Boundary-Guided Lightweight Semantic Segmentation With Multi-Scale Semantic Context
IEEE Transactions on Multimedia(2024)
摘要
Lightweight semantic segmentation plays an essential role in image signal processing that is beneficial to many multimedia applications, such as self-driving, robotic vision, and virtual reality. Due to the powerful capability to encode image details and semantics, many lightweight dual-resolution networks have been proposed in recent years for semantic segmentation. In spite of achieving remarkable progresses, they often ignore semantic context ranged from different scales. Furthermore, most of them always neglect the object boundaries, serving as a significant assistance for lightweight semantic segmentation. To alleviate these problems, this paper develops a Boundary-guide dual-resolution lightweight network with multi-scale Semantic Context, called BSCNet, for semantic segmentation. Specifically, to enhance the capability of feature representation, an Extremely Lightweight Pyramid Pooling Module (ELPPM) is designed to capture multi-scale semantic context at the top of low-resolution branch of BSCNet. In addition, to increase feature similarity of the same object while keeping feature discrimination of different objects, pixel information is propagated throughout the entire object area using a simple Boundary Auxiliary Fusion Module (BAFM), where the predicted object boundaries are served as high-level guidance to refine low-level convolutional features. The comprehensive experimental results have demonstrated that our BSCNet is simple and effective, achieving state-of-the-art trade-off in terms of segmentation accuracy and running efficiency on CityScapes, CamVid, and KITTI datasets.
更多查看译文
关键词
lightweight semantic segmentation,boundary detection,feature propagation,convolutional neural networks
AI 理解论文
溯源树
样例
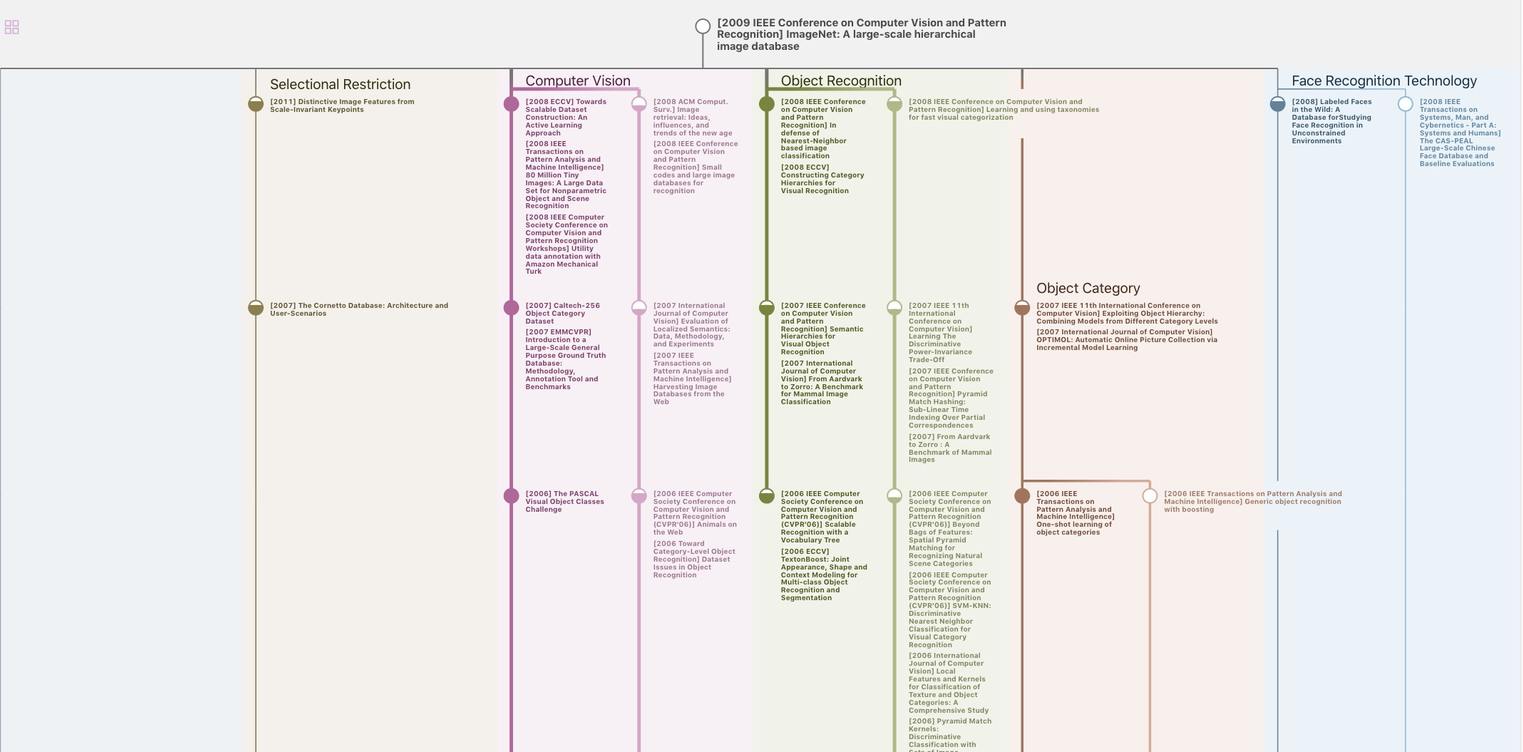
生成溯源树,研究论文发展脉络
Chat Paper
正在生成论文摘要