Bias-Augmented Consistency Training Reduces Biased Reasoning in Chain-of-Thought
arxiv(2024)
摘要
While chain-of-thought prompting (CoT) has the potential to improve the
explainability of language model reasoning, it can systematically misrepresent
the factors influencing models' behavior–for example, rationalizing answers in
line with a user's opinion without mentioning this bias. To mitigate this
biased reasoning problem, we introduce bias-augmented consistency training
(BCT), an unsupervised fine-tuning scheme that trains models to give consistent
reasoning across prompts with and without biasing features. We construct a
suite testing nine forms of biased reasoning on seven question-answering tasks,
and find that applying BCT to GPT-3.5-Turbo with one bias reduces the rate of
biased reasoning by 86
other forms of bias, reducing biased reasoning on held-out biases by an average
of 37
this method may hold promise for reducing biased reasoning from as-of-yet
unknown biases and on tasks where supervision for ground truth reasoning is
unavailable.
更多查看译文
AI 理解论文
溯源树
样例
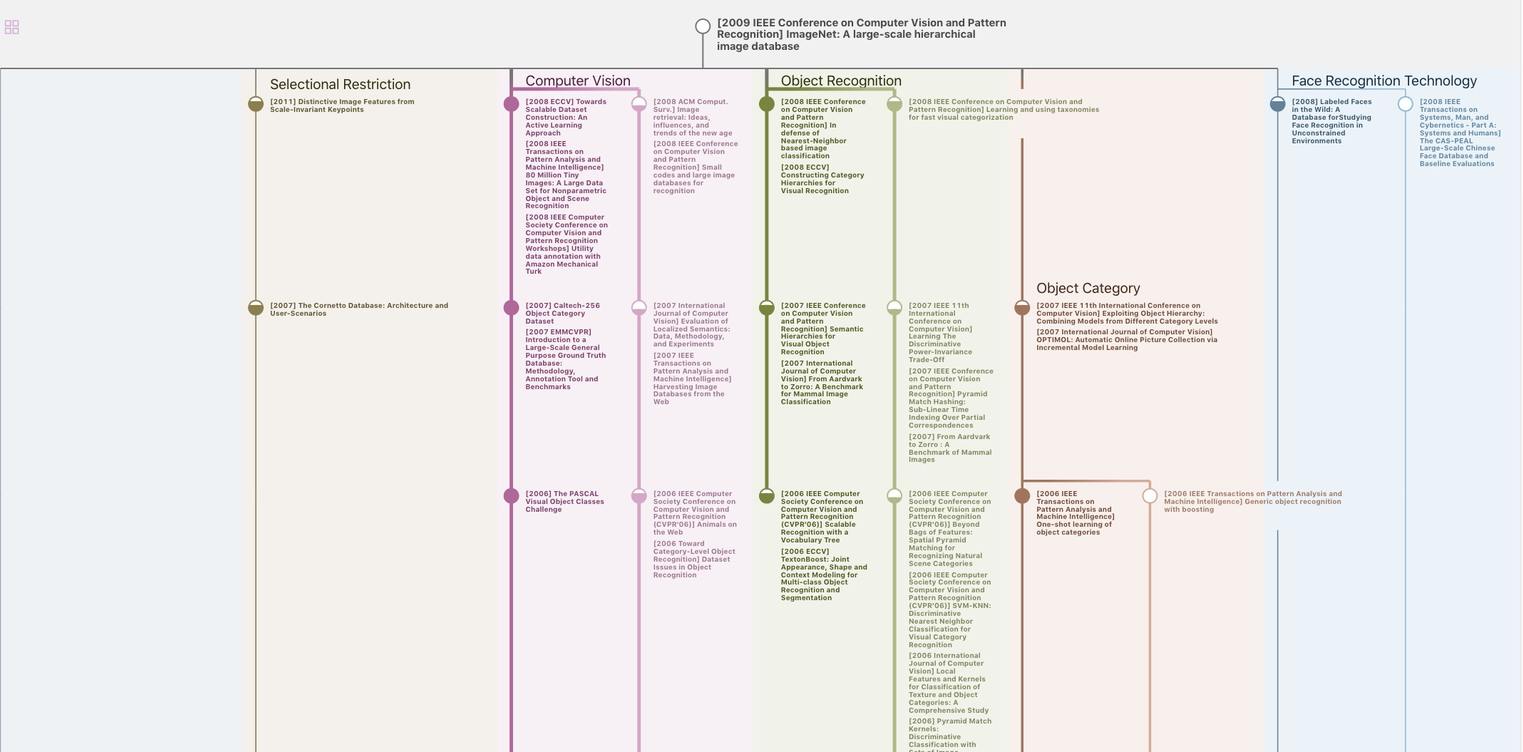
生成溯源树,研究论文发展脉络
Chat Paper
正在生成论文摘要