Enhancing Space Mission Return through On-Board Data Reduction using Unsupervised Machine Learning
crossref(2024)
摘要
The efficient use of the provided downlink capacity for scientific data is a fundamental aspect of space exploration. The use thereof can be optimised through sophisticated data reduction techniques and automation of processes on board that otherwise require interaction with the operations centres on Earth. Machine learning-based autonomous methods serve both purposes; yet space-based ML applications remain relatively rare compared to the application of ML on Earth to data acquired in space. In this contribution, we present a potential application of unsupervised machine learning to cluster mass spectrometric data on-board a spacecraft. Data were acquired from a phoscorite rock [1] using a prototype of a laser ablation ionisation mass spectrometer (LIMS) for space research [2]. Two unsupervised dimensionality reduction algorithms, UMAP and densMAP [3,4], were employed to construct low-dimensional representations of the data. Clusters corresponding to different mineral phases within these embeddings were found using HDBSCAN [5]. The impact of data pre-processing and model parameter selection on the classification outcome was investigated through varying levels of pre-processing and extensive grid searches. Both UMAP and densMAP effectively isolated major mineral phases present within the rock sample, but densMAP additionally found minor inclusions present only in a small number of mass spectra. However, densMAP exhibited higher sensitivity to data pre-processing, yielding lower scores for minimally treated data compared to UMAP. For highly processed data, both UMAP and densMAP exhibited high stability across a broad model parameter space. Given that the data were recorded using a miniature mass spectrometric instrument designed for space flight, these methods demonstrate effective strategies for substantial reduction of data similarly to what is anticipated on future space missions. Autonomous clustering of data into groups of different chemical composition, followed by the downlink of a representative mass spectrum of each cluster, aids in identifying relevant data. Mission return can therefore be enhanced through the selective downlink of data of interest. As both UMAP and densMAP, coupled with HDBSCAN, are relatively complex algorithms compared to more traditional techniques, such as k-means, it is important to evaluate the benefits and drawbacks of using simpler methods on-board spacecraft. [1] Tulej, M. et al., 2022, https://doi.org/10.3390/universe8080410. [2] Riedo, A. et al., 2012, https://doi.org/10.1002/jms.3104. [3] McInnes, L. et al., 2018, https://doi.org/10.48550/arXiv.1802.03426. [4] Narayan, A., et al., 2021, https://doi.org/10.1038/s41587-020-00801-7. [5] McInnes, L., et al., 2017, https://doi.org/10.21105/JOSS.00205.
更多查看译文
AI 理解论文
溯源树
样例
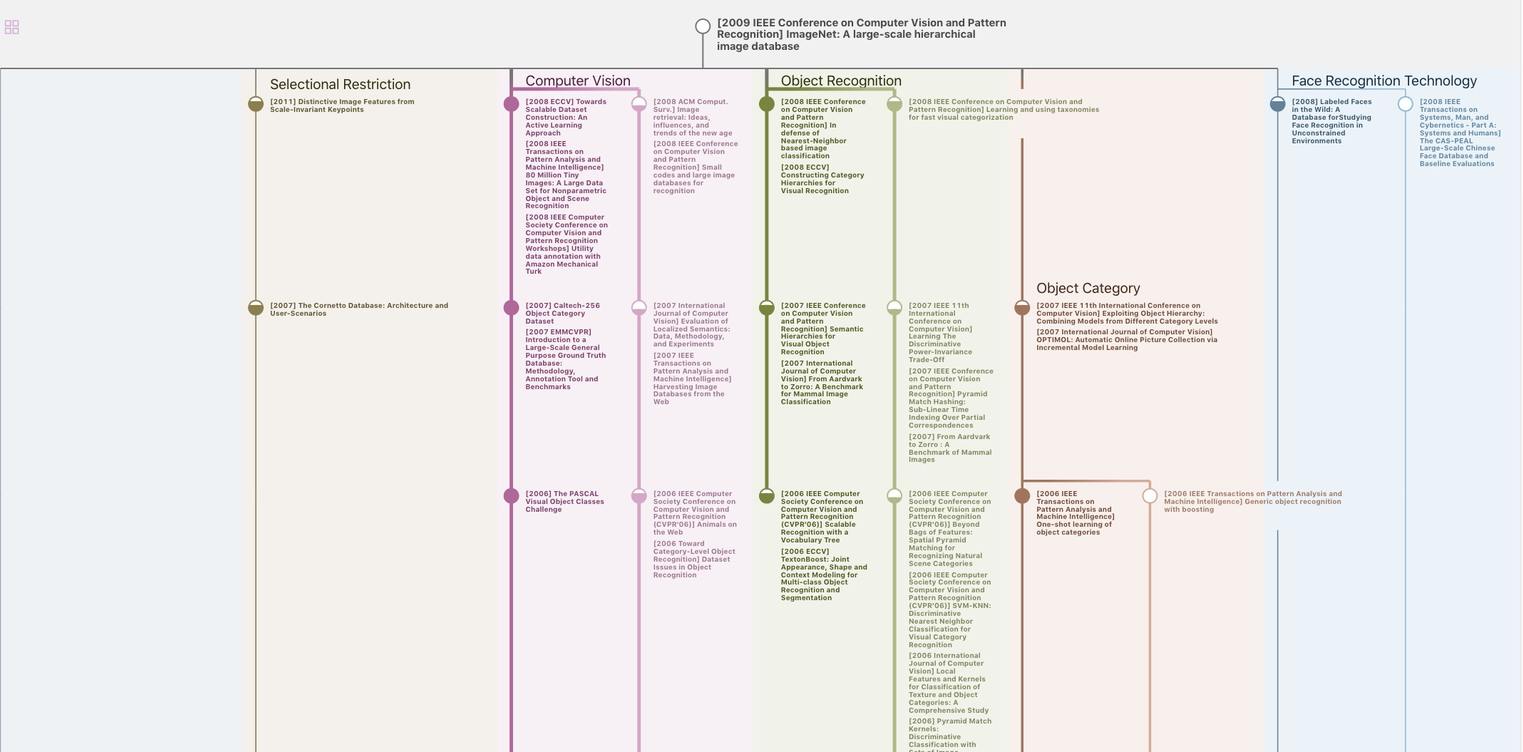
生成溯源树,研究论文发展脉络
Chat Paper
正在生成论文摘要