MedChatZH: A tuning LLM for traditional Chinese medicine consultations
Computers in Biology and Medicine(2024)
摘要
Generative Large Language Models (LLMs) have achieved significant success in various natural language processing tasks, including Question-Answering (QA) and dialogue systems. However, most models are trained on English data and lack strong generalization in providing answers in Chinese. This limitation is especially evident in specialized domains like traditional Chinese medical QA, where performance suffers due to the absence of fine-tuning and high-quality datasets. To address this, we introduce MedChatZH, a dialogue model optimized for Chinese medical QA based on transformer decoder with LLaMA architecture. Continued pre-training on a curated corpus of Chinese medical books is followed by fine-tuning with a carefully selected medical instruction dataset, resulting in MedChatZH outperforming several Chinese dialogue baselines on a real-world medical dialogue dataset. Our model, code, and dataset are publicly available on GitHub (https://github.com/tyang816/MedChatZH) to encourage further research in traditional Chinese medicine and LLMs.
更多查看译文
关键词
Generative large language models (LLMs),Question-answering (QA),Dialogue model,Traditional Chinese medical QA,Fine-tuning
AI 理解论文
溯源树
样例
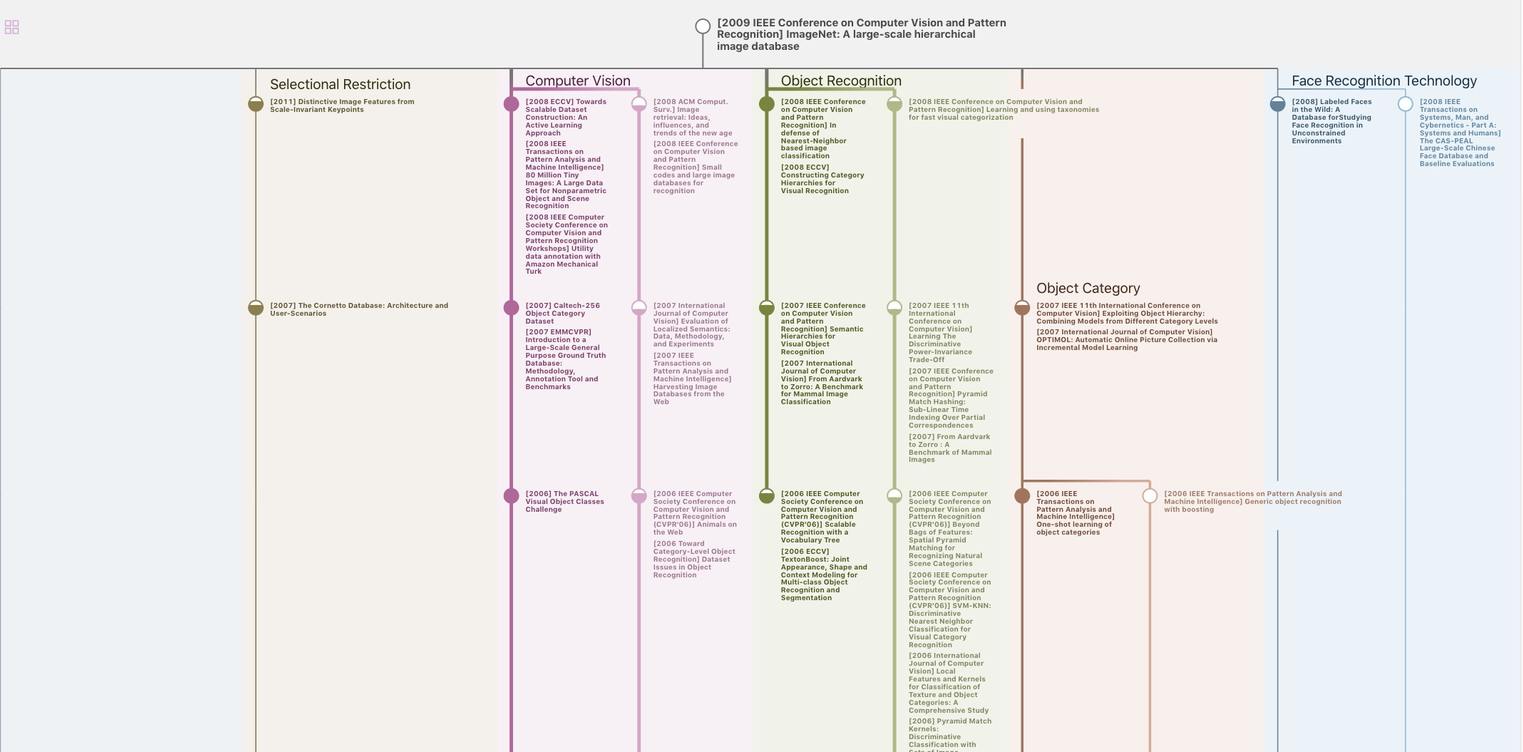
生成溯源树,研究论文发展脉络
Chat Paper
正在生成论文摘要