A feature-based framework to investigate atmospheric predictability.
crossref(2024)
摘要
Atmospheric predictability is intrinsically limited by the upscale growth of initial small-scale, small-amplitude errors. For practical predictability, model error and initial-condition uncertainty also contribute significantly. The accurate representation and interactions of these factors within numerical weather prediction systems determine the extent to which forecast uncertainty is correctly modeled. An improved understanding of upscale error-growth mechanisms and their flow dependence in numerical weather prediction models has several implications: it enables more focused model verification and development, aids in recognizing limitations in emerging forecasts systems like machine-learning-based approaches, and may indicate when the intrinsic limit of predictability has been reached. Studying the flow dependence of error growth requires a local perspective, which is not provided by the traditional spectral perspective on upscale error growth. We here take a complementary approach and apply a feature-based perspective. We have developed an automated algorithm to identify error features in gridded data and track their spatial and temporal evolution. Errors are considered in terms of potential vorticity (PV) and near the tropopause, where they maximize. A previously derived PV-error tendency equation is evaluated to quantify the different contributions to error-growth experiments with the global prediction Model ICON from the German Weather Service. Errors in these experiments grow from differences in the seeding of a stochastic convection scheme. In a suite of experiments, this source of uncertainty competes with initial-condition uncertainty of varying magnitude. Evaluation of the process-specific error-growth rates allow the detailed quantification of the upscale-growth mechanisms. For this purpose, we integrate the growth rates over the respective area associated with an error feature. Examination of the combined growth rates of all features in an upscale-error-growth experiment reproduces a previously found three-stage multi-scale upscale-growth paradigm. Illustration the importance of flow dependence, the growth rates from a single feature can substantially differ from the overall average. Further highlighting this importance, intrinsic limits of predictivity can be identified for some features even in the presence of substantial initial-condition uncertainty. The presentation will conclude with a comparison of error evolution in conventional numerical weather prediction systems to a data-driven, machine-learned model.
更多查看译文
AI 理解论文
溯源树
样例
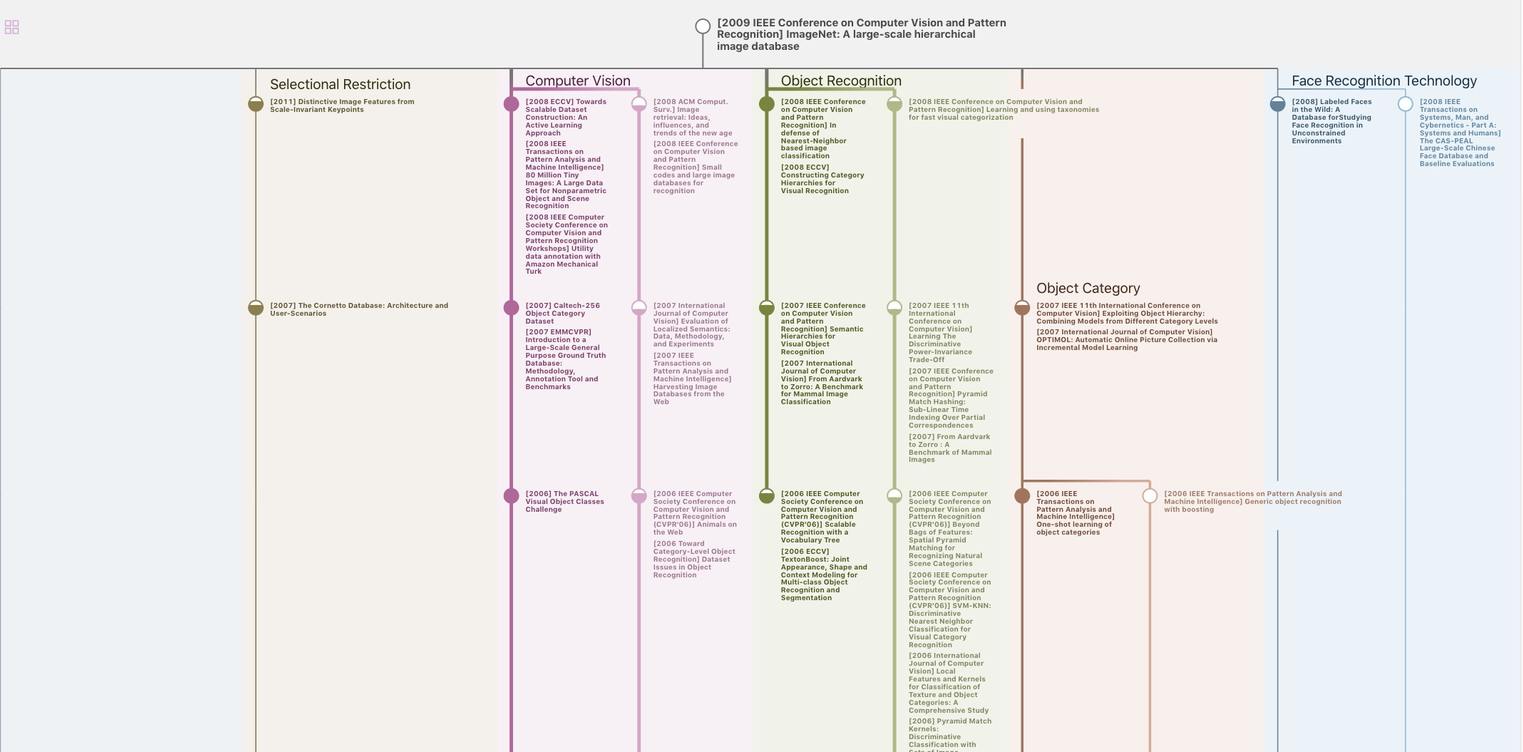
生成溯源树,研究论文发展脉络
Chat Paper
正在生成论文摘要