MedInsight: A Multi-Source Context Augmentation Framework for Generating Patient-Centric Medical Responses using Large Language Models
CoRR(2024)
摘要
Large Language Models (LLMs) have shown impressive capabilities in generating
human-like responses. However, their lack of domain-specific knowledge limits
their applicability in healthcare settings, where contextual and comprehensive
responses are vital. To address this challenge and enable the generation of
patient-centric responses that are contextually relevant and comprehensive, we
propose MedInsight:a novel retrieval augmented framework that augments LLM
inputs (prompts) with relevant background information from multiple sources.
MedInsight extracts pertinent details from the patient's medical record or
consultation transcript. It then integrates information from authoritative
medical textbooks and curated web resources based on the patient's health
history and condition. By constructing an augmented context combining the
patient's record with relevant medical knowledge, MedInsight generates
enriched, patient-specific responses tailored for healthcare applications such
as diagnosis, treatment recommendations, or patient education. Experiments on
the MTSamples dataset validate MedInsight's effectiveness in generating
contextually appropriate medical responses. Quantitative evaluation using the
Ragas metric and TruLens for answer similarity and answer correctness
demonstrates the model's efficacy. Furthermore, human evaluation studies
involving Subject Matter Expert (SMEs) confirm MedInsight's utility, with
moderate inter-rater agreement on the relevance and correctness of the
generated responses.
更多查看译文
AI 理解论文
溯源树
样例
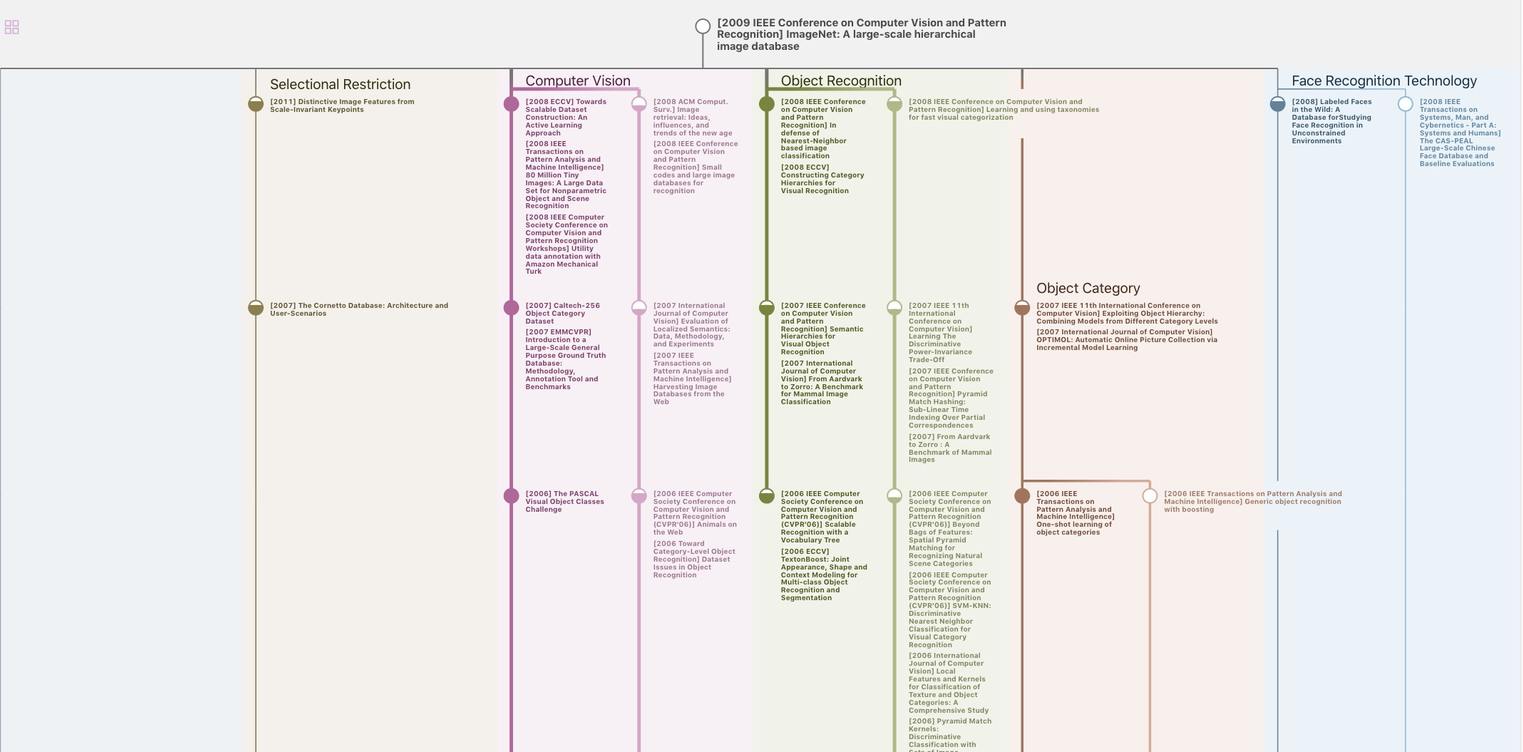
生成溯源树,研究论文发展脉络
Chat Paper
正在生成论文摘要