GELF: A global error-based learning function for globally optimal adaptive reliability analysis
Structural Safety(2024)
摘要
Kriging has gained significant attention for reliability analysis primarily because of the analytical form of its uncertainty information, which facilitates adaptive training and establishing stopping criteria for the training process. Learning functions play a significant role in both selection of training points and stoppage of the training. For these functions, most existing learning functions evaluate candidate training points individually. However, lack of consideration for the global effects can lead to suboptimal training. In addition, the subjectivity of these stopping criteria may result in over or undertraining of surrogate models. To overcome these gaps, we propose Global Error-based Learning Function (GELF) for optimal refinement of Kriging surrogate models for the specific purpose of reliability analysis. Instead of prioritizing training points based on their uncertainty and proximity to the limit state like the existing learning functions, GELF for the first time directly and analytically associates the maximum error in the failure probability estimate to the global effect of choosing a candidate training point. This development subsequently facilitates an adaptive training scheme that minimizes the error in adaptive reliability estimation to the highest degree. For this purpose, GELF uses hypothetical future uncertainty information by treating the current construction of the surrogate model as a generative model. The proposed method is tested on three classic benchmark problems and one practical engineering problem. Results indicate that the proposed method has significantly better computational efficiency than the state-of-the-art methods while achieving high accuracy in all the numerical examples.
更多查看译文
关键词
Reliability analysis,Surrogate modeling,Adaptive Kriging,Gaussian process,Global error-based learning function,Error-based stopping criterion
AI 理解论文
溯源树
样例
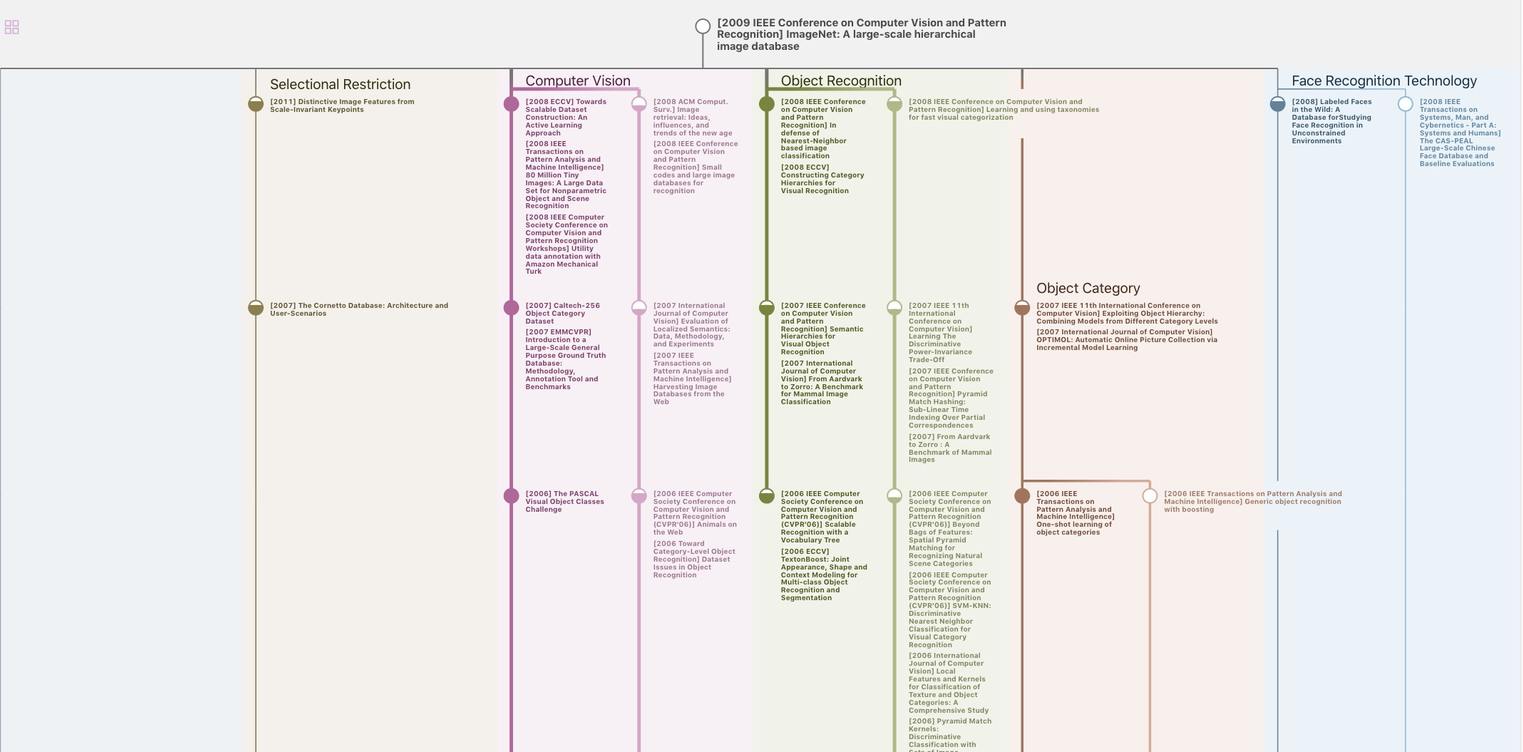
生成溯源树,研究论文发展脉络
Chat Paper
正在生成论文摘要