A subspace aggregating algorithm for accurate classification
Computational Statistics(2024)
摘要
We present a technique for learning via aggregation in supervised classification. The new method improves classification performance, regardless of which classifier is at its core. This approach exploits the information hidden in subspaces by combinations of aggregating variables and is applicable to high-dimensional data sets. We provide algorithms that randomly divide the variables into smaller subsets and permute them before applying a classification method to each subset. We combine the resulting classes to predict the class membership. Theoretical and simulation analyses consistently demonstrate the high accuracy of our classification methods. In comparison to aggregating observations through sampling, our approach proves to be significantly more effective. Through extensive simulations, we evaluate the accuracy of various classification methods. To further illustrate the effectiveness of our techniques, we apply them to five real-world data sets.
更多查看译文
关键词
Boosting,Bagging,Random forest,Supervised learning
AI 理解论文
溯源树
样例
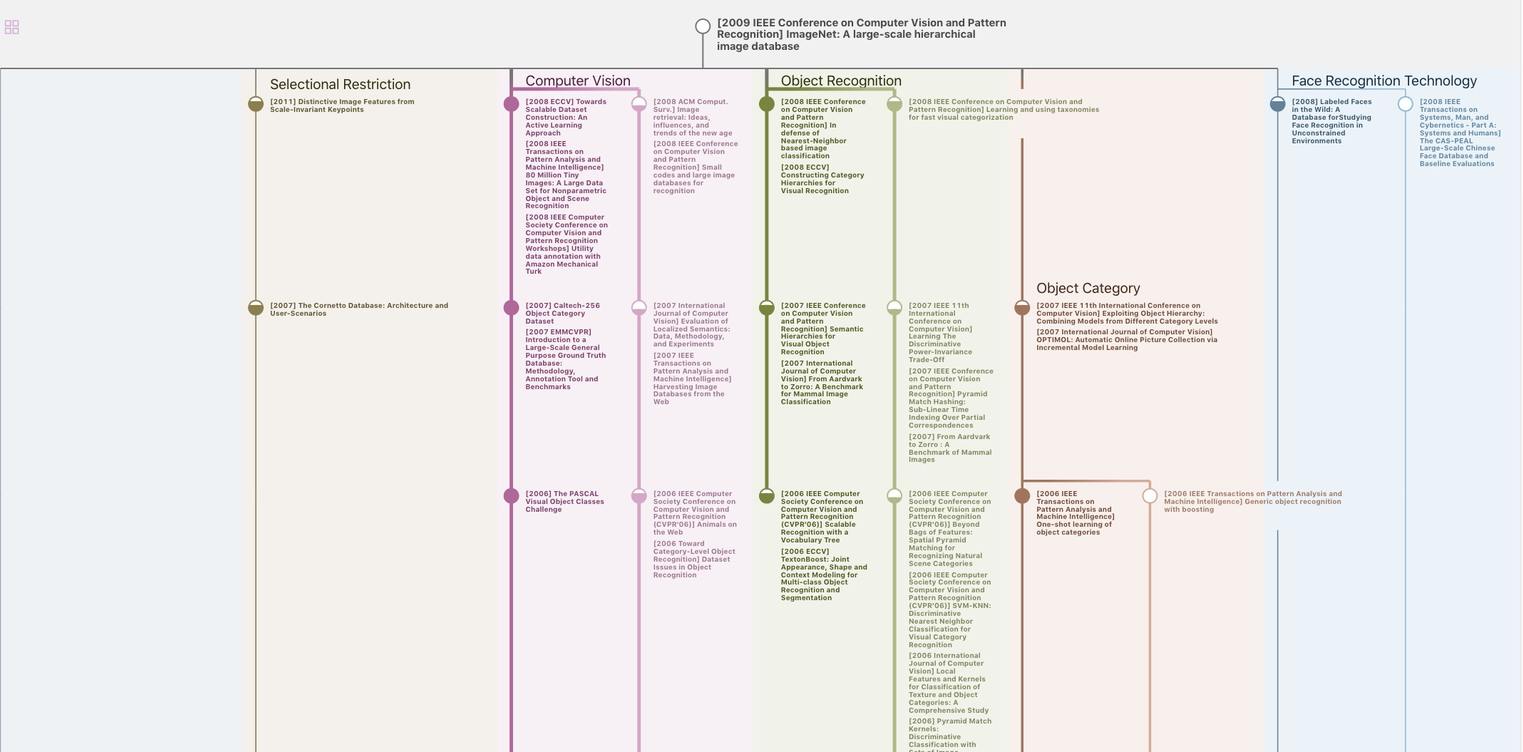
生成溯源树,研究论文发展脉络
Chat Paper
正在生成论文摘要