34.3 A 22nm 64kb Lightning-Like Hybrid Computing-in-Memory Macro with a Compressed Adder Tree and Analog-Storage Quantizers for Transformer and CNNs.
IEEE International Solid-State Circuits Conference(2024)
摘要
SRAM-based computing-in-memory (CIM) has made significant progress in improving the energy efficiency (EF) of neural operators, specifically MAC, used in AI applications. Prior CIM methods have demonstrated attractive energy efficiencies (EF) under a fixed/less of accumulation length, sparsity, toggle rate, and bit precision [1] –[6]. Analog CIMs (ACIM) offer potentially higher EF but are susceptible to PVT variations. On the other hand, digital CIMs (DCIM) are robust but provide moderate energy efficiency. In prior weight-wise-cut structures [1], when processing INT8 MUL operations, a calculation error occurred as the low-place-value weight directly influences the results, which leads to accuracy loss. In prior vertical-cut structures [2], more digital components are used to ensure inference accuracy; however, 64 local-computing cells are required for each bank, which results in a large area overhead and power consumption. As depicted in Fig. 34.3.1, challenges arise when applying these approaches to higher bitprecision MAC operations: (1) in balancing tradeoffs between inference accuracy and the area/energy overhead of hybrid analog-digital CIM; (2) the significant energy consumption and error accumulation of the readout circuit in ACIM; and (3) the limited EF and DCIM performance due to the large-scale adder tree.
更多查看译文
关键词
Adder Tree,Energy Efficiency,Power Consumption,Calculation Error,Compressor,Artificial Intelligence Applications,Inference Accuracy,Truth Table,Energy Error,Sparse Regularization,Area Overhead,Vision Transformer,Minimum Efficiency,Moderate Efficiency,Digital Components,Energy Efficiency Performance,Maximum Energy Efficiency,Large Power Consumption,NOR Gate
AI 理解论文
溯源树
样例
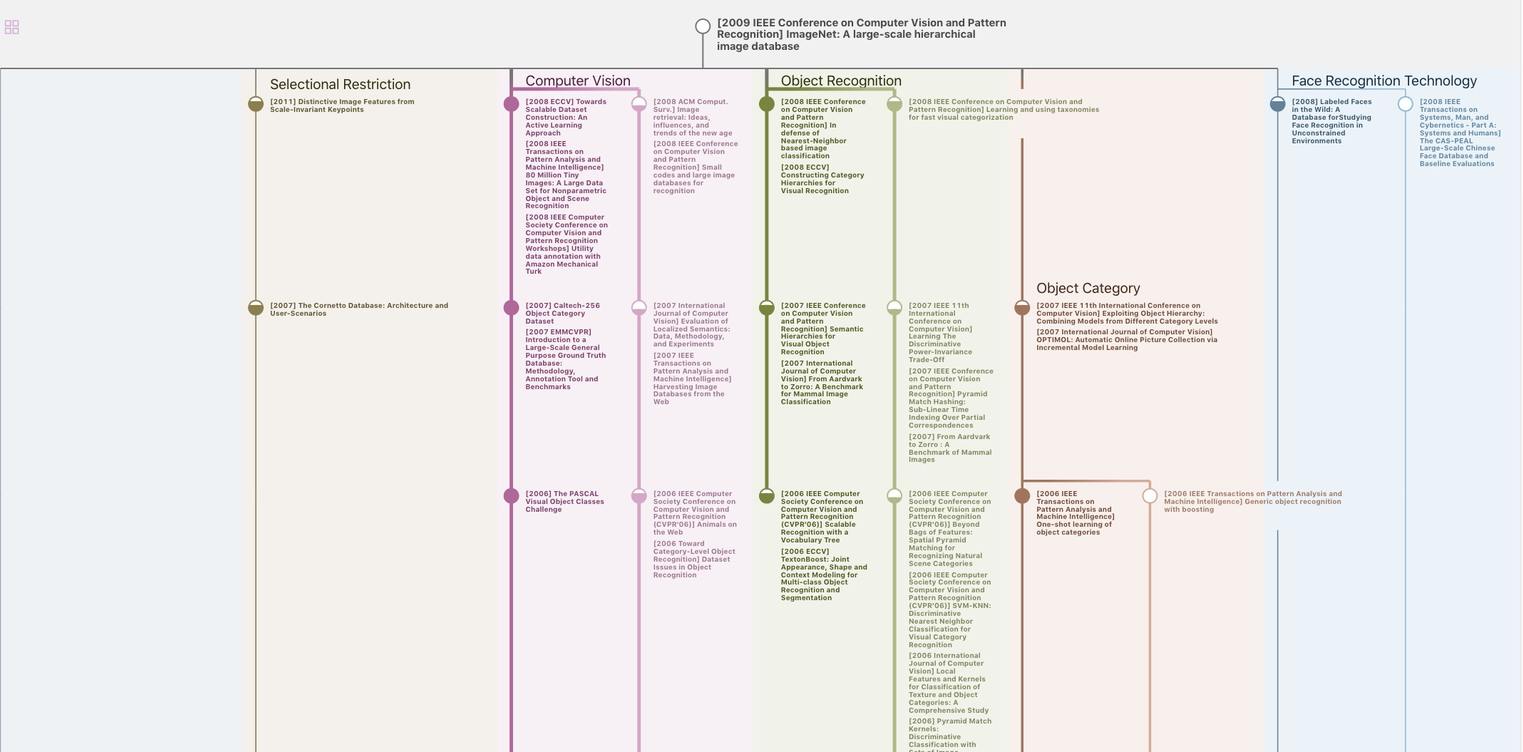
生成溯源树,研究论文发展脉络
Chat Paper
正在生成论文摘要