E-MuLA: An Ensemble Multi-Localized Attention Feature Extraction Network for Viral Protein Subcellular Localization
INFORMATION(2024)
摘要
Accurate prediction of subcellular localization of viral proteins is crucial for understanding their functions and developing effective antiviral drugs. However, this task poses a significant challenge, especially when relying on expensive and time-consuming classical biological experiments. In this study, we introduced a computational model called E-MuLA, based on a deep learning network that combines multiple local attention modules to enhance feature extraction from protein sequences. The superior performance of the E-MuLA has been demonstrated through extensive comparisons with LSTM, CNN, AdaBoost, decision trees, KNN, and other state-of-the-art methods. It is noteworthy that the E-MuLA achieved an accuracy of 94.87%, specificity of 98.81%, and sensitivity of 84.18%, indicating that E-MuLA has the potential to become an effective tool for predicting virus subcellular localization.
更多查看译文
关键词
subcellular localization,multi-classification,viral protein,deep learning
AI 理解论文
溯源树
样例
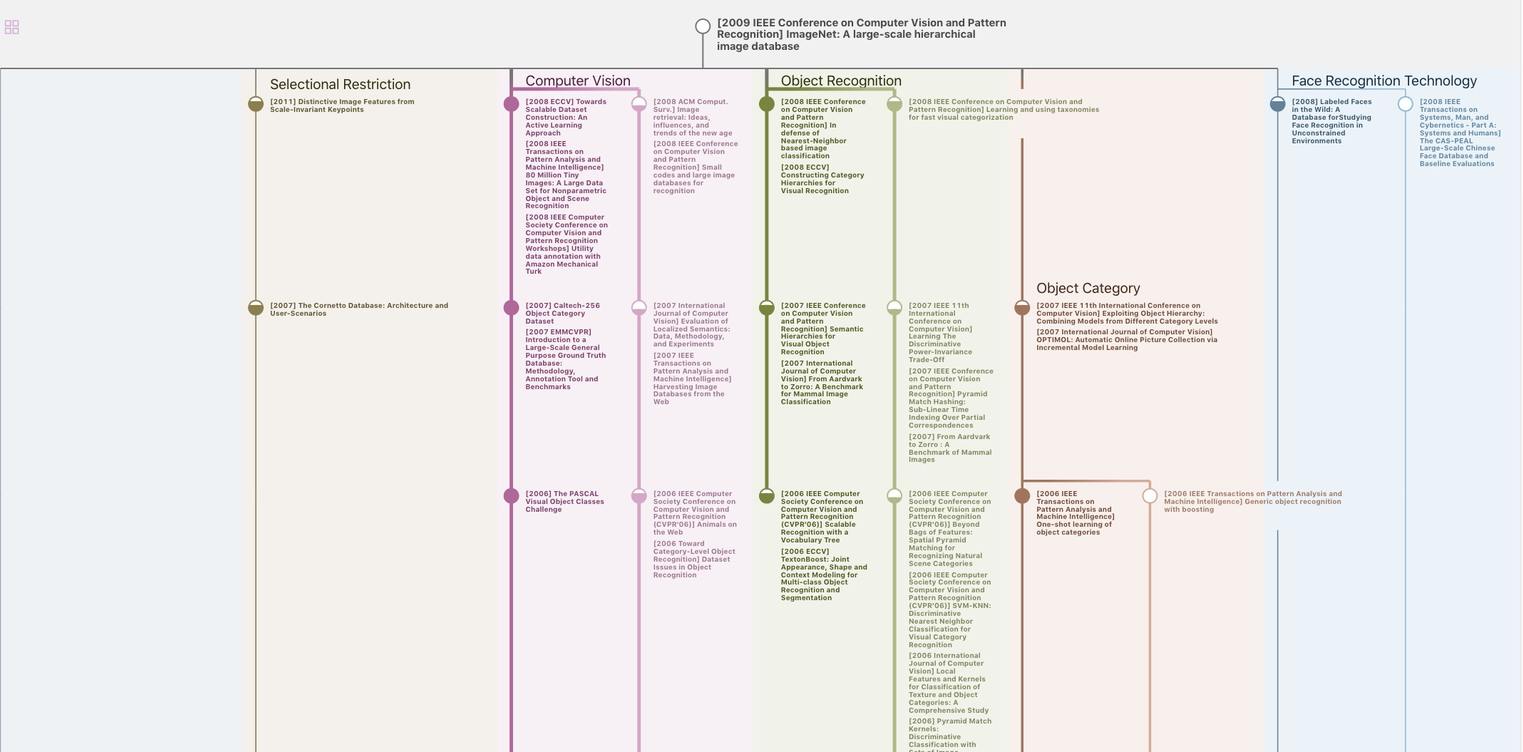
生成溯源树,研究论文发展脉络
Chat Paper
正在生成论文摘要