Approximately Optimal Domain Adaptation with Fisher’s Linear Discriminant
Mathematics(2024)
摘要
We propose and study a data-driven method that can interpolate between a classical and a modern approach to classification for a class of linear models. The class is the convex combinations of an average of the source task classifiers and a classifier trained on the limited data available for the target task. We derive the expected loss of an element in the class with respect to the target distribution for a specific generative model, propose a computable approximation of the loss, and demonstrate that the element of the proposed class that minimizes the approximated risk is able to exploit a natural bias–variance trade-off in task space in both simulated and real-data settings. We conclude by discussing further applications, limitations, and potential future research directions.
更多查看译文
关键词
statistical learning,domain adaptation,transfer learning,physiological prediction,linear classifiers
AI 理解论文
溯源树
样例
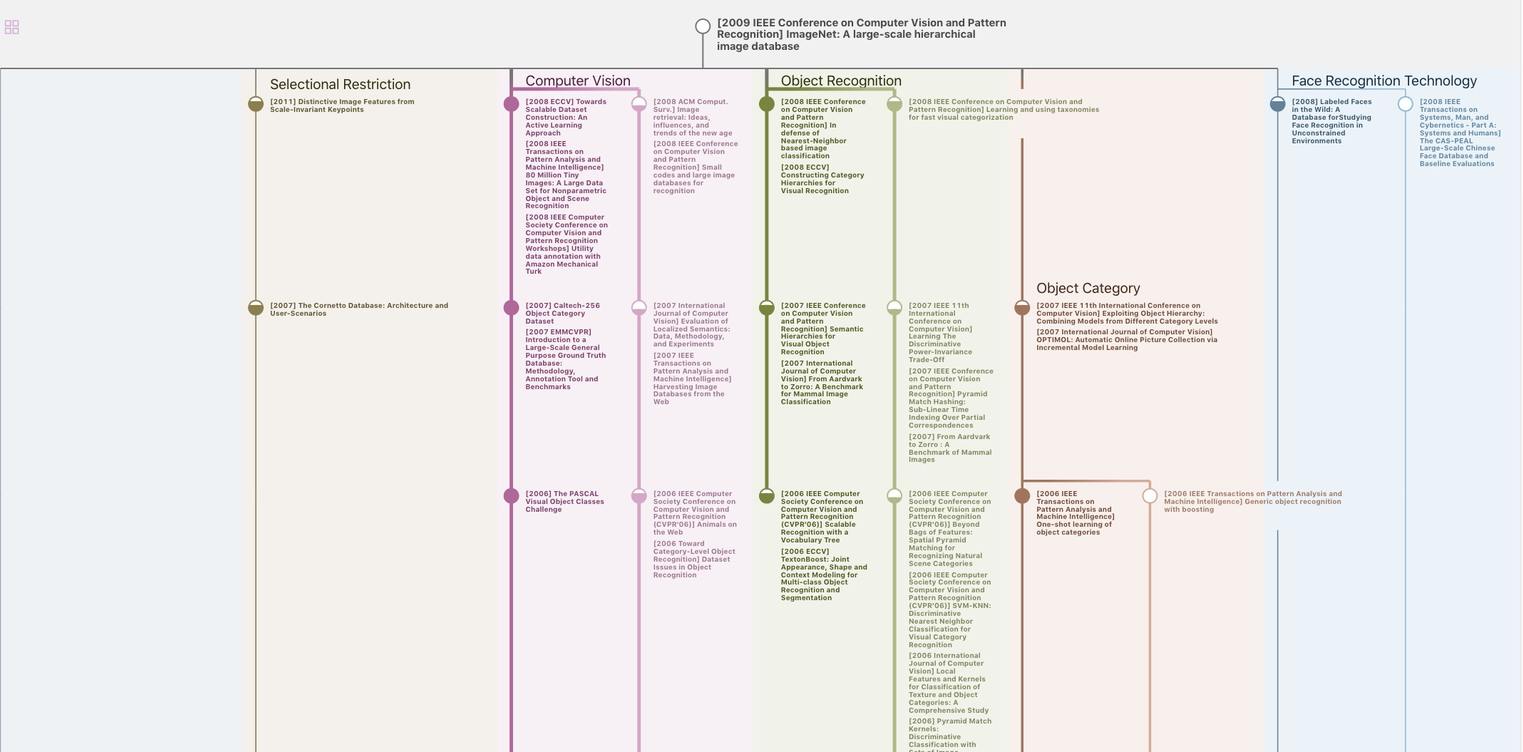
生成溯源树,研究论文发展脉络
Chat Paper
正在生成论文摘要