Progressive deep snake for instance boundary extraction in medical images
Expert Systems with Applications(2024)
摘要
Boundary extraction is meaningful in medical image analysis since it explicitly extracts the tissue/lesions boundary coordinates, which benefits the follow-up diagnosis processes. However, accurately extracting human tissue/lesions is challenging due to unclear target boundaries or pseudo boundaries, which could lead to inferior performance of existing boundary extraction and/or semantic segmentation methods. We propose a Progressive Deep Snake (PDS), which is designed with a multi-step contour evolution (MCE) and a sequential label generation module (SLGM) to explicitly extract the smooth and continuous boundary of different tissue/lesions even if they are unclear, with irregular shape, or occluded by pseudo boundaries. (1) MCE is designed to initialize and evolve the contours to the target boundaries, where a multi-step deep supervision strategy is designed to improve flexibility and accuracy. (2) SLGM is designed to generate easy-to-difficult supervision signals for the contour evolution, which provides targets of moderate difficulty in each step for better guiding the contour evolution. Experiments on a challenging verbal segment datasets show that our method achieves state-of-the-art performance (MR_AVBCE dataset: mIoU: 0.9234, mDice: 0.9598, mBoundF: 0.9241, mWcov: 0.9704). Our code is available at https://github.com/sysu19351118/PDS-pytorch.
更多查看译文
关键词
Boundary extraction,Deep learning,Vertebral segmentation
AI 理解论文
溯源树
样例
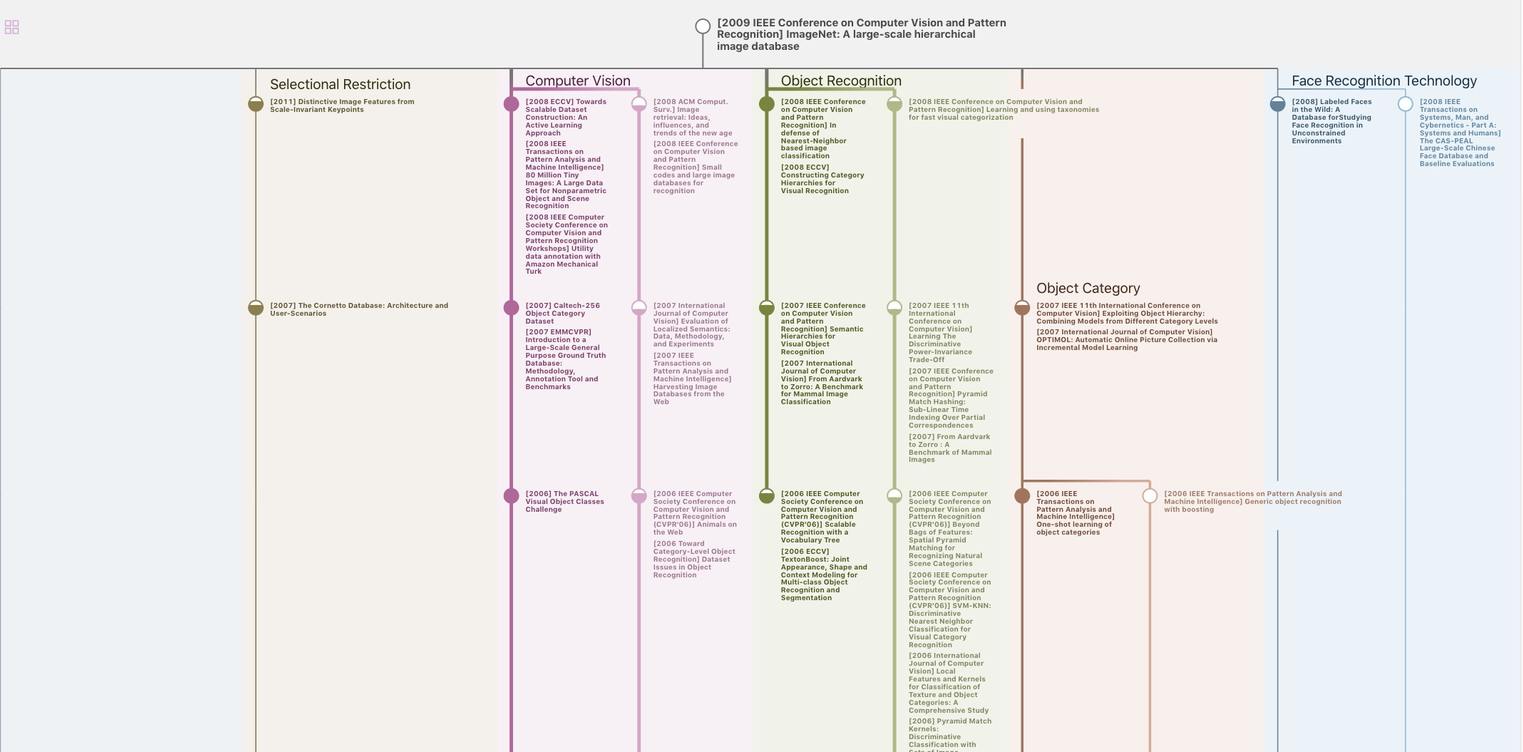
生成溯源树,研究论文发展脉络
Chat Paper
正在生成论文摘要