Enhancing variational Monte Carlo simulations using a programmable quantum simulator
PHYSICAL REVIEW A(2024)
摘要
Programmable quantum simulators based on Rydberg atom arrays are a fast-emerging quantum platform, bringing together long coherence times, high-fidelity operations, and large numbers of interacting qubits deterministically arranged in flexible geometries. Today's Rydberg array devices are demonstrating their utility as quantum simulators for studying phases and phase transitions in quantum matter. In this paper, we show that unprocessed and imperfect experimental projective measurement data can be used to enhance in silico simulations of quantum matter, by improving the performance of variational Monte Carlo simulations. As an example, we focus on data spanning the disordered-to-checkerboard transition in a 16 x 16 square-lattice array [S. Ebadi et al., Nature (London) 595, 227 (2021)] and employ the data-enhanced variational Monte Carlo algorithm to train powerful autoregressive wave-function ansatze based on recurrent neural networks (RNNs). We observe universal improvements in the convergence times of our simulations with this hybrid training scheme. Notably, we also find that pretraining with experimental data enables relatively simple RNN ansatze to accurately capture phases of matter that are not learned with a purely variational training approach. Our work highlights the promise of hybrid quantum-classical approaches for large-scale simulation of quantum many-body systems, combining autoregressive language models with experimental data from existing quantum devices.
更多查看译文
AI 理解论文
溯源树
样例
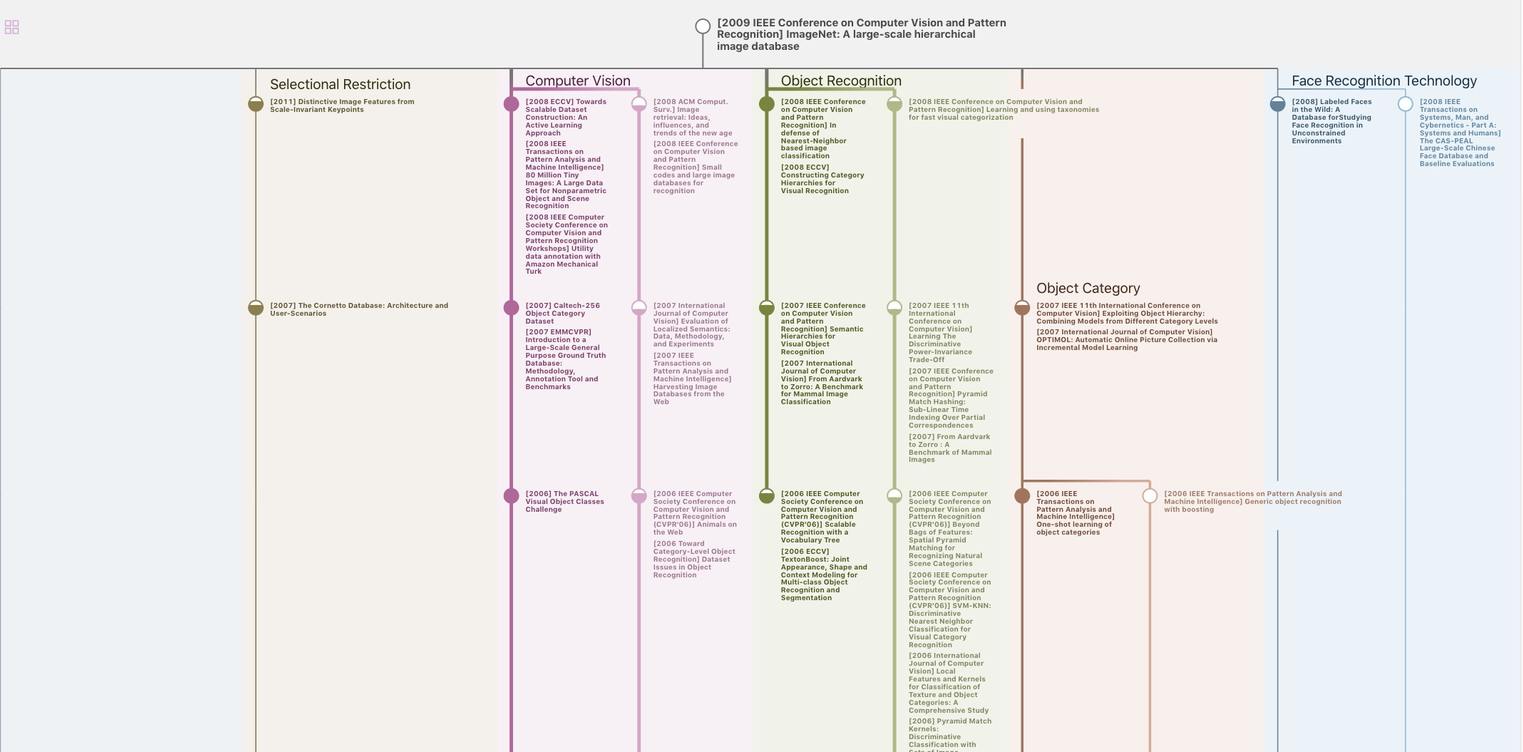
生成溯源树,研究论文发展脉络
Chat Paper
正在生成论文摘要