Automatic Detection and Tracking of Anatomical Landmarks in Transesophageal Echocardiography for Quantification of Left Ventricular Function
Ultrasound in Medicine & Biology(2024)
摘要
Objective
Evaluation of left ventricular (LV) function in critical care patients is useful for guidance of therapy and early detection of LV dysfunction, but the tools currently available are too time-consuming. To resolve this issue, we previously proposed a method for the continuous and automatic quantification of global LV function in critical care patients based on the detection and tracking of anatomical landmarks on transesophageal heart ultrasound. In the present study, our aim was to improve the performance of mitral annulus detection in transesophageal echocardiography (TEE).
Methods
We investigated several state-of-the-art networks for both the detection and tracking of the mitral annulus in TEE. We integrated the networks into a pipeline for automatic assessment of LV function through estimation of the mitral annular plane systolic excursion (MAPSE), called autoMAPSE. TEE recordings from a total of 245 patients were collected from St. Olav's University Hospital and used to train and test the respective networks. We evaluated the agreement between autoMAPSE estimates and manual references annotated by expert echocardiographers in 30 Echolab patients and 50 critical care patients. Furthermore, we proposed a prototype of autoMAPSE for clinical integration and tested it in critical care patients in the intensive care unit.
Results
Compared with manual references, we achieved a mean difference of 0.8 (95% limits of agreement: –2.9 to 4.7) mm in Echolab patients, with a feasibility of 85.7%. In critical care patients, we reached a mean difference of 0.6 (95% limits of agreement: –2.3 to 3.5) mm and a feasibility of 88.1%. The clinical prototype of autoMAPSE achieved real-time performance.
Conclusion
Automatic quantification of LV function had high feasibility in clinical settings. The agreement with manual references was comparable to inter-observer variability of clinical experts.
更多查看译文
关键词
Echocardiography,Ultrasound,Transesophageal echocardiography,Deep learning,Machine learning,Vision transformer,Convolutional neural network,Mitral annular plane systolic excursion
AI 理解论文
溯源树
样例
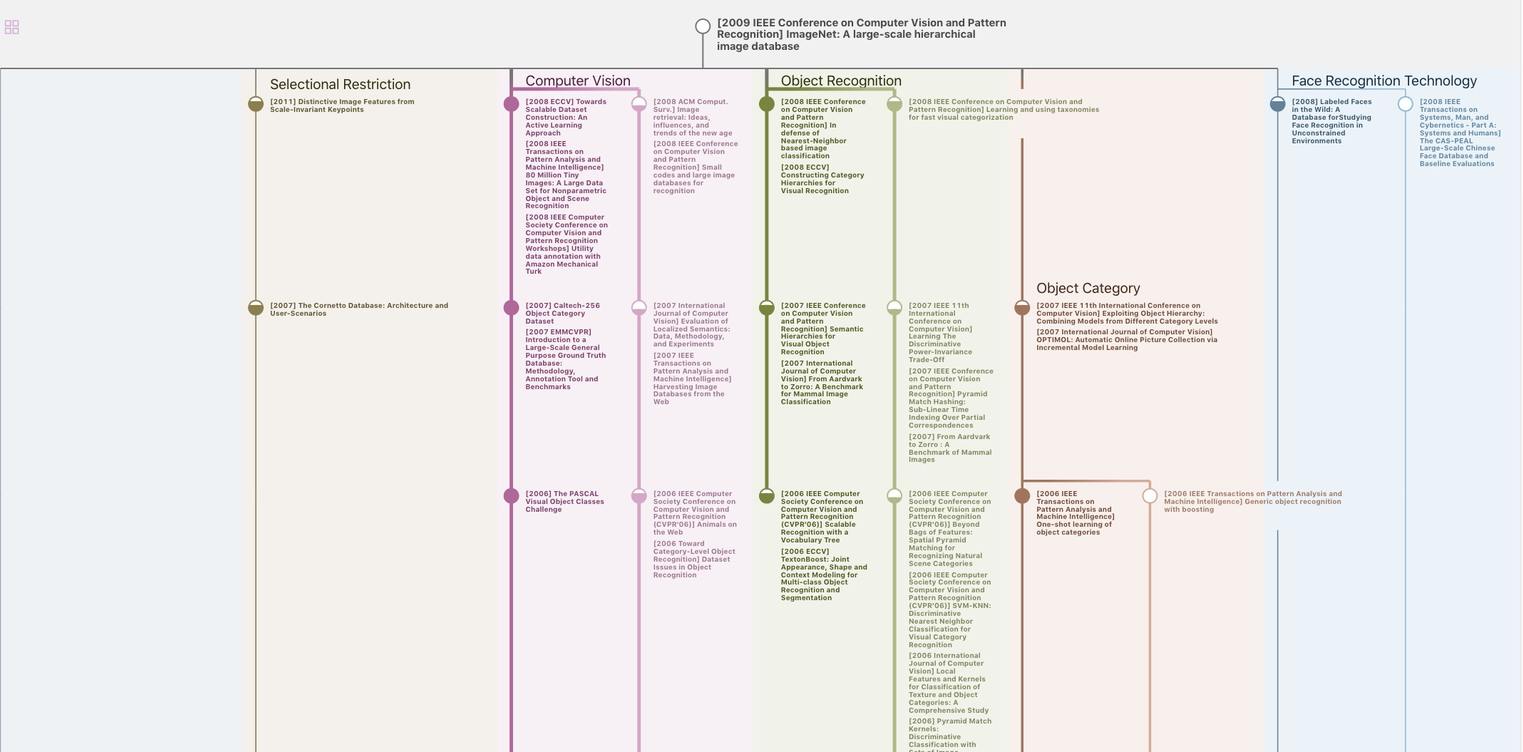
生成溯源树,研究论文发展脉络
Chat Paper
正在生成论文摘要