Battery Cross-Operation-Condition Lifetime Prediction via Interpretable Feature Engineering Assisted Adaptive Machine Learning
ACS ENERGY LETTERS(2023)
摘要
We develop an adaptive machine-learning framework thataddressescross-operation-condition battery lifetime prediction, particularlyunder extreme conditions. This framework uses correlation alignmentto correct feature divergence under fast-charging and extremely fast-chargingconditions. We report a linear correlation between feature adaptabilityand prediction accuracy. Higher adaptability generally leads to betterprediction accuracy, aiding efficient feature engineering. Our analysisshows that the first 120 cycles provide sufficient information forlifetime prediction, and extending data to the first 320 cycles onlymarginally improves prediction accuracy. An early prediction usingonly one feature at the 20th cycle produces a 93.3% accuracy, savingup to 99.4% computation time and repetitive tests. Our quantitativeadaptability evaluation enhances prediction accuracy while reducinginformation redundancy via proper feature and cycle selections. Theproposed framework is validated under another unseen complex operationcondition with a 90.3% accuracy without prior knowledge.
更多查看译文
关键词
cross-operation-condition
AI 理解论文
溯源树
样例
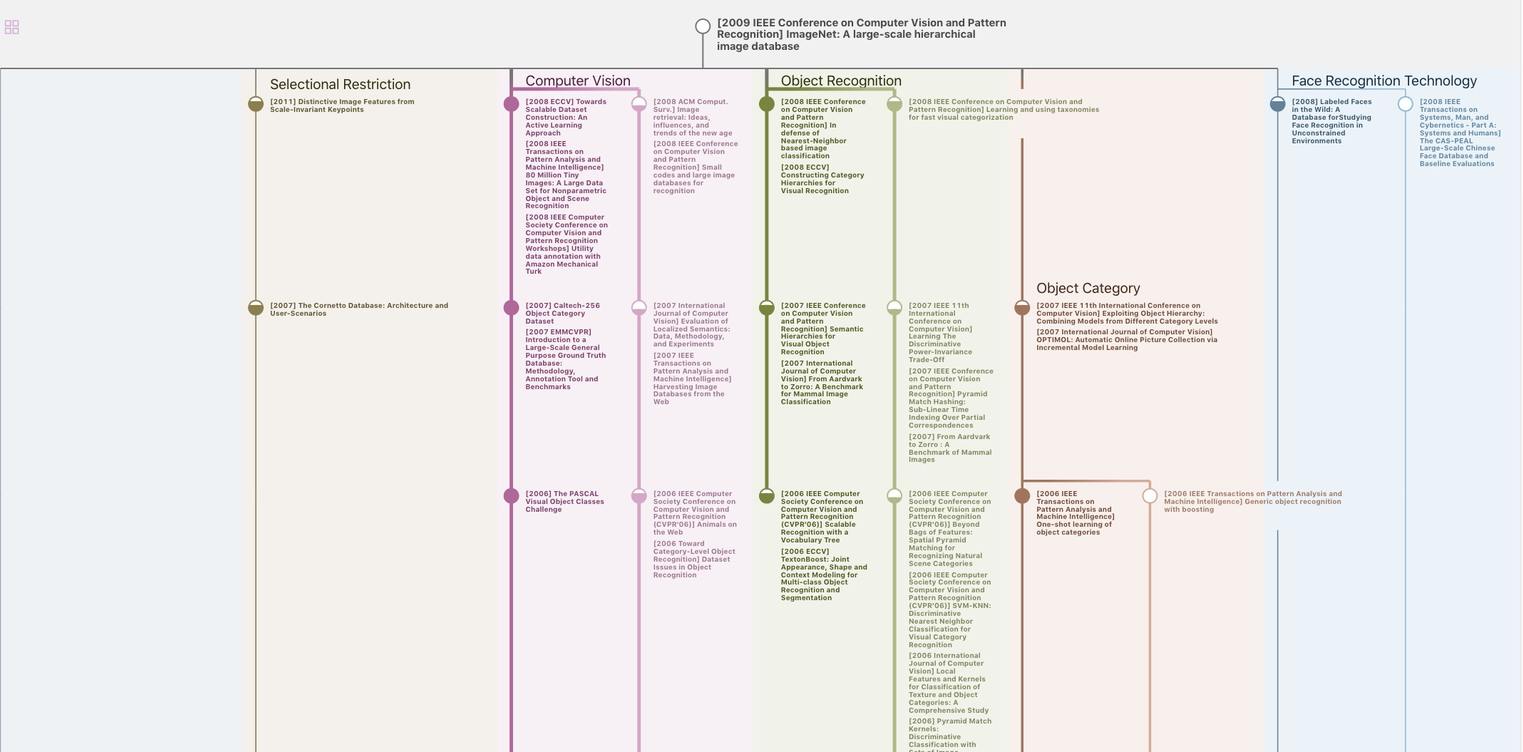
生成溯源树,研究论文发展脉络
Chat Paper
正在生成论文摘要