Surface-SOS: Self-Supervised Object Segmentation via Neural Surface Representation.
IEEE transactions on image processing : a publication of the IEEE Signal Processing Society(2024)
摘要
Self-supervised Object Segmentation (SOS) aims to segment objects without any annotations. Under conditions of multi-camera inputs, the structural, textural and geometrical consistency among each view can be leveraged to achieve fine-grained object segmentation. To make better use of the above information, we propose Surface representation based Self-supervised Object Segmentation (Surface-SOS), a new framework to segment objects for each view by 3D surface representation from multi-view images of a scene. To model high-quality geometry surfaces for complex scenes, we design a novel scene representation scheme, which decomposes the scene into two complementary neural representation modules respectively with a Signed Distance Function (SDF). Moreover, Surface-SOS is able to refine single-view segmentation with multi-view unlabeled images, by introducing coarse segmentation masks as additional input. To the best of our knowledge, Surface-SOS is the first self-supervised approach that leverages neural surface representation to break the dependence on large amounts of annotated data and strong constraints. These constraints typically involve observing target objects against a static background or relying on temporal supervision in videos. Extensive experiments on standard benchmarks including LLFF, CO3D, BlendedMVS, TUM and several real-world scenes show that Surface-SOS always yields finer object masks than its NeRF-based counterparts and surpasses supervised single-view baselines remarkably. Code is available at: https://github.com/zhengxyun/Surface-SOS.
更多查看译文
关键词
Self-supervised learning,neural surface representation,multi-view object segmentation
AI 理解论文
溯源树
样例
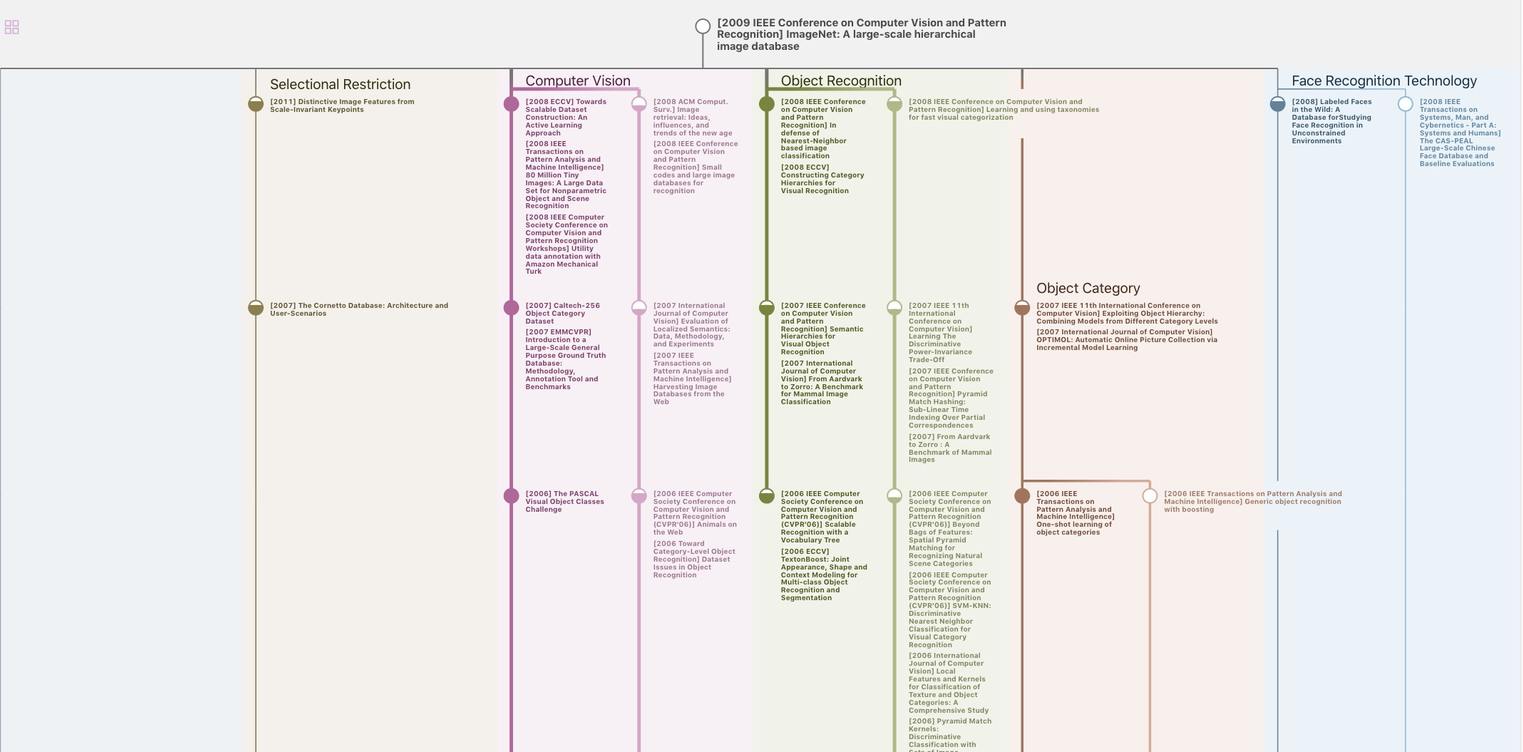
生成溯源树,研究论文发展脉络
Chat Paper
正在生成论文摘要