Measurement and sampling noise undermine inferences about awareness in location probability learning: A modeling approach
crossref(2024)
摘要
Occasionally, experimental psychologists enter into the realm of psychometrics without being fully aware of the risks involved in the study of individual differences. Here we re-assess the many studies on location probability learning that suggest that people can unconsciously learn to suppress salient but irrelevant distractors frequently presented in a certain location. In the additional singleton task, one of the arguments to support this claim is that suppression in search times does not significantly differ between “aware” and “unaware” participants. Although rarely acknowledged, this null interaction could also result if the data are contaminated by measurement and/or sampling noise. Unfortunately, the reliability of the awareness measure cannot be assessed with standard methods, since it is a single-trial test. In the present study we offer model-based estimations of measurement and sampling noise in empirical data. Our goal is to determine how often researchers would mistakenly conclude that learning is unconscious, given data from a model based on the opposite claim (i.e., that learning is conscious) but including noise in participants’ search times and awareness responses. To do so, we fitted this noisy conscious model to a public dataset involving 159 participants who performed the additional singleton task. Estimated parameters from this model were used, first, to predict the observed pattern of results and, second, to simulate new responses and participants. Results suggest that, under reasonable measurement noise and sample sizes, simulated evidence from the model can paradoxically but falsely support arguments used to defend the unconscious learning hypothesis. This study serves as an illustration to experimental psychologists of the risks of neglecting basic psychometric requirements in individual differences research.
更多查看译文
AI 理解论文
溯源树
样例
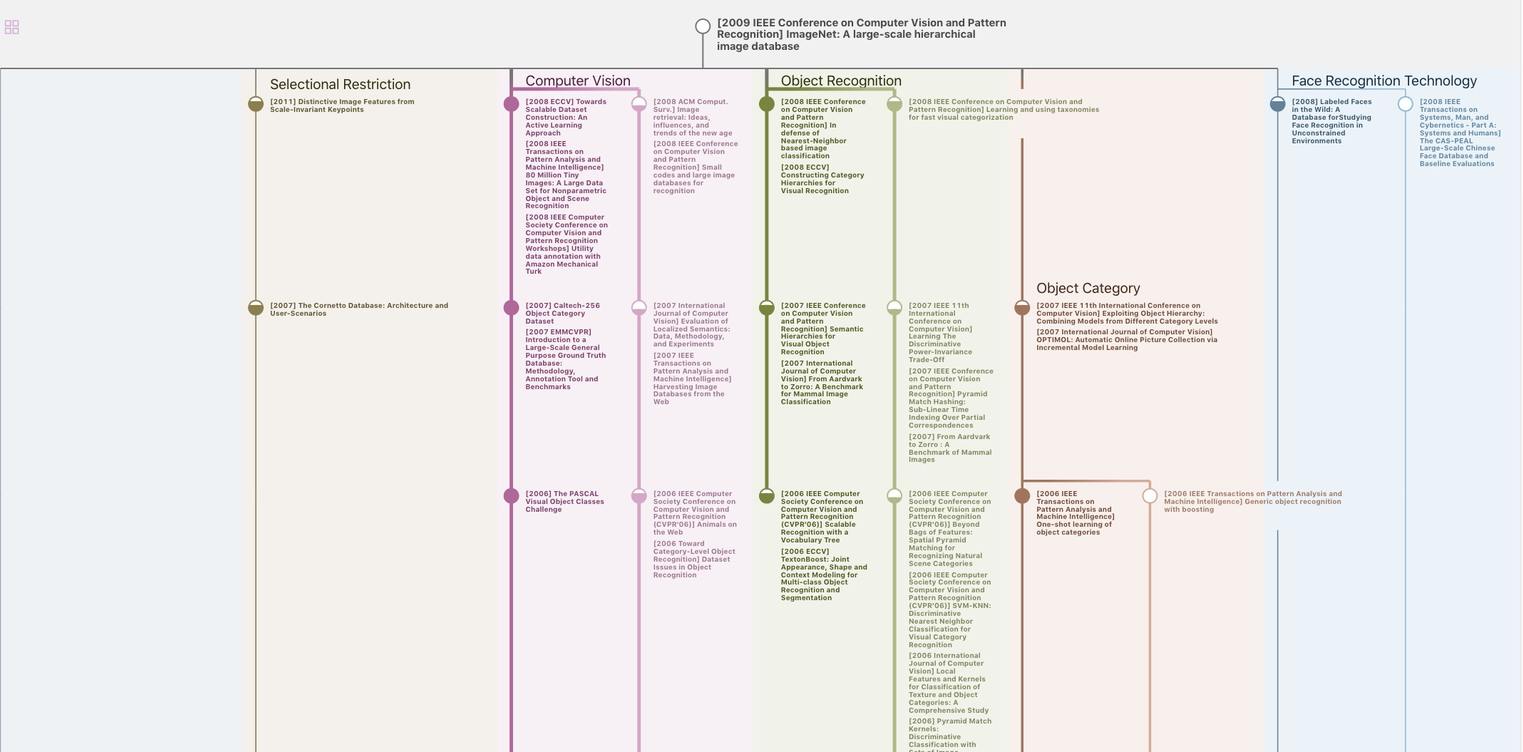
生成溯源树,研究论文发展脉络
Chat Paper
正在生成论文摘要