Retrieval of Leaf Area Index from MODIS Surface Reflectance by Incorporating the Sub-pixel Information from Decametric-resolution Data
IEEE Transactions on Geoscience and Remote Sensing(2024)
摘要
High-frequency leaf area index (LAI) dataset is essential for vegetation dynamic monitoring and crop yield estimation. However, due to the negative impacts of land surface heterogeneity, current hectometric-resolution LAI products cannot satisfy the uncertainty requirement of LAI dataset in practice. Here, we proposed a method named Utilization of Sub-Pixel Information (USPI) that leverages fine-scale remote sensing data to improve the accuracy of hectometric-resolution LAI retrieval. Specifically, based on machine learning (ML) models trained by representative samples, we retrieved the USPI LAI from MODIS reflectance by incorporating the sub-pixel information from Sentinel-2 LAI estimates. The USPI LAI was comprehensively evaluated using 30-m LAI reference maps in three aspects: the performance of different ML models, the comparison with MODIS LAI products, and the potential correction of USPI LAI for clumping effect. Results showed that Gaussian Process Regression (GPR) model outperformed other ML models for deriving LAI estimates. Furthermore, USPI LAI exhibited better performance than MODIS LAI product, with bias, root mean square error (RMSE), and R
2
of -0.308, 0.593, and 0.826, respectively, especially for pixels contaminated by atmospheric conditions. Nevertheless, the underestimation of USPI LAI should be noted because the effective LAI provided by Sentinel-2 was involved in the GPR training process. Thus, it is necessary to introduce the accurate clumping index dataset for further improvement of USPI LAI retrievals. Our study indicates that incorporating the sub-pixel information from decametric-resolution data can effectively reduce the uncertainty of hectometric-resolution LAI retrieval, which is promising for generating the high-accuracy LAI time series dataset.
更多查看译文
关键词
leaf area index,spatial heterogeneity,sub-pixel information,multi-scale data,MODIS
AI 理解论文
溯源树
样例
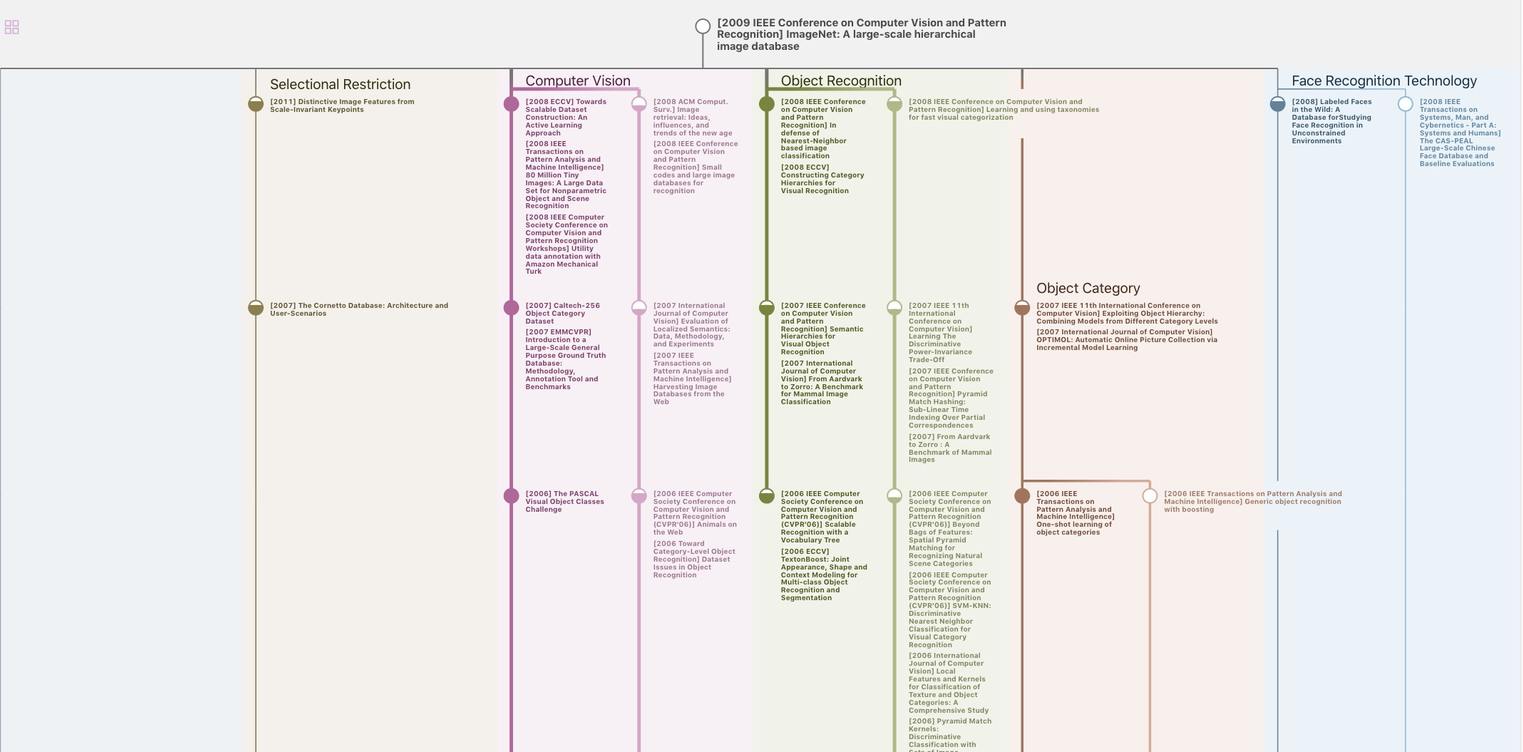
生成溯源树,研究论文发展脉络
Chat Paper
正在生成论文摘要