Prediction of airborne allergenic pollen concentrations with machine learning  
crossref(2024)
摘要
Over the past 30 years, the prevalence of allergies has increased continuously. Allergic rhinitis and asthma are among the most frequent non-communicable diseases and cause serious public health concerns worldwide, with the highest prevalence rates among children and adolescents. Moreover, experts assume that climate change will worsen the impact of allergies within the next decades. Therefore, it is essential to develop high quality methods and tools that can forecast allergenic pollen in the air to prevent sensitized against contact to high concentrations of aeroallergens. In this study we aim to develop a tool for prediction of pollen concentrations based on machine learning (ML) methods with the use of measured pollen concentrations and modelled meteorological parameters. We focus on the birch pollen, which is the most allergenic tree taxon in Central Europe. We use daily pollen concentration from Wrocław aerobiological station (south-west Poland) for years 2006 – 2022. Pollen grains were gathered with the use of the Burkard trap and counted following the recommendations of the International Association for Aerobiology. Meteorological data for the analysed period were provided with the Weather Research and Forecasting (WRF) model. We test different machine learning algorithms including: Random Forest, xgBoost, Support Vector Regression (SVR) and Multilayer Perceptron (MLP). The algorithms are used to detect the days with pollen concentrations exceeding the threshold levels of 20, 75 and 90 pollen m-3, which correspond to the first symptoms, symptoms in all subjects and severe symptoms, respectively. For each ML algorithm, the whole data set was split into training and testing subsets in a proportion where the training set was ¾ of the data and the rest was the independent test set for the final model verification. Each model was checked during cross-validation for optimal hyperparameters. We test different parameters, including temporal variables and lagged predictors (e.g. pollen concentrations, air temperature, relative humidity, wind speed, solar radiation, planetary boundary height, rainfall – all derived with the mesoscale meteorological WRF model) to choose the most significant for prediction of pollen concentrations. We also compare the performance of the different algorithms in terms of such error metrics as F1 score, ROC-AUC and PR-AUC. The results of the analysis will be applied to forecast pollen concentrations based on the automatic pollen detector, newly installed at the station, and weather forecasts.
更多查看译文
AI 理解论文
溯源树
样例
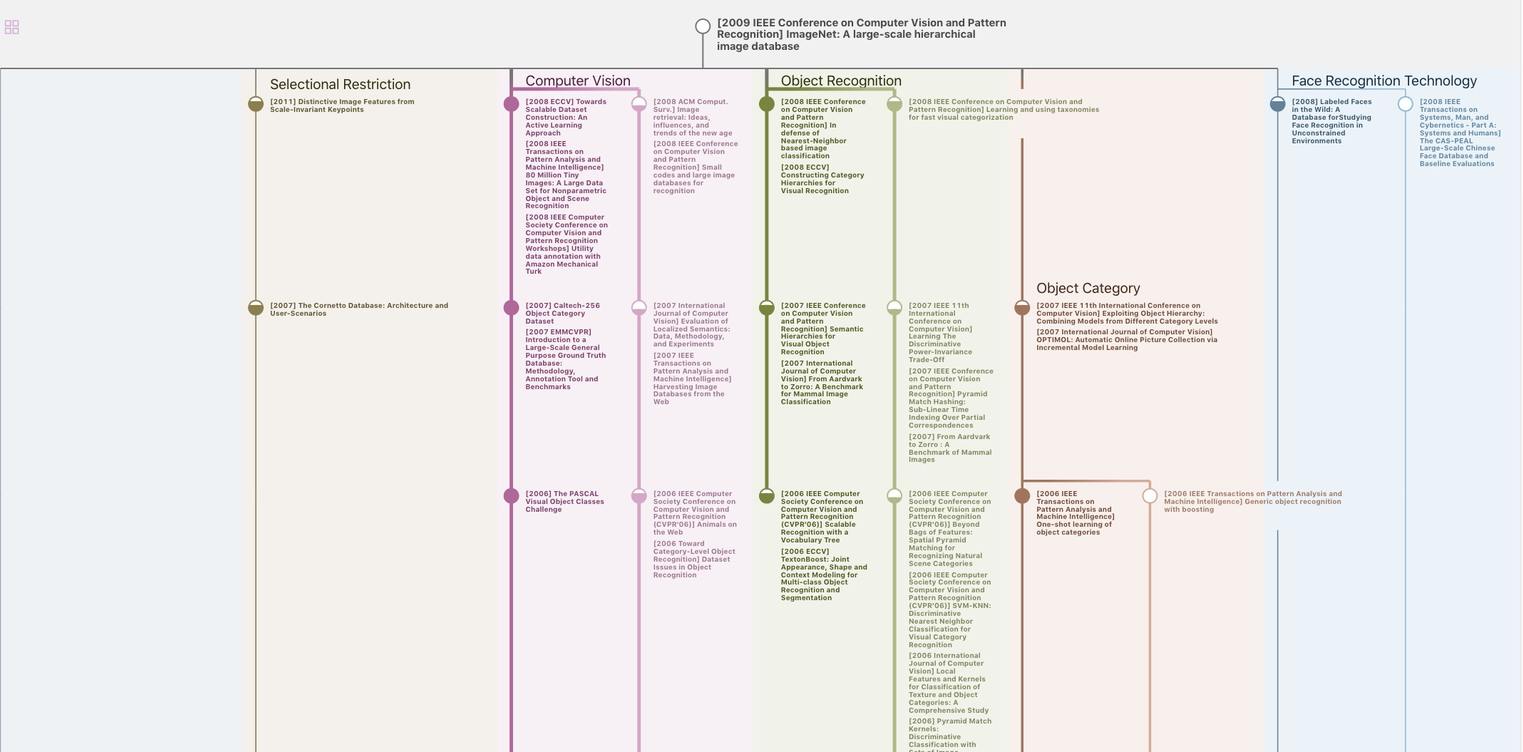
生成溯源树,研究论文发展脉络
Chat Paper
正在生成论文摘要