Tactical Decision Making for Autonomous Trucks by Deep Reinforcement Learning with Total Cost of Operation Based Reward
CoRR(2024)
摘要
We develop a deep reinforcement learning framework for tactical decision
making in an autonomous truck, specifically for Adaptive Cruise Control (ACC)
and lane change maneuvers in a highway scenario. Our results demonstrate that
it is beneficial to separate high-level decision-making processes and low-level
control actions between the reinforcement learning agent and the low-level
controllers based on physical models. In the following, we study optimizing the
performance with a realistic and multi-objective reward function based on Total
Cost of Operation (TCOP) of the truck using different approaches; by adding
weights to reward components, by normalizing the reward components and by using
curriculum learning techniques.
更多查看译文
AI 理解论文
溯源树
样例
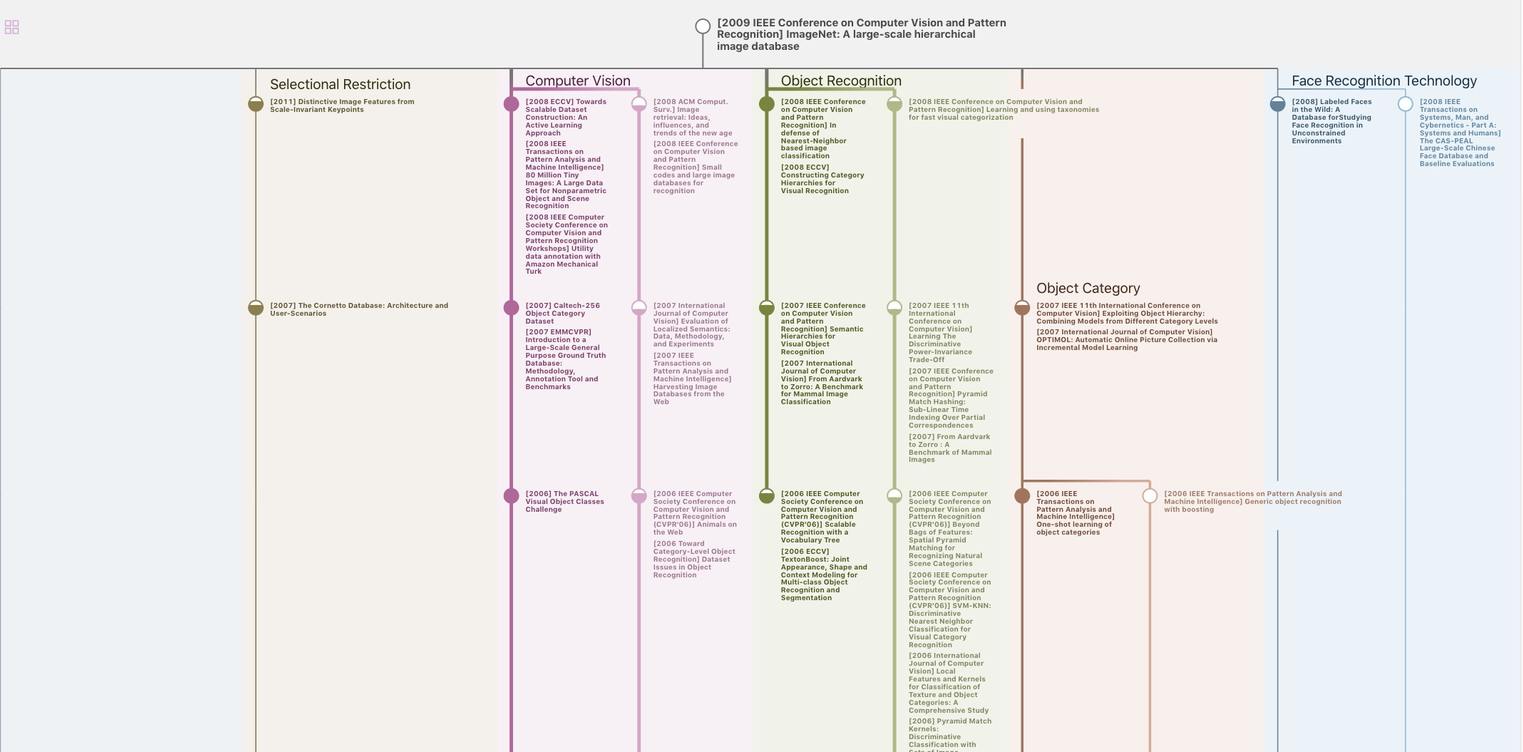
生成溯源树,研究论文发展脉络
Chat Paper
正在生成论文摘要