Cross-view hypergraph contrastive learning for attribute-aware recommendation
Information Processing & Management(2024)
摘要
Recommender systems typically model user–item interaction data to learn user interests and preferences. However, user interactions are often sparse and noisy. Moreover, existing works have constraints in modeling high-order interactions flexibly and sufficiently. We propose a novel Cross-view Hypergraph Contrastive Learning for Attribute-aware Recommendation (CHCLA) to deal with such issues. For considering the high-order interactions, CHCLA models user–item interaction through a graph convolutional network and user/item attribute information through hypergraph convolutional networks, learning user and item representations in two distinct ways. Furthermore, for the problem of sparse and noisy supervised signals, CHCLA uses self-supervised cross-view contrastive learning by generating self-augmented contrastive views between user–item interaction graph and user/item attribute interaction hypergraph to improve the robustness of graph representations learning. CHCLA not only captures user behavior representations but also accommodates inherent user and item attribute preferences. Extensive experiments illustrate that CHCLA performs better than advanced approaches, the NDCG@10 of CHCLA on MovieLens, Book-crossing and Taobao datasets is improved by 1.29%, 2.11% and 8.09% respectively, offering a promising avenue for further research and advancement in recommender systems.
更多查看译文
关键词
Recommender systems,Attribute interactions,Graph neural networks,Hypergraph neural networks,Contrastive learning
AI 理解论文
溯源树
样例
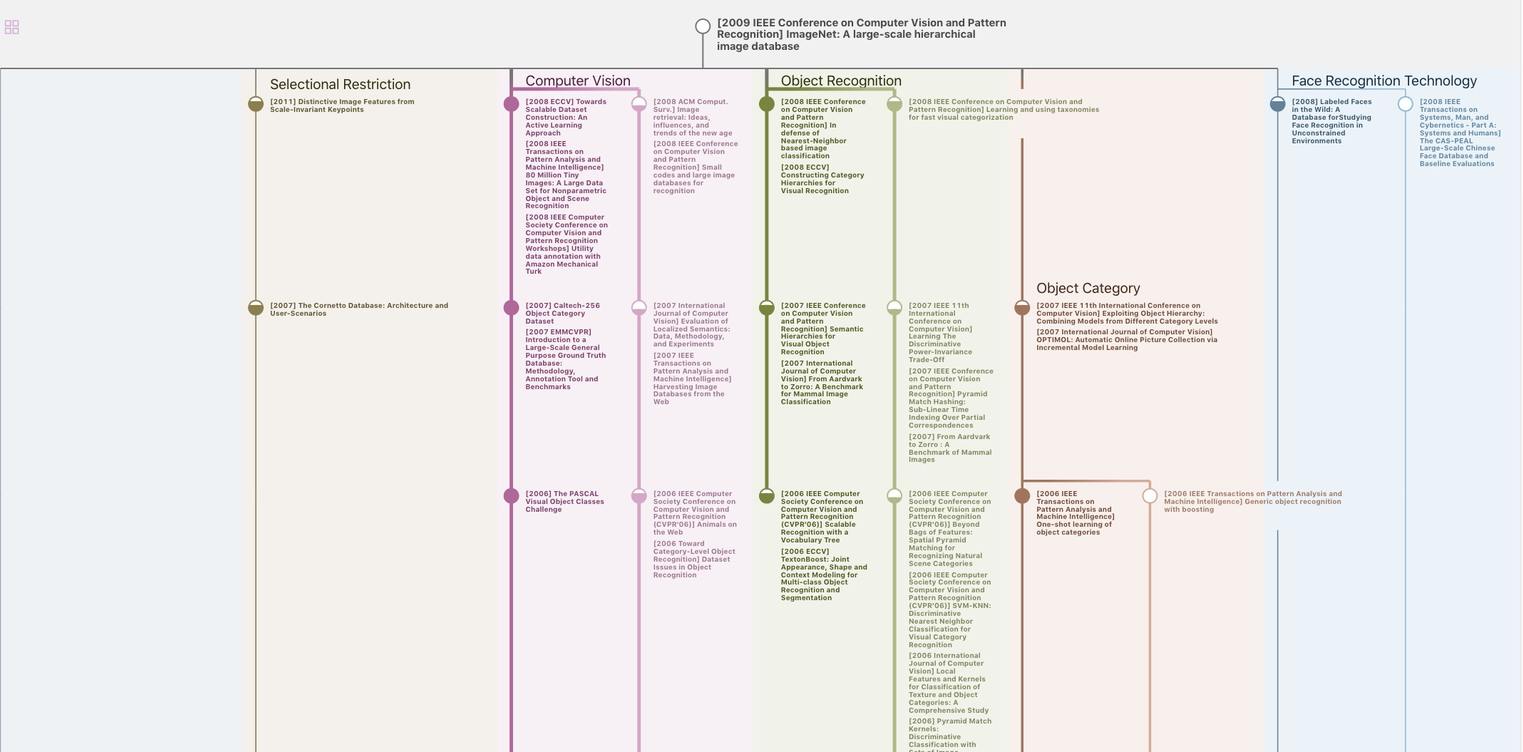
生成溯源树,研究论文发展脉络
Chat Paper
正在生成论文摘要