Decentralized Stochastic Optimization With Pairwise Constraints and Variance Reduction
IEEE TRANSACTIONS ON SIGNAL PROCESSING(2024)
摘要
This paper focuses on minimizing the decentralized finite-sum optimization over a network, where each pair of neighboring agents is associated with a nonlinear proximity constraint. Additionally, each agent possesses a private convex cost that can be decomposed into an average of multiple constituent functions. The goal of the network is to collectively minimize the sum of individual costs while satisfying all constraints. Due to their fast convergence and low computational burden, stochastic variance reduction methods have primarily been studied for finite-sum minimization problems. However, these algorithms did not consider the constrained optimization problems. To bridge this gap, we propose a decentralized stochastic algorithmic framework called VQ-VR. This framework extends the virtual-queue-based algorithm introduced in [1] to stochastic settings for constrained optimization instead of using the classical saddle point method. VQ-VR operates between stochastic variance-reduced gradient descent steps and virtual queue updates. Furthermore, we describe and analyze two specific instantiations of this framework, namely VQ-SVRG and VQ-SAGA. Our convex analysis relies on the drift of a novel quadratic Lyapunov function. We prove that VQ-SVRG and VQ-SAGA both achieve the sublinear convergence rate of O(1/K) in terms of expected cost suboptimality and constraint violations for smooth and general convex problems, where K is the number of iterations. To the best of our knowledge, VQ-VR is the first stochastic algorithm capable of solving decentralized nonlinear constrained optimization problems with a convergence rate of O(1/K). Additionally, we present numerical results on two specific applications: decentralized QCQP and decentralized logistic regression. These results verify the theoretical results and demonstrate that, on a per-gradient-evaluation basis, our algorithms achieve a relative cost gap improvement of more than 7dB compared to existing methods.
更多查看译文
关键词
Stochastic optimization,distributed optimization,constrained optimization,variance reduction
AI 理解论文
溯源树
样例
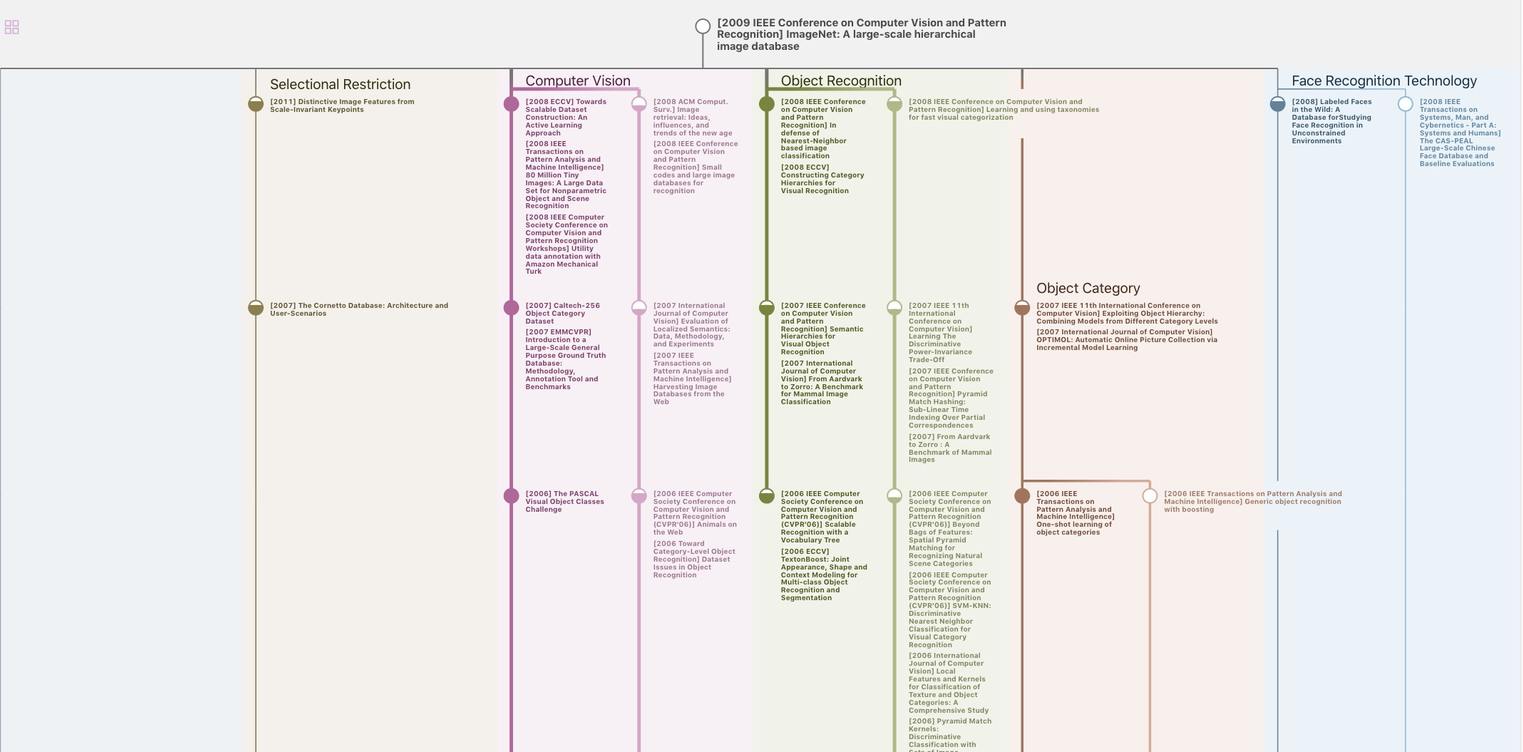
生成溯源树,研究论文发展脉络
Chat Paper
正在生成论文摘要