Stability and Accuracy-Aware Learning for Task Offloading in UAV-MEC-Assisted Smart Farms
IEEE Transactions on Network and Service Management(2024)
摘要
Sentiment Analysis Systems (SASs) are data-driven Artificial Intelligence (AI) systems that assign one or more numbers to convey the polarity and emotional intensity of a given piece of text. However, like other automatic machine learning systems, SASs can exhibit model uncertainty, resulting in drastic swings in output with even small changes in input. This issue becomes more problematic when inputs involve protected attributes like gender or race, as it can be perceived as bias or unfairness. To address this, we propose a novel method to assess and rate SASs. We perturb inputs in a controlled causal setting to test if the output sentiment is sensitive to protected attributes while keeping other components of the textual input, such as chosen emotion words, fixed. Based on the results, we assign labels (ratings) at both fine-grained and overall levels to indicate the robustness of the SAS to input changes. The ratings can help decision-makers improve online content by reducing hate speech, often fueled by biases related to protected attributes such as gender and race. These ratings provide a principled basis for comparing SASs and making informed choices based on their behavior. The ratings also benefit all users, especially developers who reuse off-the-shelf SASs to build larger AI systems but do not have access to their code or training data to compare.
更多查看译文
关键词
AI,Bias,Causality,Rating,Sentiment Analysis Systems,User Trust
AI 理解论文
溯源树
样例
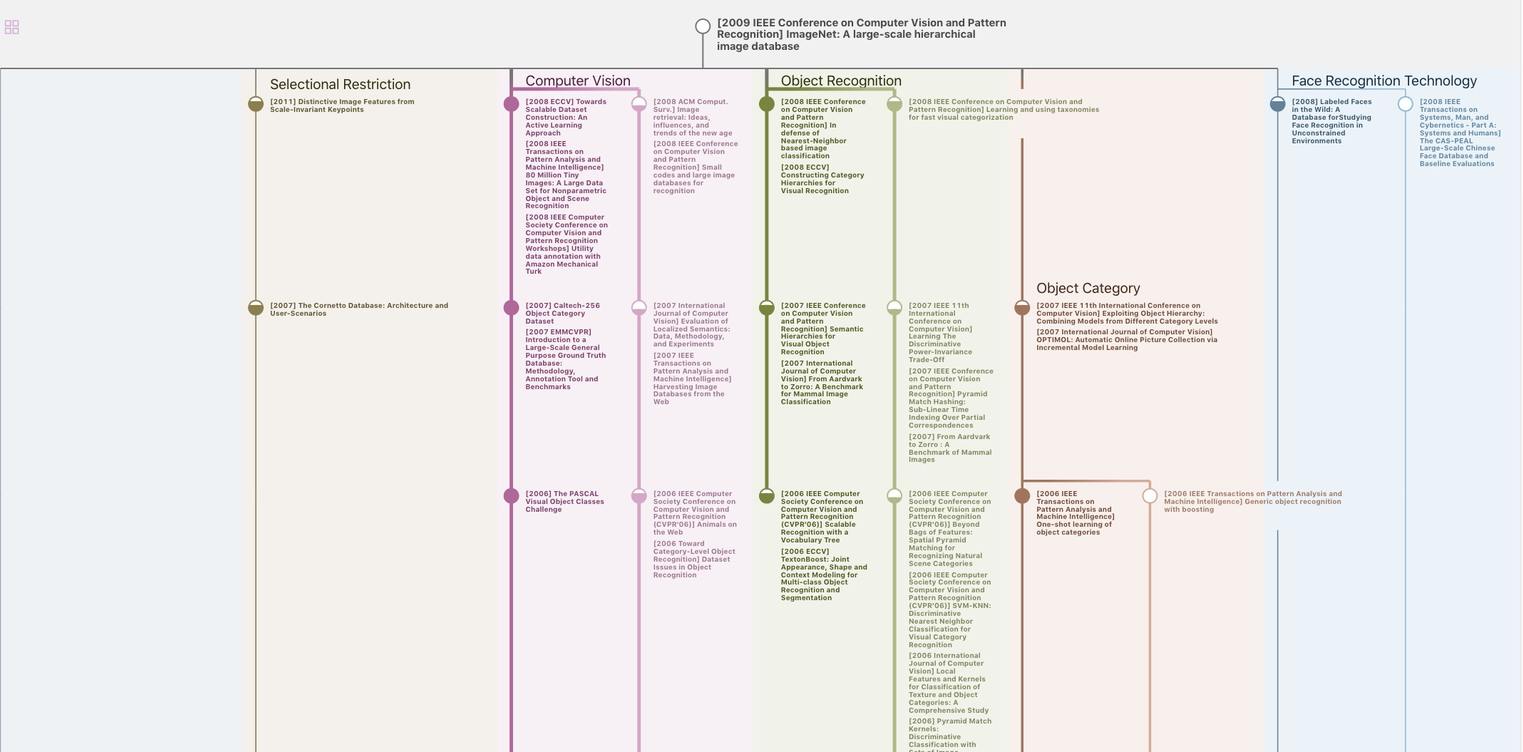
生成溯源树,研究论文发展脉络
Chat Paper
正在生成论文摘要