Machine learning in action: Revolutionizing intracranial hematoma detection and patient transport decision-making.
Journal of neurosciences in rural practice(2023)
摘要
Objectives:Traumatic intracranial hematomas represent a critical clinical situation where early detection and management are of utmost importance. Machine learning has been recently used in the detection of neuroradiological findings. Hence, it can be used in the detection of intracranial hematomas and furtherly initiate a management cascade of patient transfer, diagnostics, admission, and emergency intervention. We aim, here, to develop a diagnostic tool based on artificial intelligence to detect hematomas instantaneously, and automatically start a cascade of actions that support the management protocol depending on the early diagnosis.
Materials and Methods:A plot was designed as a staged model: The first stage of initiating and training the machine with the provisional evaluation of its accuracy and the second stage of supervised use in a tertiary care hospital and a third stage of its generalization in primary and secondary care hospitals. Two datasets were used: CQ500, a public dataset, and our dataset collected retrospectively from our tertiary hospital.
Results:A mean dice score of 0.83 was achieved on the validation set of CQ500. Moreover, the detection of intracranial hemorrhage was successful in 94% of cases for the CQ500 test set and 93% for our local institute cases. Poor detection was present in only 6-7% of the total test set. Moderate false-positive results were encountered in 18% and major false positives reached 5% for the total test set.
Conclusion:The proposed approach for the early detection of acute intracranial hematomas provides a reliable outset for generating an automatically initiated management cascade in high-flow hospitals.
更多查看译文
AI 理解论文
溯源树
样例
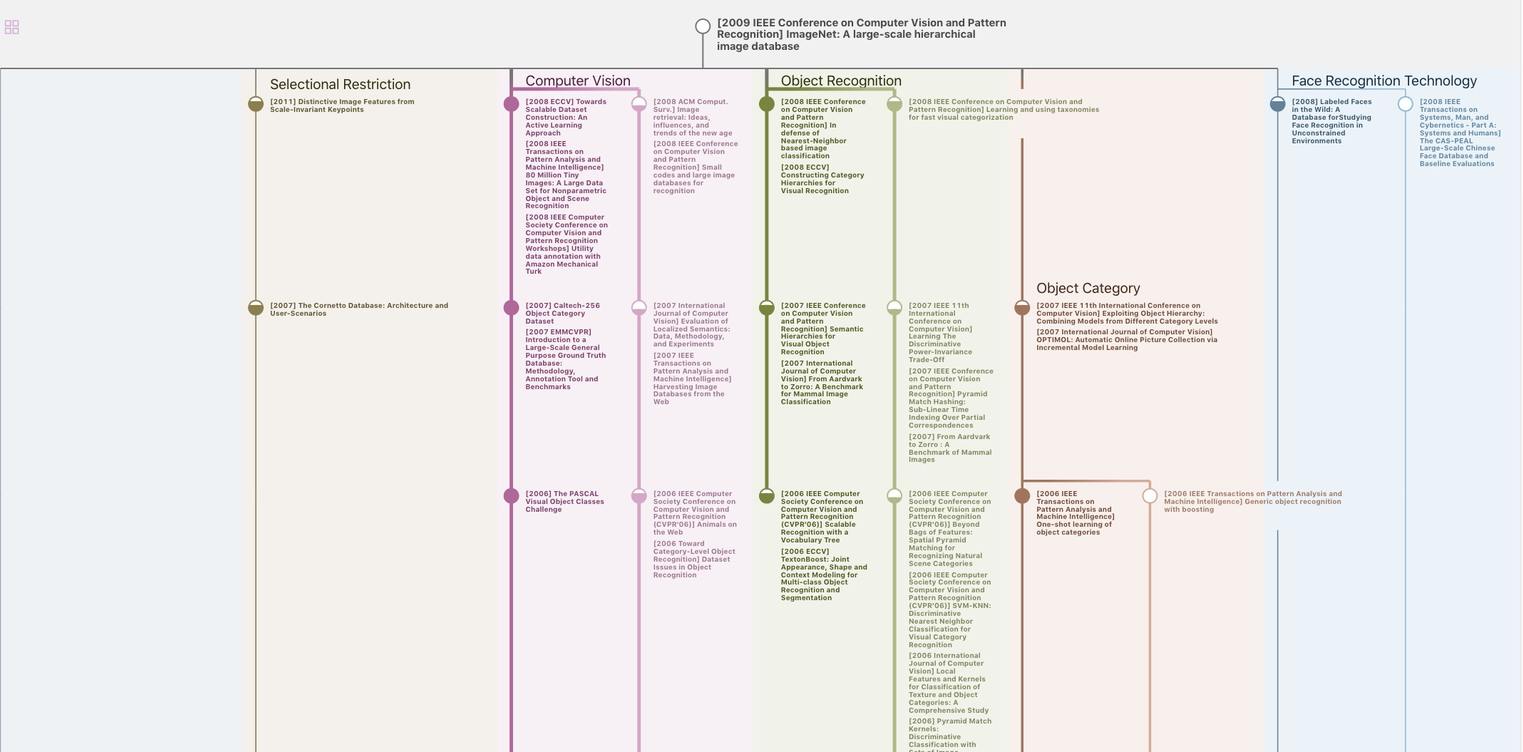
生成溯源树,研究论文发展脉络
Chat Paper
正在生成论文摘要