Using Supervised Machine Learning Algorithms for Ground Motion Prediction: A Comparison with the Traditional functional form Approach in Central Italy
crossref(2024)
摘要
The ground motion intensity measures are often obtained using Ground-Motion-Prediction-Equations (GMPEs) or more in general referred to Ground Motion Models (GMMs), which are empirical mathematical equations that relate the ground motion toseismological parameters (e.g., magnitude, source-to-site distance,focal depth and the average shear-wave velocity in the uppermost 30 m, Vs30). GMPEs are worldwide used as a tool for seismic hazard assessment and seismic design, usually derived from past earthquakes records through linear regression and predefined functional forms. In the last 20 years, the application of artificial intelligence in earth sciences has been significatively applied to solve nonlinear problems that cannot be explained by empirical approaches. The last seismic events in central Italy, including the earthquakes in L'Aquila (2009) and the Amatrice-Visso-Norcia sequence (2016-2018), have provided a substantial dataset comprising approximately 34,000 waveforms, contributing to the creation of a robust and accurate database(central Italy dataset). In this work, we leverage the valuable data compiled by their work for the calibration of prediction models based on supervised Machine Learning (ML) to predict and evaluate the ground motion intensity measures and comparison the result with an existing GMPE currently used in Italy (ITA18). Several ML regression algorithms are systematically examined and validated, the XGBoost algorithm is identified as the optimal choice, offering a balanced performance in terms of error minimization, interpretability, and computational efficiency. The evaluation encompasses the estimation of diverse Intensity Measures (IMs), such as Peak Ground Acceleration (PGA), Peak Ground Velocity (PGV), and Acceleration Response Spectra at 5% damping (SA) across different time periods (e.g., 0.1 second, 1.0 second, and 2.0 seconds). The ML model developed in this research demonstrates high accuracy, exhibiting notable improvements compared to the Italian Ground Motion Prediction Equation (GMPE). These advancements suggest the model's efficacy in enhancing seismic hazard assessment. Moreover, the versatility of this model extends beyond the study area, as it can be applied to various worldwide geological contexts, provided seismic data is available. The outcomes of this work not only contribute to refining local seismic risk evaluations but also offer valuable insights for seismic studies in diverse global regions.
更多查看译文
AI 理解论文
溯源树
样例
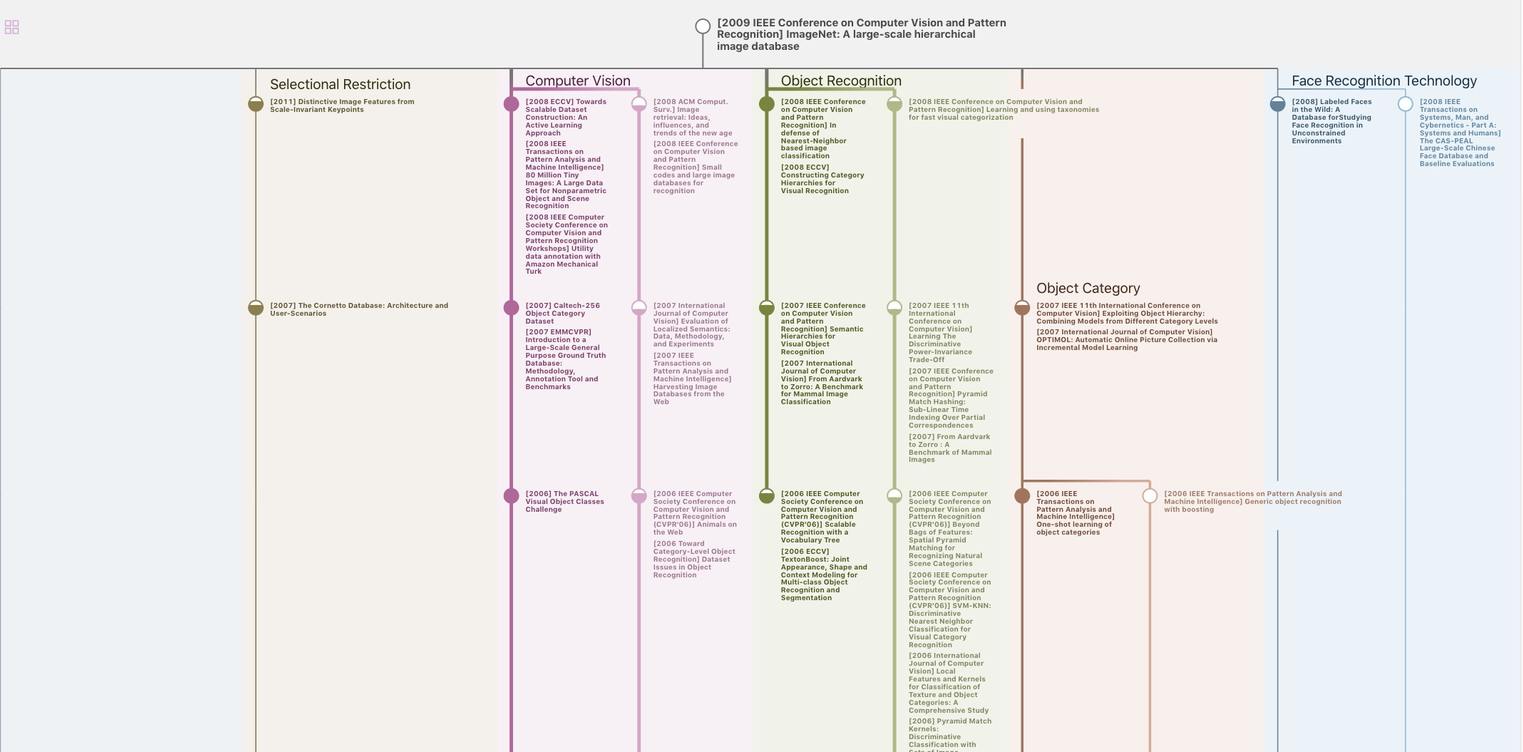
生成溯源树,研究论文发展脉络
Chat Paper
正在生成论文摘要