Probabilistic Metaplasticity for Continual Learning with Memristors
arxiv(2024)
摘要
Crossbar architectures utilizing memristor devices hold promise to address
continual learning challenges in resource-constrained edge devices. However,
these nanoscale devices often exhibit low precision and high variability in
conductance modulation, rendering them unsuitable for continual learning
solutions that consolidate weights through precise modulation. This issue can
be circumvented by accumulating weight gradients in auxiliary high-precision
memory and updating memristor weights when gradients are equivalent to
memristor weight resolution. However, it leads to frequent memory access, high
memory overhead, and energy dissipation. In this research, we propose
probabilistic metaplasticity, which consolidates weights by modulating their
update probability rather than magnitude. The proposed mechanism eliminates
high-precision modification to weight magnitude and consequently,
high-precision memory for gradient accumulation. We demonstrate the efficacy of
the proposed mechanism by integrating probabilistic metaplasticity into a
spiking network trained on an error threshold with low-precision memristor
weights. Evaluations of two continual learning benchmarks show that
probabilistic metaplasticity consumes 67
parameters and up to two orders of magnitude lower energy during parameter
updates compared to an auxiliary memory-based solution while achieving
state-of-the-art performance. The proposed model shows potential for
energy-efficient continual learning with low-precision emerging devices.
更多查看译文
AI 理解论文
溯源树
样例
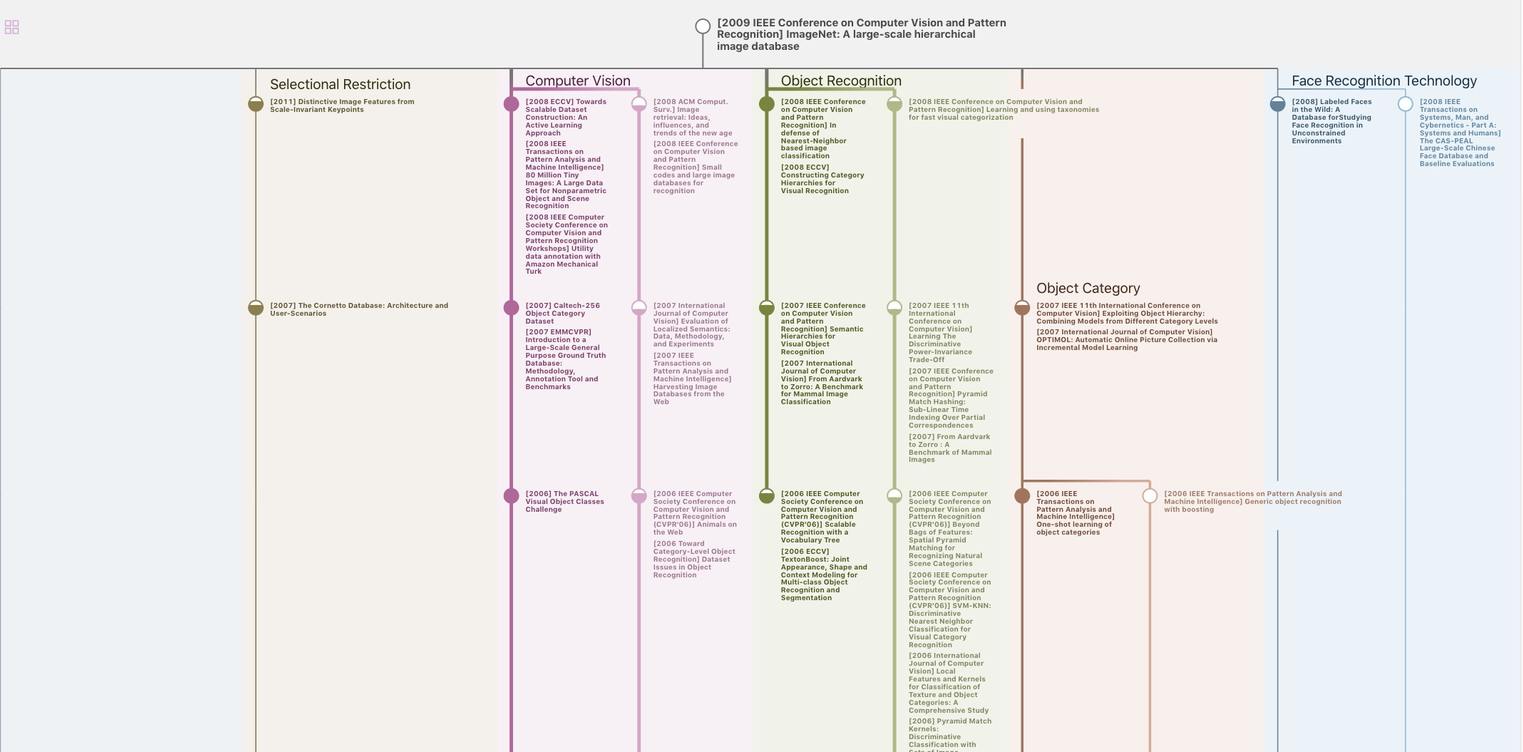
生成溯源树,研究论文发展脉络
Chat Paper
正在生成论文摘要