Model Compression by Count-Sketch for Over-the-Air Stateless Federated Learning
IEEE Internet of Things Journal(2024)
摘要
Motivated by the rapidly increasing computing performance of devices and the abundance of device-generated data, federated learning (FL) has emerged as a new distributed machine learning (ML) scheme with a wide range of applications. However, it is well-known that FL might be severely degraded by communication overhead, as it heavily relies on communication between clients and a central server. To overcome this communication bottleneck, the wireless communication community has explored AirComp FL, applying Over-the-Air Computation (AirComp) for model aggregation. In this paper, we introduce a novel AirComp FL algorithm, A-FedCS, which utilizes count-sketch (CS) for model compression. A-FedCS exhibits scalability, addressing challenges faced by existing approaches struggling with scarce channel resources or rarely revisiting clients. Experimental results demonstrate that the proposed scheme outperforms state-of-the-art schemes, including CA-DSGD and D-DSGD. We show that the improvement is more significant in stateless FL through experiments with various settings of tasks, transmission power, bandwidth, and the number of clients. Additionally, we provide a mathematical analysis of A-FedCS by deriving its convergence rate.
更多查看译文
关键词
Federated learning,Over-the-Air computation,Count-sketch,Stateless federated learning
AI 理解论文
溯源树
样例
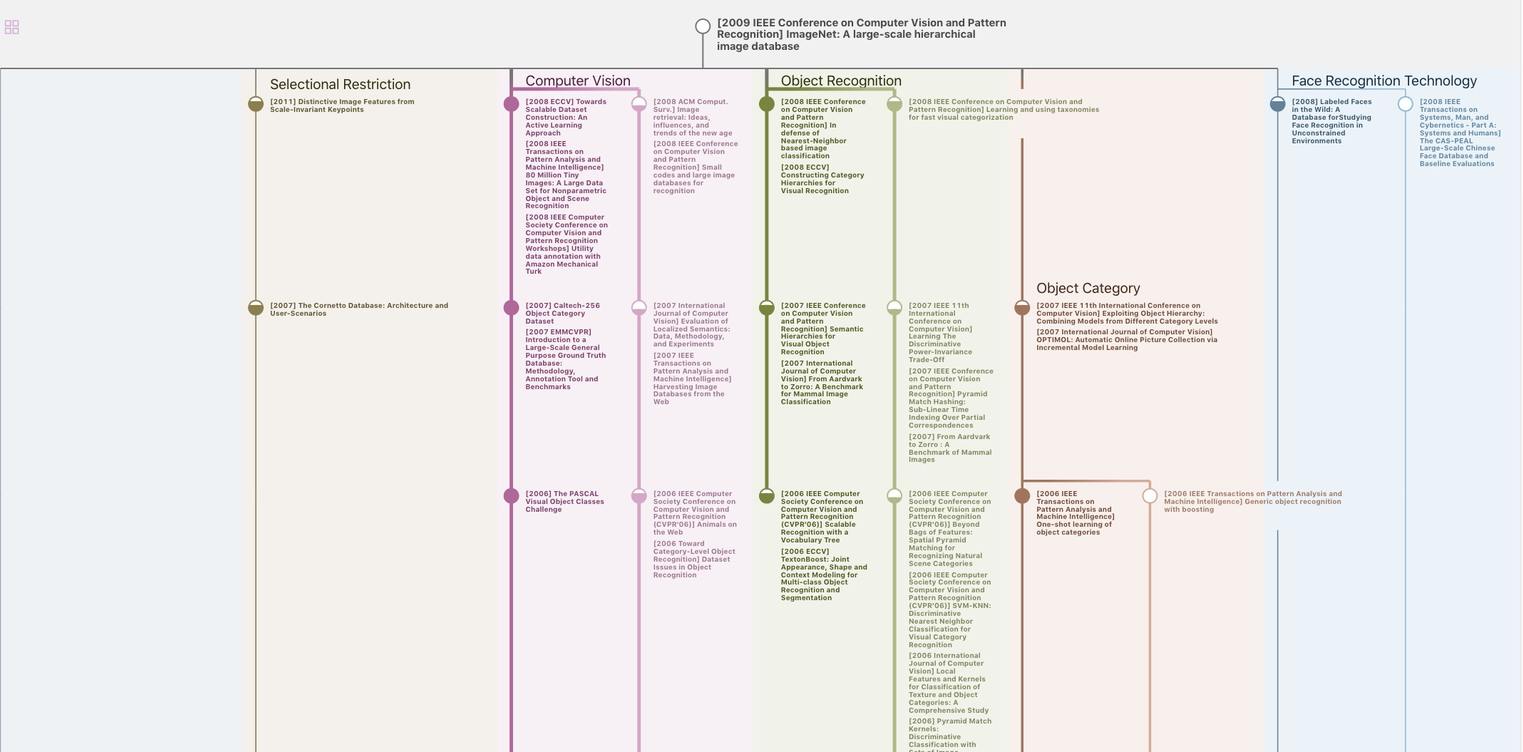
生成溯源树,研究论文发展脉络
Chat Paper
正在生成论文摘要