Applications of model-aware reinforcement learning in Bayesian quantum metrology
arxiv(2024)
摘要
An important practical problem in the field of quantum metrology and sensors
is to find the optimal sequences of controls for the quantum probe that realize
optimal adaptive estimation. In Belliardo et al., arXiv:2312.16985 (2023), we
solved this problem in general, by introducing a procedure capable of
optimizing a wide range of tasks in quantum metrology and estimation by
combining model-aware reinforcement learning with Bayesian inference. We take a
model-based approach to the optimisation where the physics describing the
system is explicitly taken into account in the training through automatic
differentiation. In this follow-up paper we present some applications of the
framework. The first family of examples concerns the estimation of magnetic
fields, hyperfine interactions, and decoherence times for electronic spins in
diamond. For these examples, we perform multiple Ramsey measurements on the
spin. The second family of applications concerns the estimation of phases and
coherent states on photonic circuits, without squeezing elements, where the
bosonic lines are measured by photon counters. This exposition showcases the
broad applicability of the method, which has been implemented in the qsensoropt
library released on PyPI, which can be installed with pip.
更多查看译文
AI 理解论文
溯源树
样例
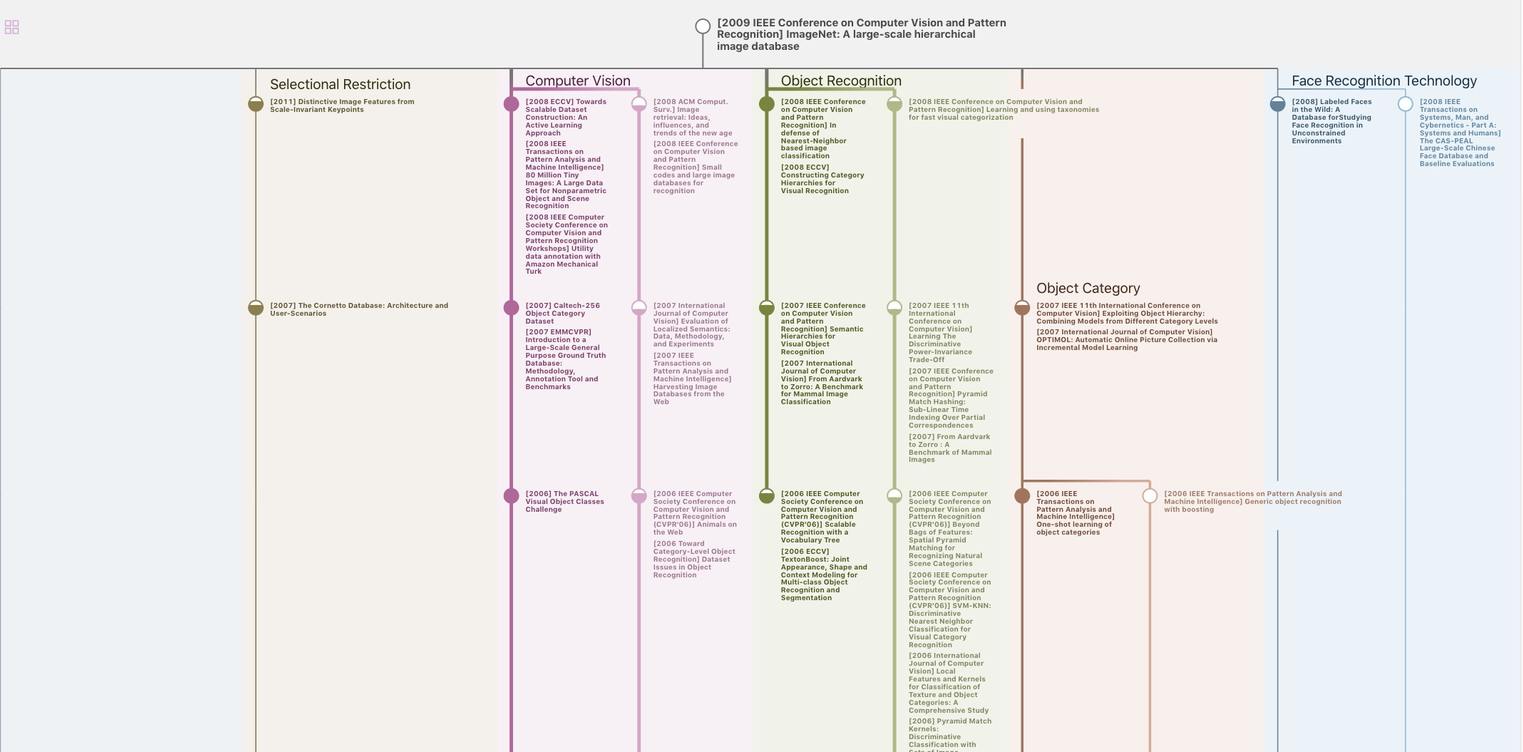
生成溯源树,研究论文发展脉络
Chat Paper
正在生成论文摘要