Personalized Audiobook Recommendations at Spotify Through Graph Neural Networks
arxiv(2024)
摘要
In the ever-evolving digital audio landscape, Spotify, well-known for its
music and talk content, has recently introduced audiobooks to its vast user
base. While promising, this move presents significant challenges for
personalized recommendations. Unlike music and podcasts, audiobooks, initially
available for a fee, cannot be easily skimmed before purchase, posing higher
stakes for the relevance of recommendations. Furthermore, introducing a new
content type into an existing platform confronts extreme data sparsity, as most
users are unfamiliar with this new content type. Lastly, recommending content
to millions of users requires the model to react fast and be scalable. To
address these challenges, we leverage podcast and music user preferences and
introduce 2T-HGNN, a scalable recommendation system comprising Heterogeneous
Graph Neural Networks (HGNNs) and a Two Tower (2T) model. This novel approach
uncovers nuanced item relationships while ensuring low latency and complexity.
We decouple users from the HGNN graph and propose an innovative multi-link
neighbor sampler. These choices, together with the 2T component, significantly
reduce the complexity of the HGNN model. Empirical evaluations involving
millions of users show significant improvement in the quality of personalized
recommendations, resulting in a +46
a +23
beyond audiobooks, benefiting established products like podcasts.
更多查看译文
AI 理解论文
溯源树
样例
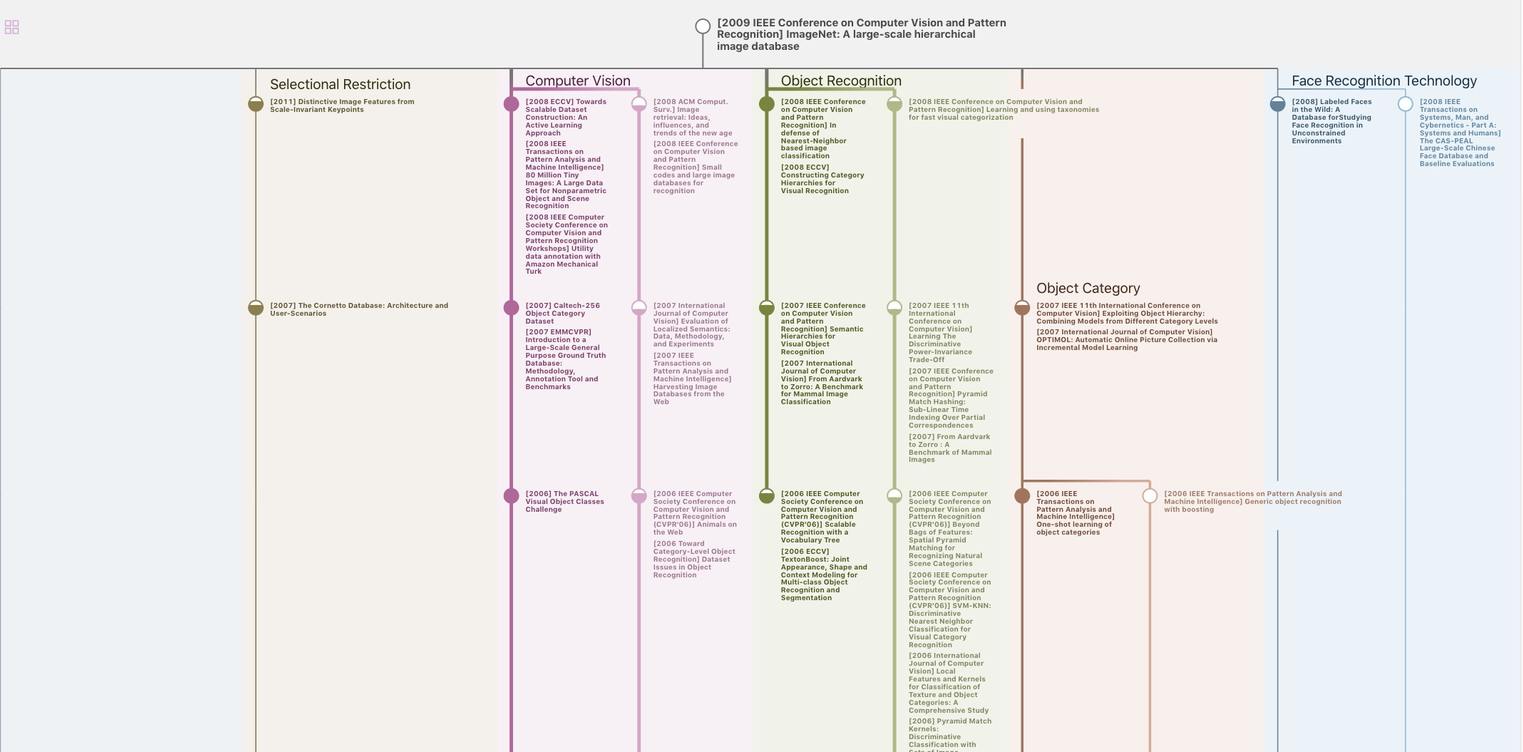
生成溯源树,研究论文发展脉络
Chat Paper
正在生成论文摘要