SliNet: Slicing-Aided Learning for Small Object Detection
IEEE SIGNAL PROCESSING LETTERS(2024)
摘要
In recent years, small object detection has been widely applied to aerial scenes. Although existing small object detection algorithms have achieved significant success, it remains challenging to ensure an acceptable processing speed and detection accuracy simultaneously in high-resolution images. In this work, we propose a novel framework called SliNet, a slicing-aided learning network with a SPPFCSPC Block and a Content-Aware ReAssembly of Features (CARAFE) Block merged. Since aerial images usually have high resolutions and small detection targets, we slice images into smaller overlapping patches and integrate SPPFCSPC and CARAFE to find attention region with larger receptive field in dense-object scenes. Experimental results on dataset VisDrone-DET2019 show that the SliNet achieves competitive performance and obtains faster detection speed due to the decrease of computational cost. On the VisDrone2019-DET-val dataset, we attain a mAP score of 46.4% with a mAP50 score of 67.1%, which is better than the state-of-the-art models, demonstrating the superiority of our approach.
更多查看译文
关键词
Object detection,small object detection,sliced inference,VisDrone
AI 理解论文
溯源树
样例
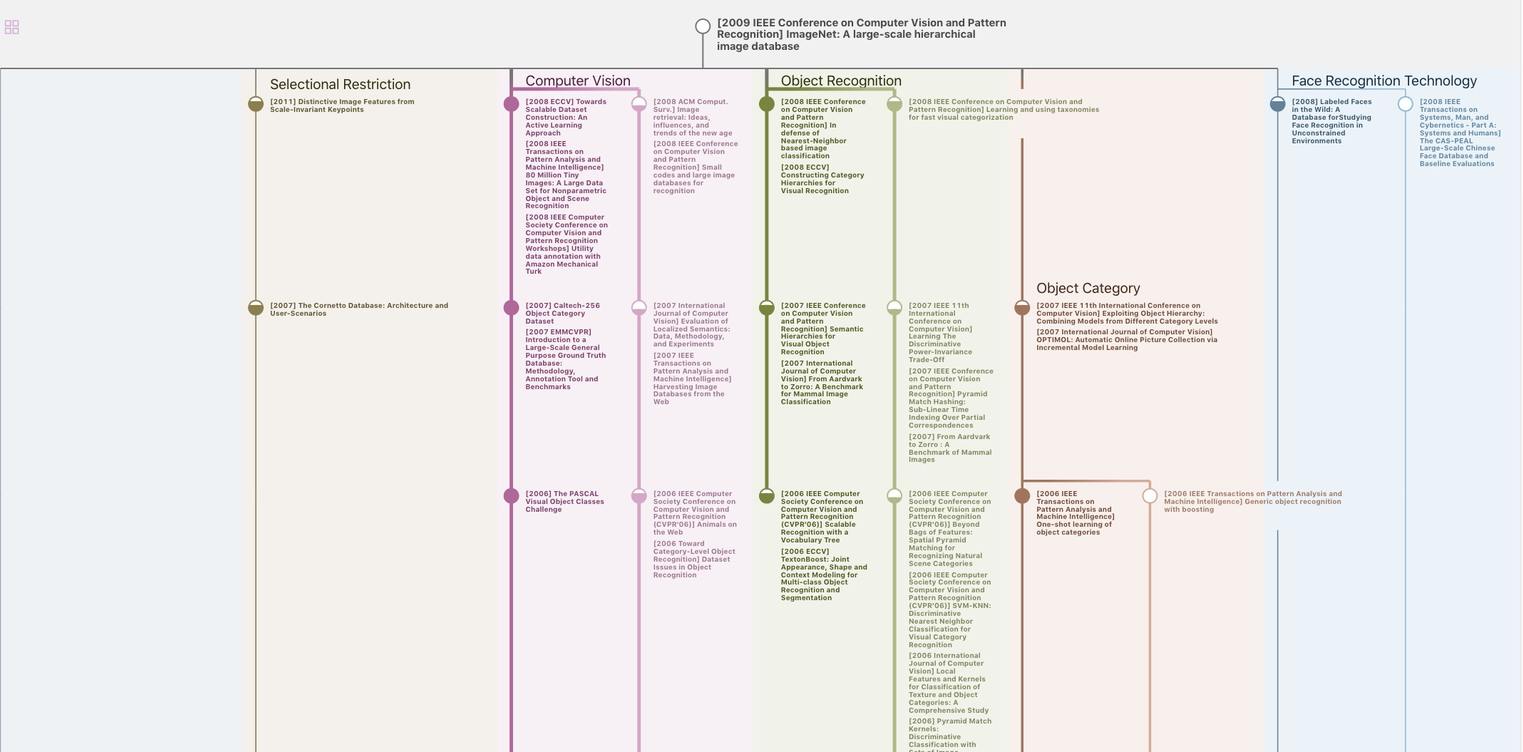
生成溯源树,研究论文发展脉络
Chat Paper
正在生成论文摘要