Soft Contrastive Cross-Modal Retrieval
APPLIED SCIENCES-BASEL(2024)
摘要
Cross-modal retrieval plays a key role in the Natural Language Processing area, which aims to retrieve one modality to another efficiently. Despite the notable achievements of existing cross-modal retrieval methodologies, the complexity of the embedding space increases with more complex models, leading to less interpretable and potentially overfitting representations. Most existing methods realize outstanding results based on datasets without any error or noise, but that is extremely ideal and leads to trained models lacking robustness. To solve these problems, in this paper, we propose a novel approach, Soft Contrastive Cross-Modal Retrieval (SCCMR), which integrates the deep cross-modal model with soft contrastive learning and smooth label cross-entropy learning to boost common subspace embedding and improve the generalizability and robustness of the model. To confirm the performance and effectiveness of SCCMR, we conduct extensive experiments comparing 12 state-of-the-art methods on three multi-modal datasets by using image-text retrieval as a showcase. The experimental results show that our proposed method outperforms the baselines.
更多查看译文
关键词
cross-modal retrieval,soft contrastive learning,smooth label learning,common subspace,deep learning
AI 理解论文
溯源树
样例
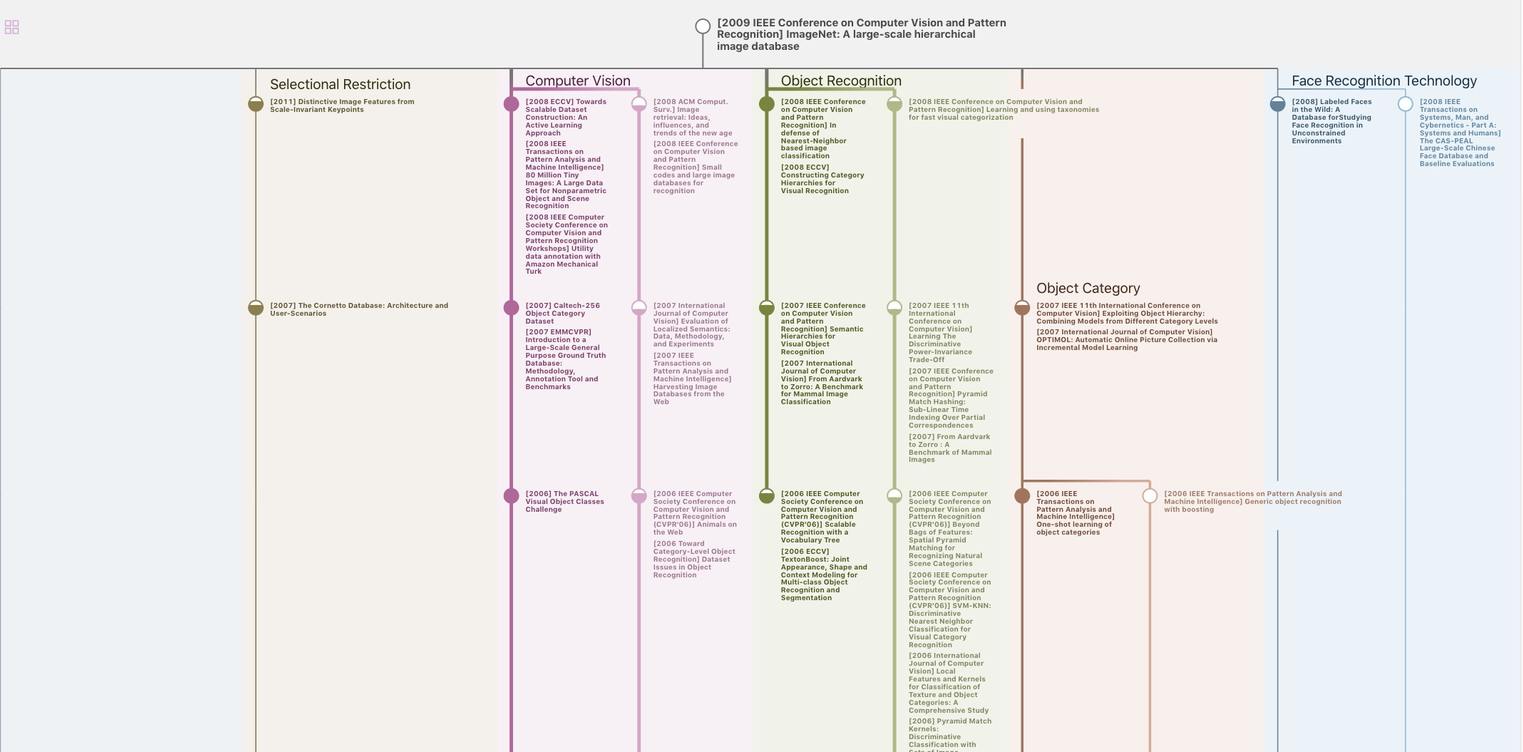
生成溯源树,研究论文发展脉络
Chat Paper
正在生成论文摘要