FR-nnUNet: A Novel Medical Segmentation Network Based on the Fuzzy Regions Recognition Scheme and Improved nnU-Net
crossref(2024)
摘要
Abstract The performance of the traditional supervised learning based medical image segmentation methods heavily depends on the expensive and scarce data labeling. The semi-supervised based segmentation strategies, such as the mean teacher model, have achieved milestone success in this domain. However, few studies have focused on the problem of target selection. Therefore, a novel medical image segmentation model is proposed based on the fuzzy regions recognition scheme and improved nnU-Net. The novel fuzzy regions recognition strategy is introduced and the improved nnU-Net with the new semi-supervised mean teacher network architecture is constructed, which can efficiently optimize the representation of image features via the intensity and spatial transformations. The class-conditional labeling noise identification method is applied to the image segmentation task and combines the concepts of confusion matrix and thresholding to improve the robustness of the model to class imbalance and overconfidence predictions. Experimental results show that the proposed fuzzy selective mean teacher model can achieve excellent segmentation performance on the Left Atrium and ACDC datasets. Compared with the traditional methods, it can not only improve the objective segmentation evaluation metrics in terms of the Dice coefficient, Jaccard coefficient, and Hausdorff95 distance but also obtain better segmentation results especially when less labeled data is used.
更多查看译文
AI 理解论文
溯源树
样例
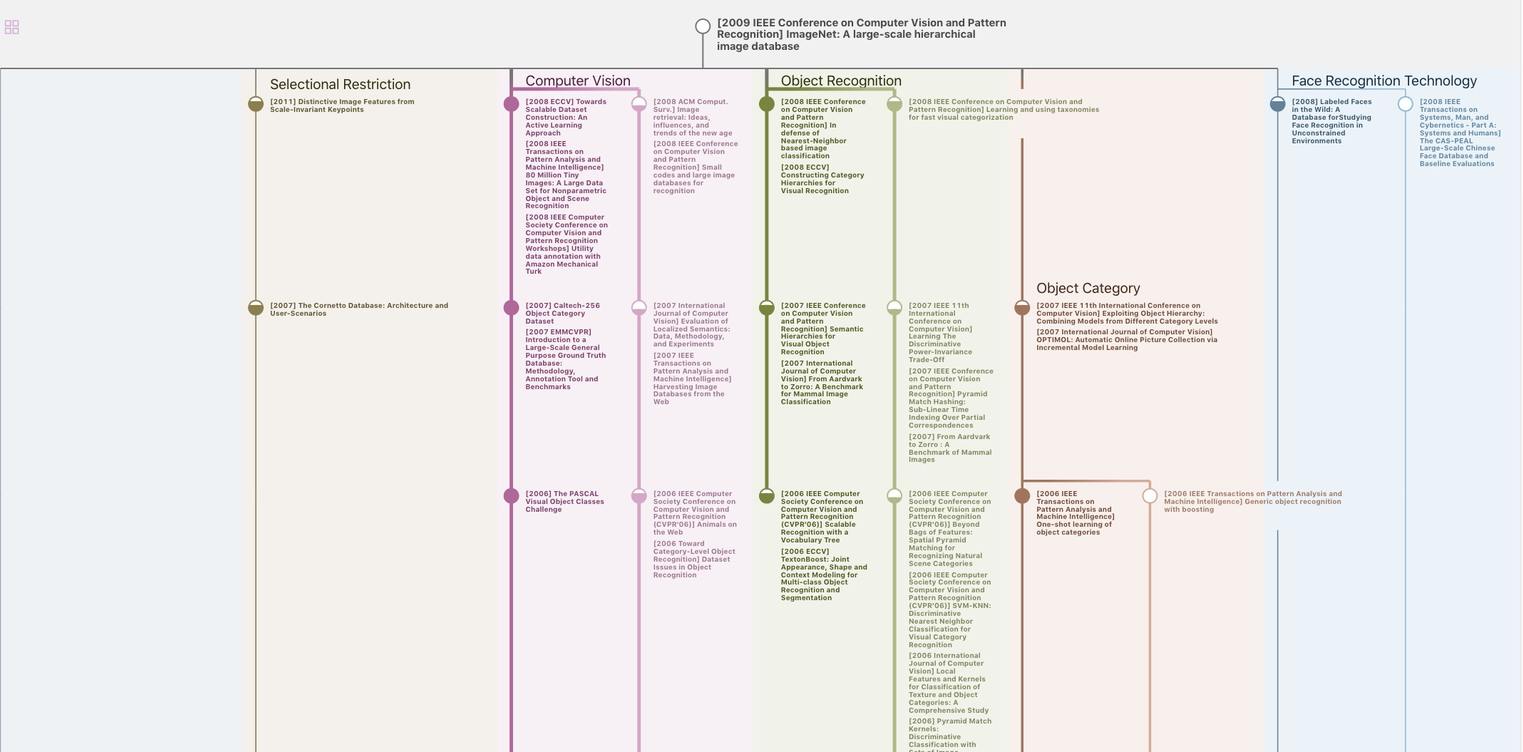
生成溯源树,研究论文发展脉络
Chat Paper
正在生成论文摘要