6.9 A 0.35V 0.367TOPS/W Image Sensor with 3-Layer Optical-Electronic Hybrid Convolutional Neural Network
2024 IEEE International Solid-State Circuits Conference (ISSCC)(2024)
摘要
Traditional computer-vision technology that relies on image sensors coupled with cloud processing or on-chip Artificial Intelligence (AI) processors have encountered significant challenges in terms of power consumption, delays arising from data transmission, and/or memory access. In-sensor and near-sensor computing have been reported to solve this issue by applying pixel or array level feature extraction [2–6]. In [2, 4], capacitors are utilized for analog-domain Haar filtering to reduce the processing power consumption, but sacrificing the Fill Factor (FF) due to the use of the capacitor array [2], or complicated pixel-level logic [4]. An image sensor in introduced in [3] with Hog feature-based object detection, achieving both low power and high accuracy for the detection of up to three object classes. However, it does not work on more complex tasks. Convolutional Neural Networks (CNNs) [5, 6] offer enhanced accuracy in practical applications, but increase the power consumption and circuit complexity. Optical-domain processing [7] is capable of parallel information processing through an optical architecture based on free space. However, in [7] this is based on diffraction and requires coherent light as input, which constrains the practicality for edge computing. This paper presents a Pulse Width Modulation (PWM) pixel-based image sensor array that integrates an optical-electronic hybrid 3-layer convolutional processing unit. The first layer of the convolution is performed in the optical domain without introducing extra power consumption. The integration of optical convolution with electrical convolution offers the potential to leverage the energy-efficient advantages of optical convolution, while simultaneously providing the essential programmability needed to address multiple use-cases through reprogramming within a single device. A dual-mode Processing Element (PE) is proposed enables both acquisition mode and calculation mode. The integration of a sum/differential dual-counter design and the incorporation of two different pixel reset methods are employed in the co-design of PWM pixels and PEs to effectively mitigate computational power consumption.
更多查看译文
关键词
Convolutional Neural Network,Image Sensor,Reprogramming,Convolutional Layers,Power Consumption,Fabrication Process,Free Space,Pulse Width,Data Transmission,Object Classification,Convolution Kernel,Acquisition Mode,Detection Power,Sensor Array,Pixel Level,Edge Computing,Adjacent Pixels,Complex Circuits,Optical Character Recognition
AI 理解论文
溯源树
样例
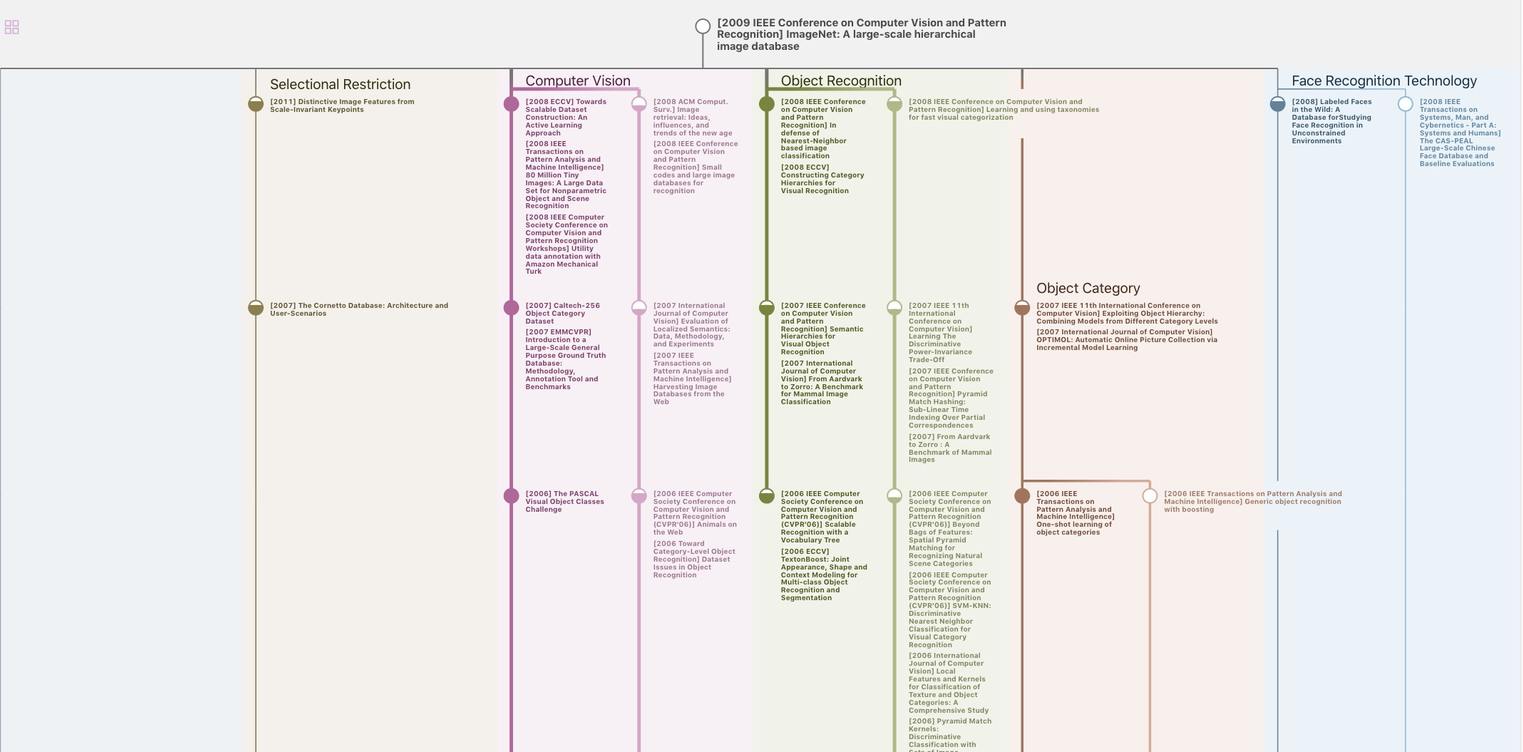
生成溯源树,研究论文发展脉络
Chat Paper
正在生成论文摘要