SCRL: Self-supervised Continual Reinforcement Learning for Domain Adaptation
2023 International Conference on Artificial Intelligence of Things and Systems (AIoTSys)(2023)
摘要
Deep reinforcement learning outperforms humans in many tasks. However, in reality, the scenarios of agent deployment often change, and the performance of models may therefore degrade, resulting in unreasonable decisions in new scenarios. Therefore, it is important for the model to own the evolution ability in new scenarios. Although recent attempts have studied the continual training of models through reward signals or self-supervised learning, on the one hand, previously used reward signals in the target domain are generally difficult to obtain and on the other hand, the performance of self-supervised evolution is always limited. In addition, adapting to the target domain usually results in forgetting the source domain. To overcome the above problems, in this paper, we propose a self-supervised continual reinforcement learning method, called SCRL, which combines reinforcement learning tasks with self-supervised learning auxiliary tasks and weight regularizes. Specifically, in the source domain, we first jointly train different objectives of reinforcement and self-supervised learning, which enables to share the same encoder. The Fisher information matrix is then calculated to record the importance of the model parameters to the source domain. In the target domain, the encoder is used for the reinforcement learning task and is updated by self-supervised learning under the control of the Fisher regularizer. Our extensive experiments on four continuous control tasks from the DeepMind Control suite showed that in the absence of reward signals, the proposed SCRL can effectively adapt the model to the target domain without catastrophically forgetting the source domain.
更多查看译文
关键词
domain adaptation,reinforcement learning,self-supervised learning,continual learning,Fisher regularizer
AI 理解论文
溯源树
样例
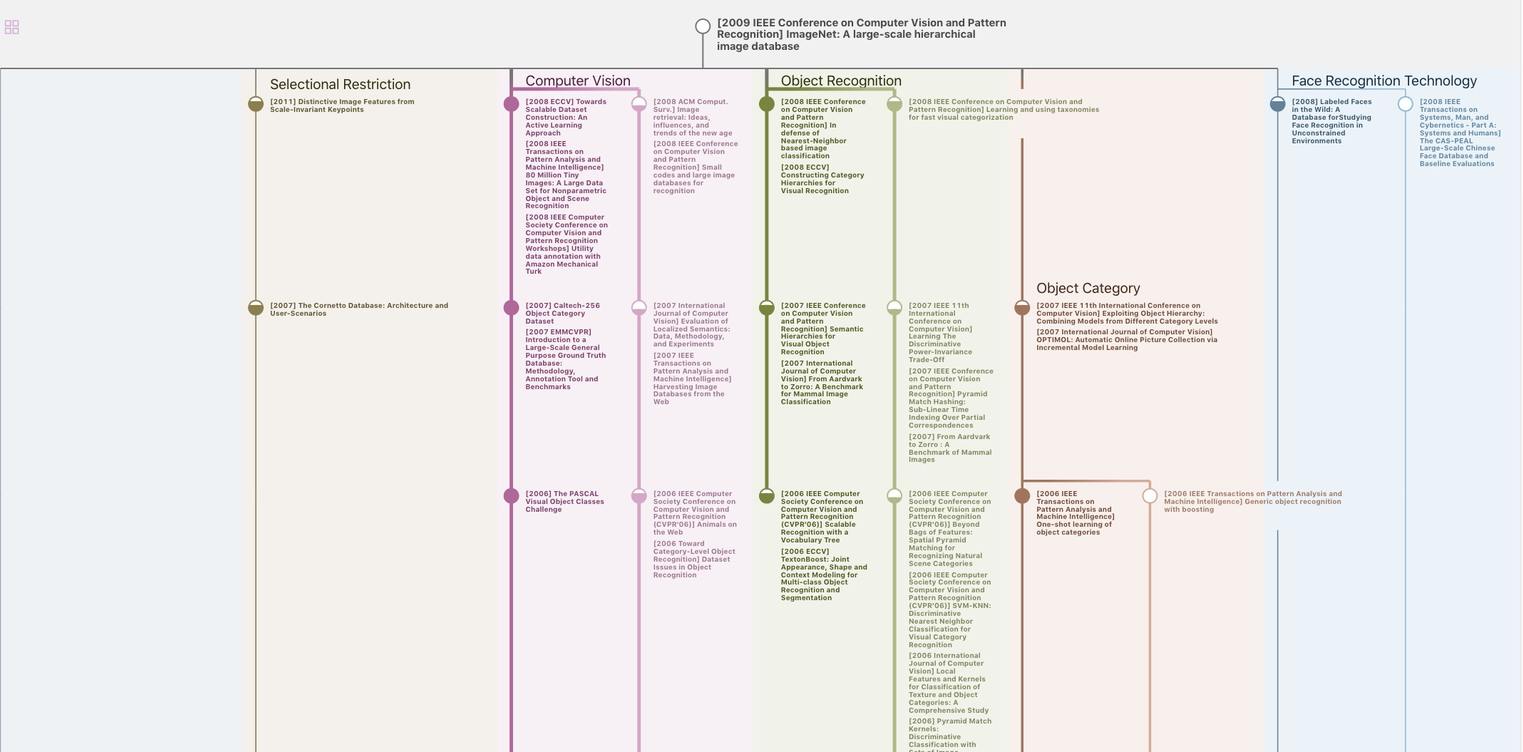
生成溯源树,研究论文发展脉络
Chat Paper
正在生成论文摘要