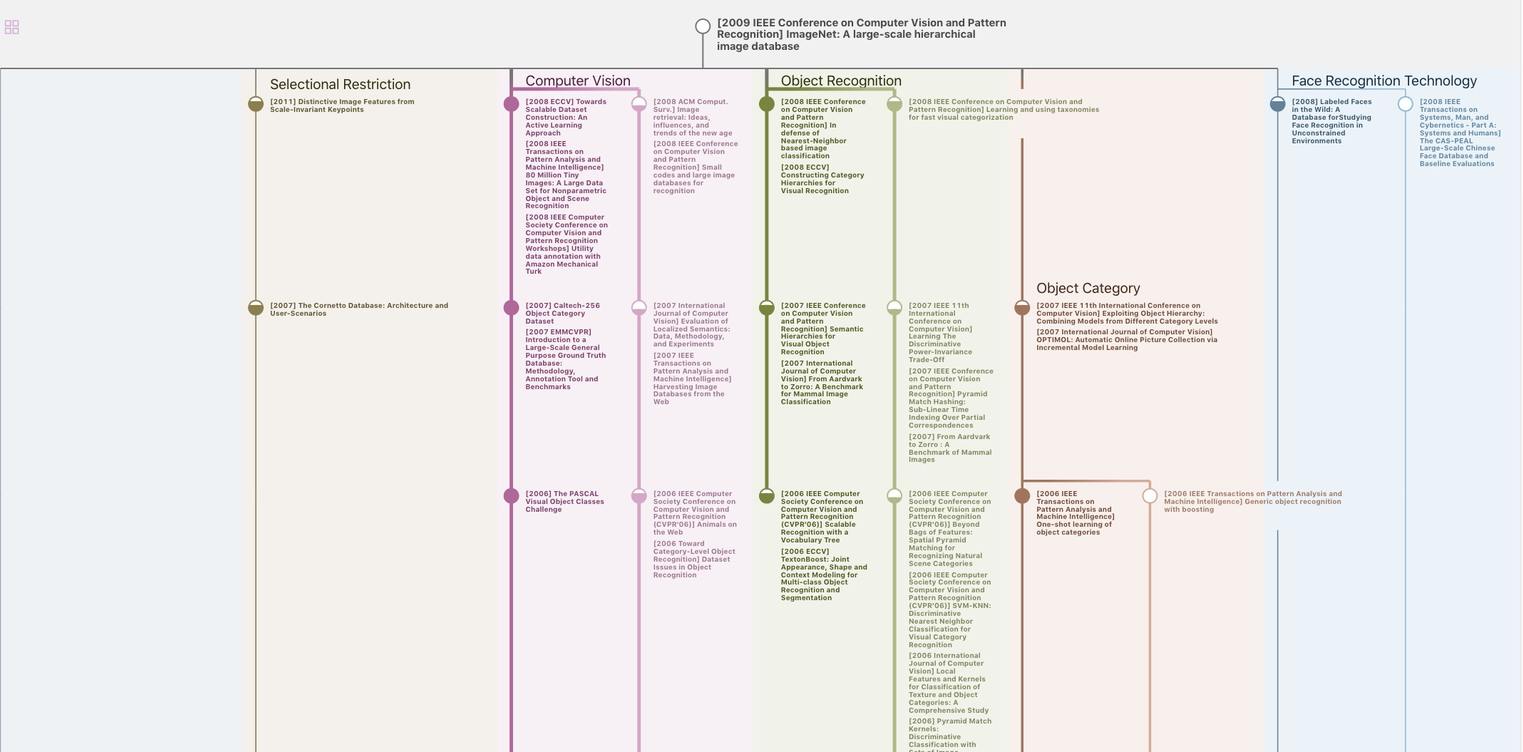
生成溯源树,研究论文发展脉络
Chat Paper
正在生成论文摘要